Multimodal and Multiscale Deep Neural Networks for the Early Diagnosis of Alzheimer’s Disease using structural MR and FDG-PET images
SCIENTIFIC REPORTS(2018)
摘要
Alzheimer’s Disease (AD) is a progressive neurodegenerative disease where biomarkers for disease based on pathophysiology may be able to provide objective measures for disease diagnosis and staging. Neuroimaging scans acquired from MRI and metabolism images obtained by FDG-PET provide in-vivo measurements of structure and function (glucose metabolism) in a living brain. It is hypothesized that combining multiple different image modalities providing complementary information could help improve early diagnosis of AD. In this paper, we propose a novel deep-learning-based framework to discriminate individuals with AD utilizing a multimodal and multiscale deep neural network. Our method delivers 82.4% accuracy in identifying the individuals with mild cognitive impairment (MCI) who will convert to AD at 3 years prior to conversion (86.4% combined accuracy for conversion within 1–3 years), a 94.23% sensitivity in classifying individuals with clinical diagnosis of probable AD, and a 86.3% specificity in classifying non-demented controls improving upon results in published literature.
更多查看译文
关键词
alzheimers,multiscale deep neural networks,structural mr,neural networks,fdg-pet
AI 理解论文
溯源树
样例
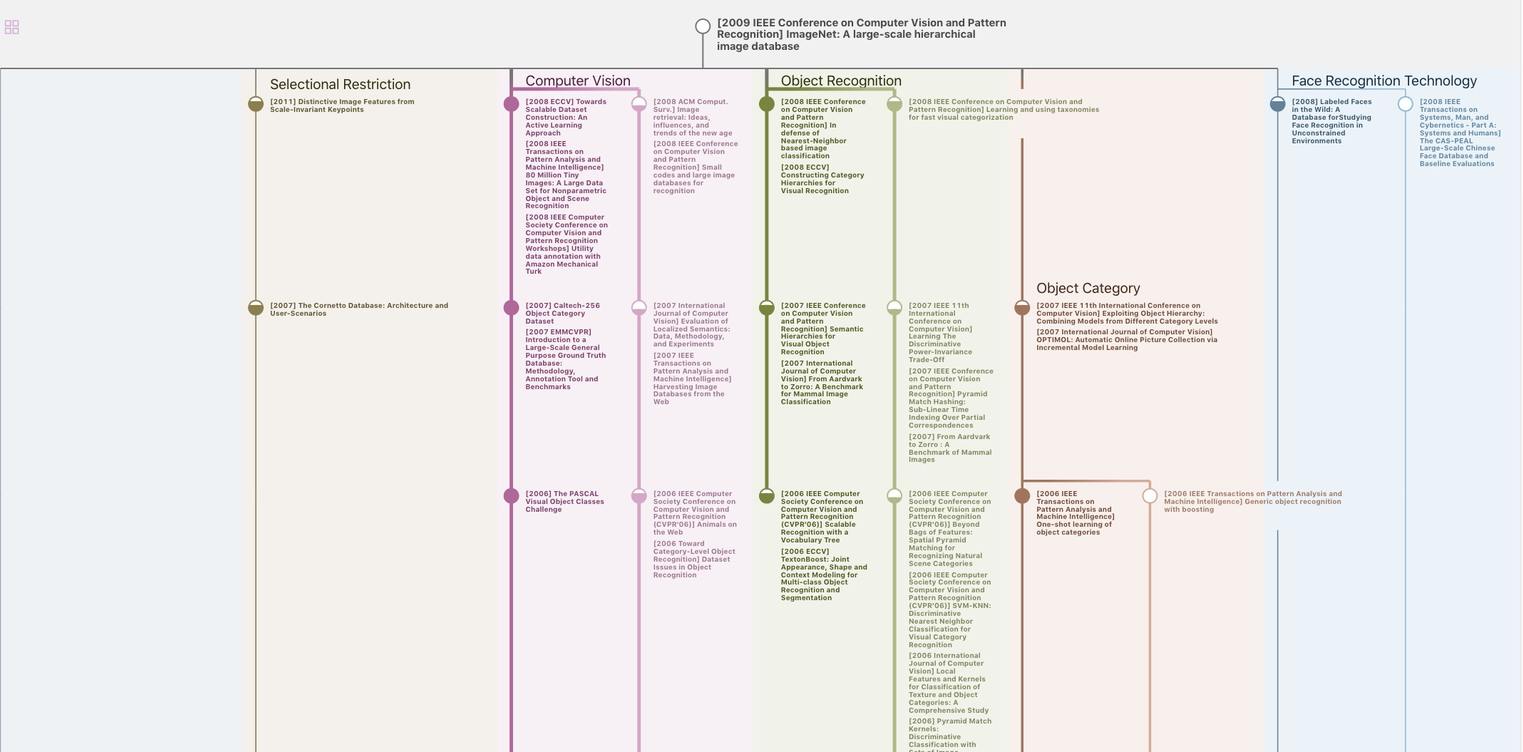
生成溯源树,研究论文发展脉络
Chat Paper
正在生成论文摘要