Cross-Modal Retrieval Using Multiordered Discriminative Structured Subspace Learning.
IEEE Trans. Multimedia(2017)
摘要
This paper proposes a novel method for cross-modal retrieval. In addition to the traditional vector (text)-to-vector (image) framework, we adopt a matrix (text)-to-matrix (image) framework to faithfully characterize the structures of different feature spaces. Moreover, we propose a novel metric learning framework to learn a discriminative structured subspace, in which the underlying data distribution is preserved for ensuring a desirable metric. Concretely, there are three steps for the proposed method. First, the multiorder statistics are used to represent images and texts for enriching the feature information. We jointly use the covariance (second-order), mean (first-order), and bags of visual (textual) features (zeroth-order) to characterize each image and text. Second, considering that the heterogeneous covariance matrices lie on the different Riemannian manifolds and the other features on the different Euclidean spaces, respectively, we propose a unified metric learning framework integrating multiple distance metrics, one for each order statistical feature. This framework preserves the underlying data distribution and exploits complementary information for better matching heterogeneous data. Finally, the similarity between the different modalities can be measured by transforming the multiorder statistical features to the common subspace. The performance of the proposed method over the previous methods has been demonstrated through the experiments on two public datasets.
更多查看译文
关键词
Correlation,Semantics,Multimedia communication,Measurement,Manifolds,Feature extraction,Data models
AI 理解论文
溯源树
样例
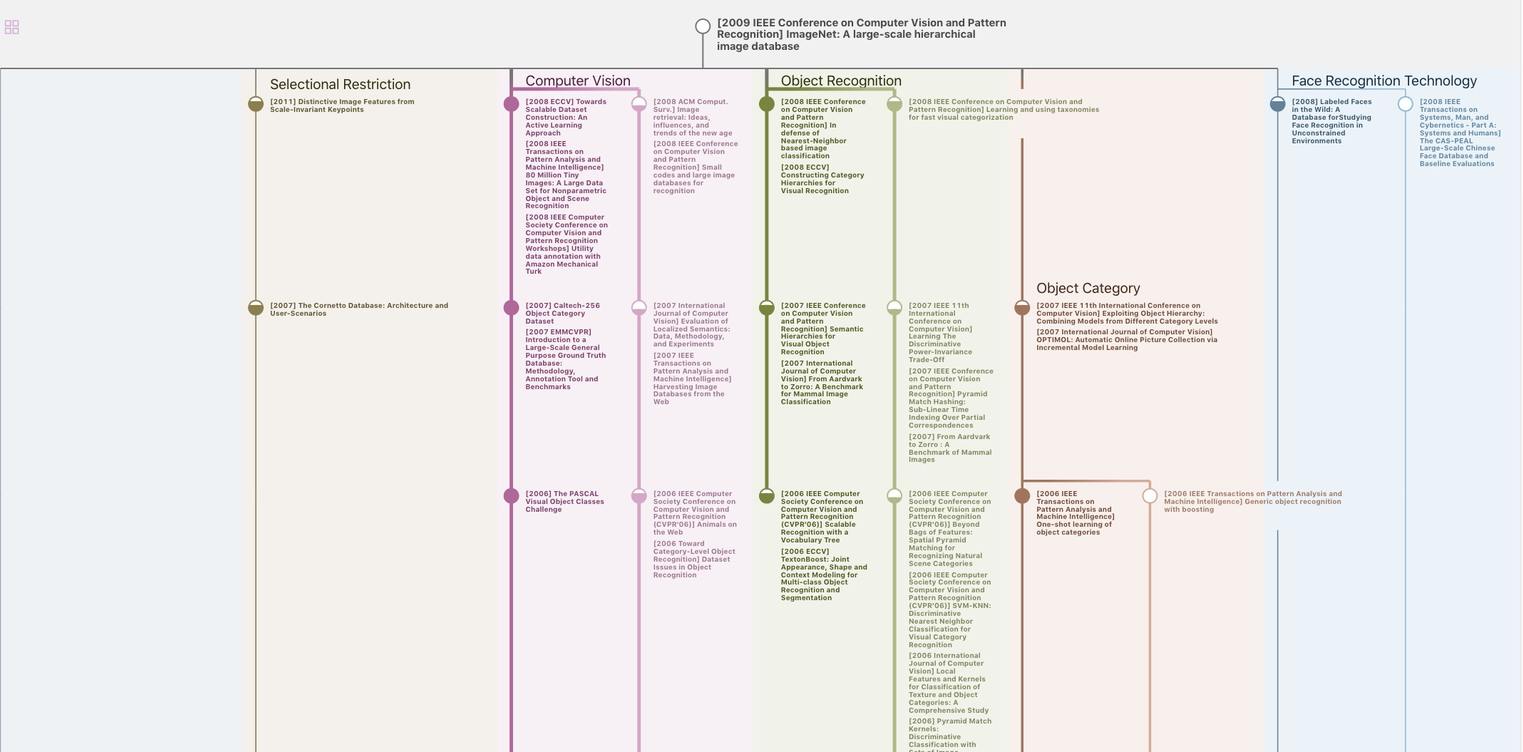
生成溯源树,研究论文发展脉络
Chat Paper
正在生成论文摘要