A Representer Theorem for Deep Kernel Learning
Journal of Machine Learning Research (JMLR)(2019)CCF ASCI 3区
Abstract
In this paper we provide a finite-sample and an infinite-sample representer theorem for the concatenation of (linear combinations of) kernel functions of reproducing kernel Hilbert spaces. These results serve as mathematical foundation for the analysis of machine learning algorithms based on compositions of functions. As a direct consequence in the finite-sample case, the corresponding in finite-dimensional minimization problems can be recast into (nonlinear) finite-dimensional minimization problems, which can be tackled with nonlinear optimization algorithms. Moreover, we show how concatenated machine learning problems can be reformulated as neural networks and how our representer theorem applies to a broad class of state-of-the-art deep learning methods.
MoreTranslated text
Key words
Deep Learning,Model Reduction,Nonlinear Systems,Inverse Problems
PDF
View via Publisher
AI Read Science
AI Summary
AI Summary is the key point extracted automatically understanding the full text of the paper, including the background, methods, results, conclusions, icons and other key content, so that you can get the outline of the paper at a glance.
Example
Background
Key content
Introduction
Methods
Results
Related work
Fund
Key content
- Pretraining has recently greatly promoted the development of natural language processing (NLP)
- We show that M6 outperforms the baselines in multimodal downstream tasks, and the large M6 with 10 parameters can reach a better performance
- We propose a method called M6 that is able to process information of multiple modalities and perform both single-modal and cross-modal understanding and generation
- The model is scaled to large model with 10 billion parameters with sophisticated deployment, and the 10 -parameter M6-large is the largest pretrained model in Chinese
- Experimental results show that our proposed M6 outperforms the baseline in a number of downstream tasks concerning both single modality and multiple modalities We will continue the pretraining of extremely large models by increasing data to explore the limit of its performance
Try using models to generate summary,it takes about 60s
Must-Reading Tree
Example
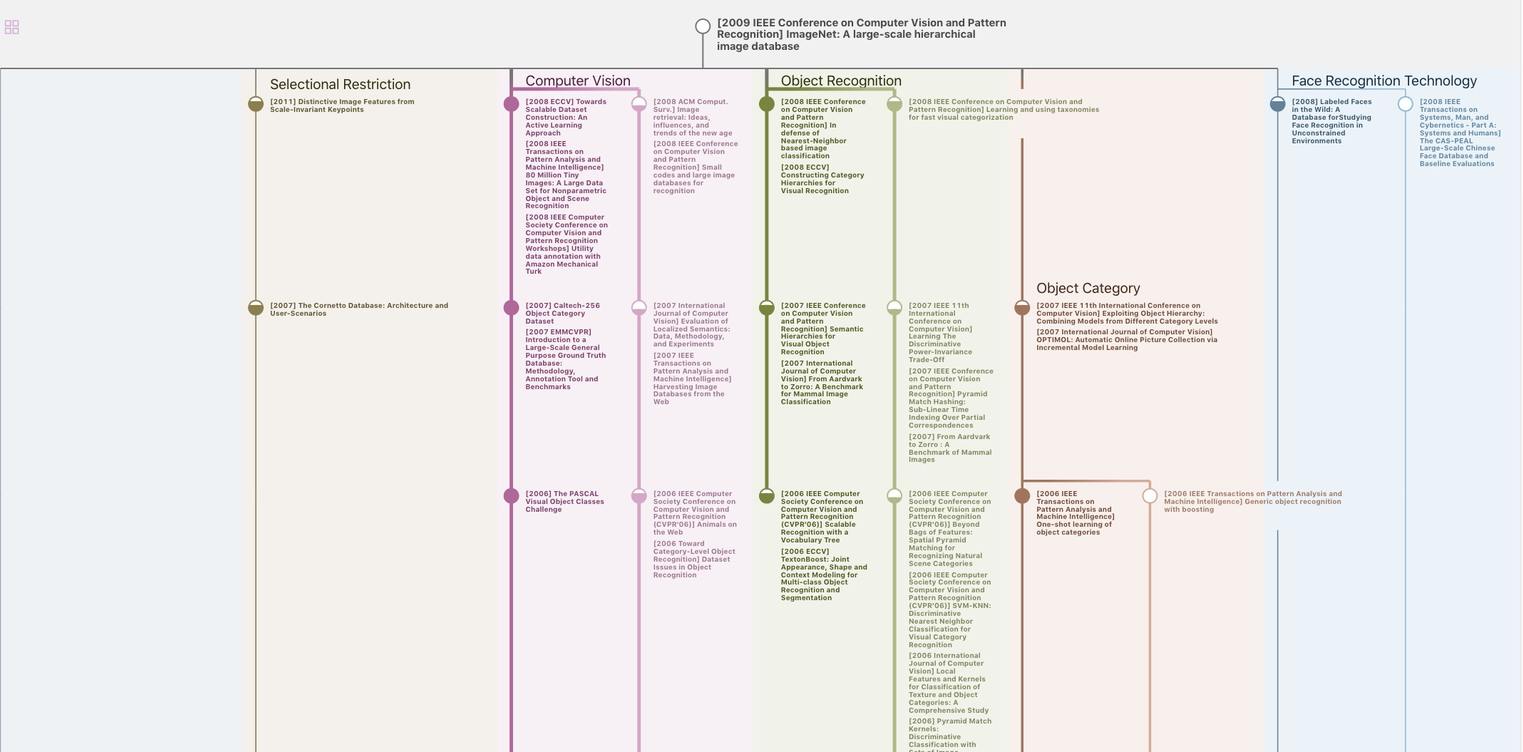
Generate MRT to find the research sequence of this paper
Related Papers
2011
被引用33 | 浏览
1970
被引用1187 | 浏览
2010
被引用12 | 浏览
2016
被引用28 | 浏览
2013
被引用34 | 浏览
Data Disclaimer
The page data are from open Internet sources, cooperative publishers and automatic analysis results through AI technology. We do not make any commitments and guarantees for the validity, accuracy, correctness, reliability, completeness and timeliness of the page data. If you have any questions, please contact us by email: report@aminer.cn
Chat Paper
GPU is busy, summary generation fails
Rerequest