A kernel based true online Sarsa(λ) for continuous space control problems.
Comput. Sci. Inf. Syst.(2017)
摘要
Reinforcement learning is an efficient learning method for the control problem by interacting with the environment to get an optimal policy. However, it al so faces challenges such as low convergence accuracy and slow convergence. More over, conventional reinforcement learning algorithms could hardly solve continuous control problems. The kernel-based method can accelerate convergence speed and improve convergence accuracy; and the policy gradient method is a good way to deal with continuous space problems. We proposed a Sarsa(?) version of true online time difference algorithm, named True Online Sarsa(?)(TOSarsa(?)), on the basis of the clustering-based sample specification method and selective kernel-based value function. The TOSarsa(?) algorithm has a consistent result with both the forward view and the backward view which ensures to get an optimal policy in less time. Afterwards we also combined TOSarsa(?) with heuristic dynamic programming. The experiments showed our proposed algorithm worked well in dealing with continuous control problem.
更多查看译文
关键词
continuous space control problems,kernel
AI 理解论文
溯源树
样例
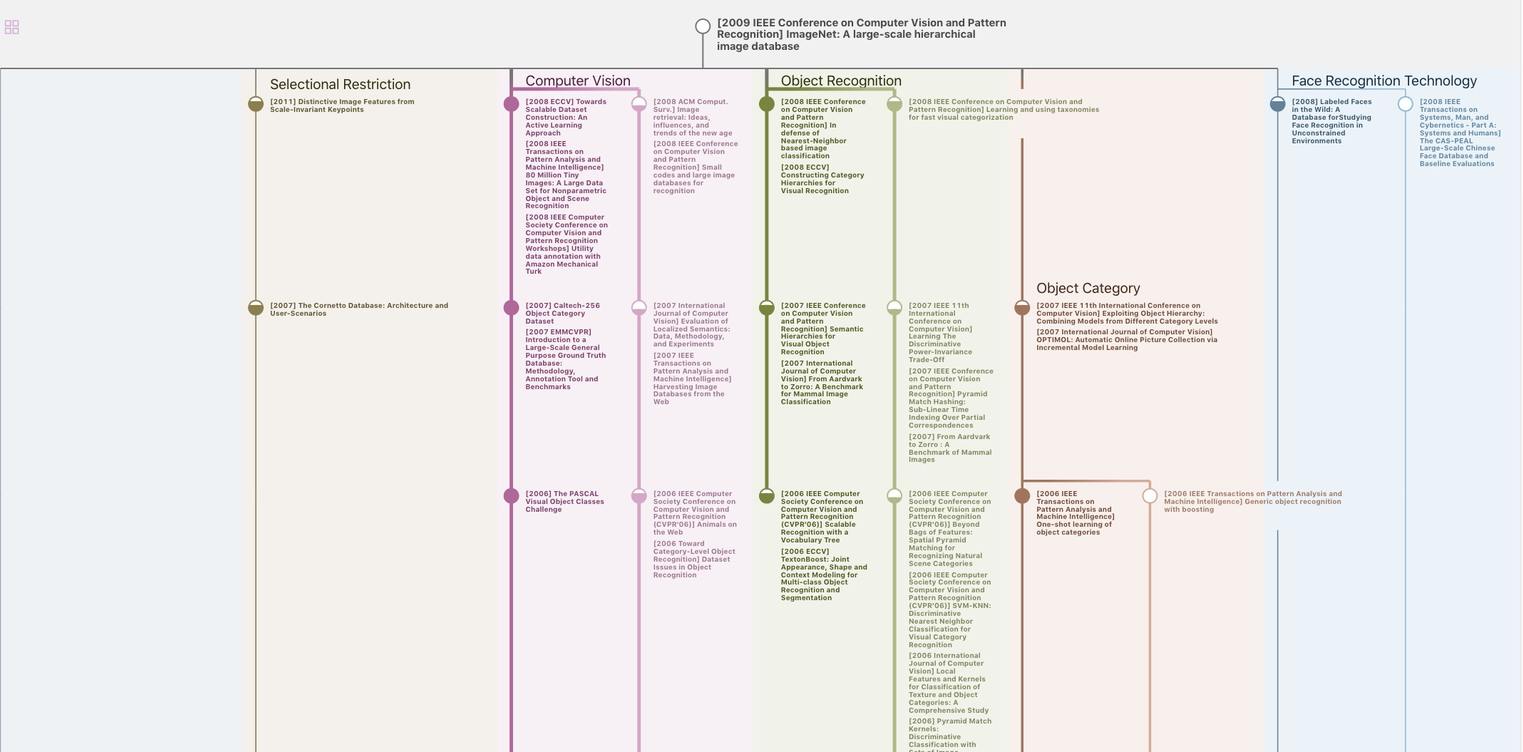
生成溯源树,研究论文发展脉络
Chat Paper
正在生成论文摘要