Attention-Based Models for Text-Dependent Speaker Verification
2018 IEEE INTERNATIONAL CONFERENCE ON ACOUSTICS, SPEECH AND SIGNAL PROCESSING (ICASSP)(2018)
摘要
Attention-based models have recently shown great performance on a range of tasks, such as speech recognition, machine translation, and image captioning due to their ability to summarize relevant information that expands through the entire length of an input sequence. In this paper, we analyze the usage of attention mechanisms to the problem of sequence summarization in our end-to-end text-dependent speaker recognition system. We explore different topologies and their variants of the attention layer, and compare different pooling methods on the attention weights. Ultimately, we show that attention-based models can improves the Equal Error Rate (EER) of our speaker verification system by relatively 14% compared to our non-attention LSTM baseline model.
更多查看译文
关键词
─ Attention-based model,sequence summarization,speaker recognition,pooling,LSTM
AI 理解论文
溯源树
样例
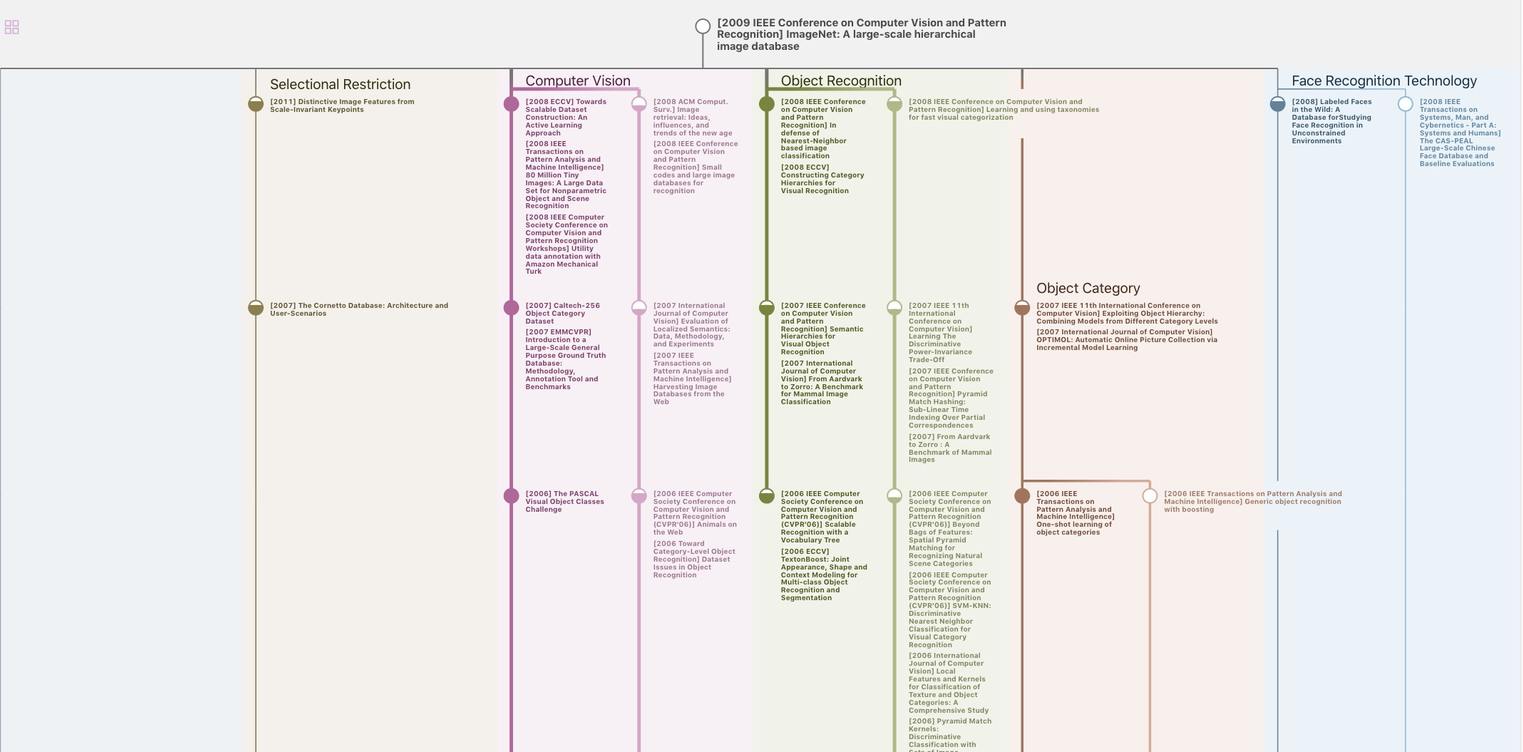
生成溯源树,研究论文发展脉络
Chat Paper
正在生成论文摘要