Embedding Learning on Spectral–Spatial Graph for Semisupervised Hyperspectral Image Classification
IEEE geoscience and remote sensing letters(2017)
摘要
Scarcity of labeled samples is the main obstacle for hyperspectral image classification tasks when labeling data is considerably costly and time-consuming in real-world scenarios. To alleviate any underfitting problem that may occur due to lack of training data, semisupervised classification frameworks explore the intrinsic information of unlabeled samples and bridge labeled and unlabeled data. In this letter, we propose a novel framework that learns underlying manifold representation and semisupervised classifier simultaneously. It avoids explicit eigenvector decomposition and directly samples via iterating random walk on the similarity graph, which makes it feasible to implement on huge graphs. To verify the efficacy of embedding the learning process, we compare the proposed method with other dimensionality reduction and manifold-learning-based approaches. Experimental results show that compared to the methods using traditional semisupervised strategies, the graph embedding method gives a better result.
更多查看译文
关键词
Hyperspectral imagery (HSI),manifold learning,neural networks,representation learning,semisupervised classification
AI 理解论文
溯源树
样例
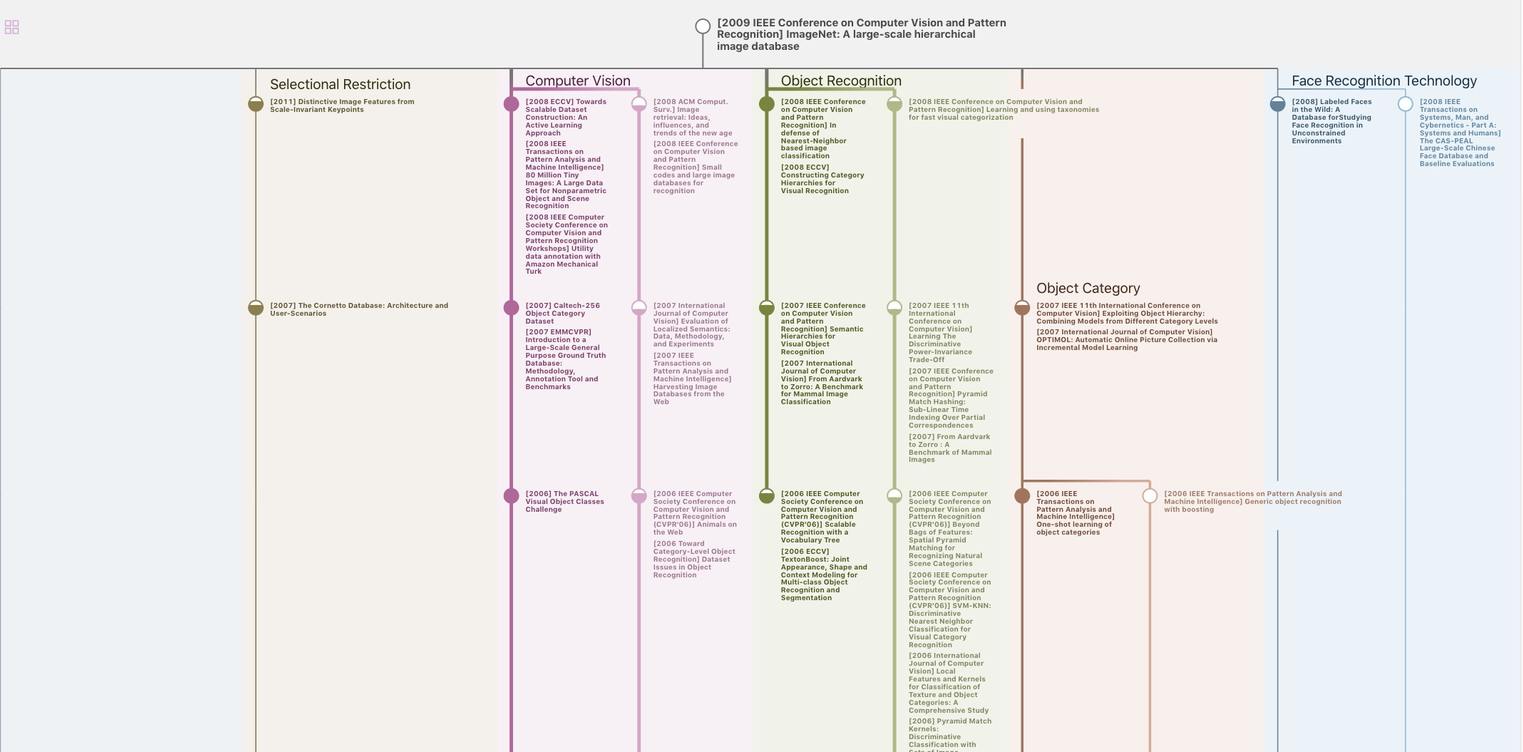
生成溯源树,研究论文发展脉络
Chat Paper
正在生成论文摘要