Learning Graphical Models from a Distributed Stream.
international conference on data engineering(2018)
摘要
A current challenge for data management systems is to support the construction and maintenance of machine learning models over data that is large, multi-dimensional, and evolving. While systems that could support these tasks are emerging, the need to scale to distributed, streaming data requires new models and algorithms. In this setting, as well as computational scalability and model accuracy, we also need to minimize the amount of communication between distributed processors, which is the chief component of latency. We study Bayesian Networks, the workhorse of graphical models, and present a communication-efficient method for continuously learning and maintaining a Bayesian network model over data that is arriving as a distributed stream partitioned across multiple processors. We show a strategy for maintaining model parameters that leads to an exponential reduction in communication when compared with baseline approaches to maintain the exact MLE (maximum likelihood estimation). Meanwhile, our strategy provides similar prediction errors for the target distribution and for classification tasks.
更多查看译文
关键词
Bayesian Networks,Distributed Stream Algorithm,Parameter Learning
AI 理解论文
溯源树
样例
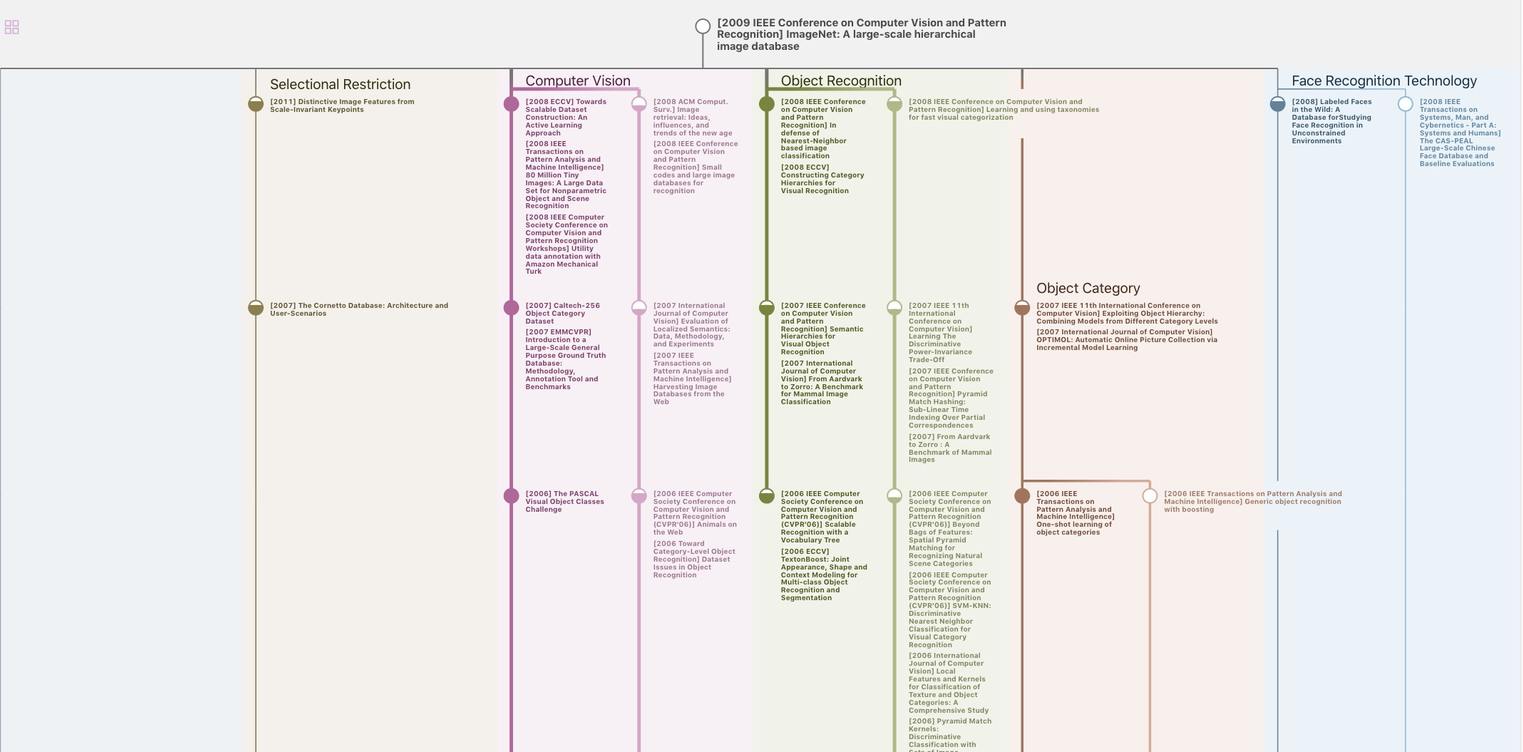
生成溯源树,研究论文发展脉络
Chat Paper
正在生成论文摘要