Facial expression recognition via learning deep sparse autoencoders.
Neurocomputing(2018)
摘要
Facial expression recognition is an important research issue in the pattern recognition field. In this paper, we intend to present a novel framework for facial expression recognition to automatically distinguish the expressions with high accuracy. Especially, a high-dimensional feature composed by the combination of the facial geometric and appearance features is introduced to the facial expression recognition due to its containing the accurate and comprehensive information of emotions. Furthermore, the deep sparse autoencoders (DSAE) are established to recognize the facial expressions with high accuracy by learning robust and discriminative features from the data. The experiment results indicate that the presented framework can achieve a high recognition accuracy of 95.79% on the extended Cohn–Kanade (CK+) database for seven facial expressions, which outperforms the other three state-of-the-art methods by as much as 3.17%, 4.09% and 7.41%, respectively. In particular, the presented approach is also applied to recognize eight facial expressions (including the neutral) and it provides a satisfactory recognition accuracy, which successfully demonstrates the feasibility and effectiveness of the approach in this paper.
更多查看译文
关键词
Facial expression recognition,Sparse autoencoders,Deep architecture,High-dimensional feature,Histogram of oriented gradients (HOG)
AI 理解论文
溯源树
样例
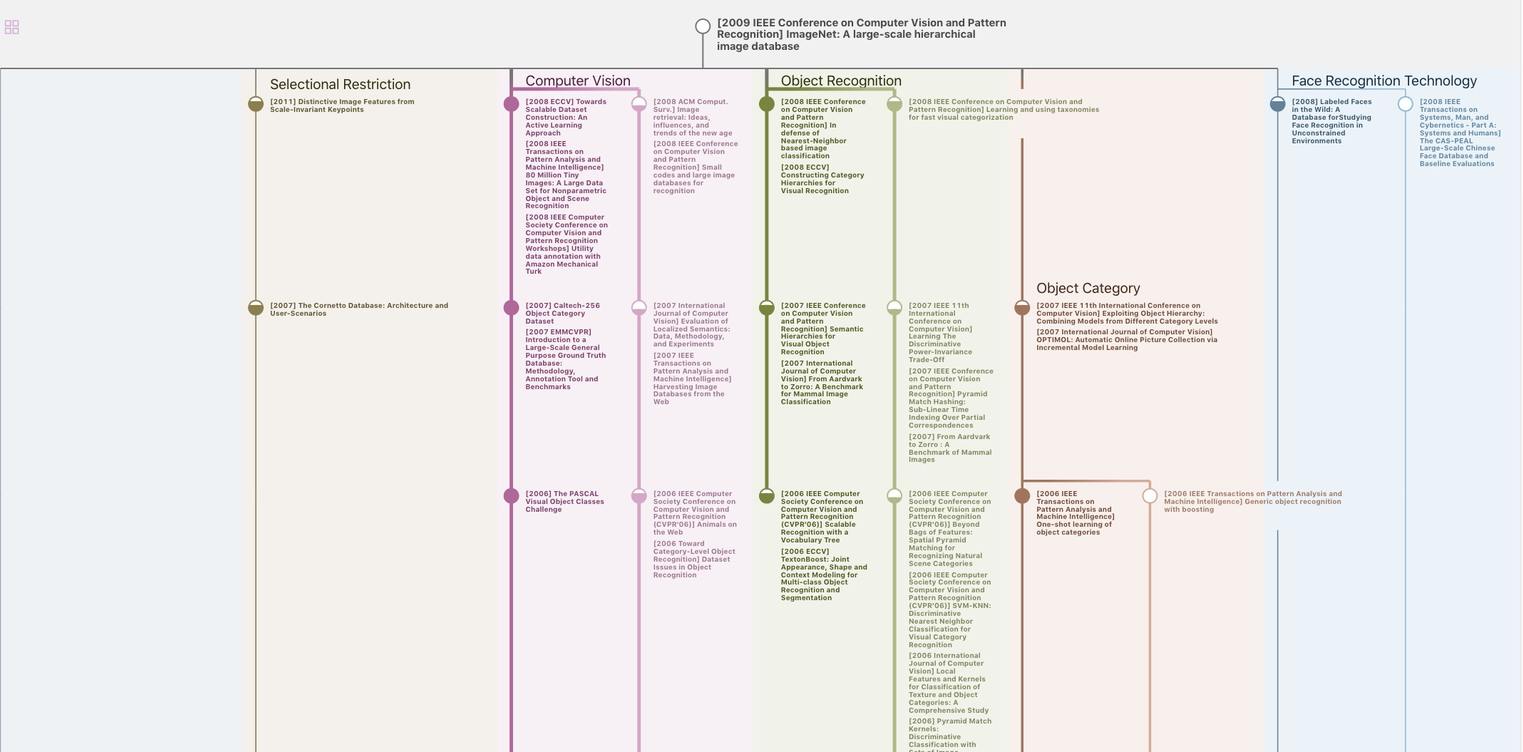
生成溯源树,研究论文发展脉络
Chat Paper
正在生成论文摘要