Investigation of Frame Alignments for GMM-based Digit-prompted Speaker Verification
Asia-Pacific Signal and Information Processing Association Annual Summit and Conference(2017)
Abstract
Frame alignments can be computed by different methods in GMM-based speaker verification. By incorporating a phonetic Gaussian mixture model ( PGMM), we are able to compare the performance using alignments extracted from the deep neural networks ( DNN) and the conventional hidden Markov model ( HMM) in digit-prompted speaker verification. Based on the different characteristics of these two alignments, we present a novel content verification method to improve the system security without much computational overhead. Our experiments on the RSR2015 Part-3 digit-prompted task show that, the DNN-based alignment performs on par with the HMM alignment. The results also demonstrate the effectiveness of the proposed Kullback-Leibler ( KL) divergence based scoring to reject speech with incorrect pass-phrases.
MoreTranslated text
Key words
frame alignments,GMM-based speaker verification,phonetic Gaussian mixture model,Kullback-Leibler divergence,RSR2015 Part-3 digit-prompted task,DNN-based alignment,content verification method,HMM alignment,computational overhead,digit-prompted speaker verification,conventional hidden Markov model,deep neural networks
AI Read Science
Must-Reading Tree
Example
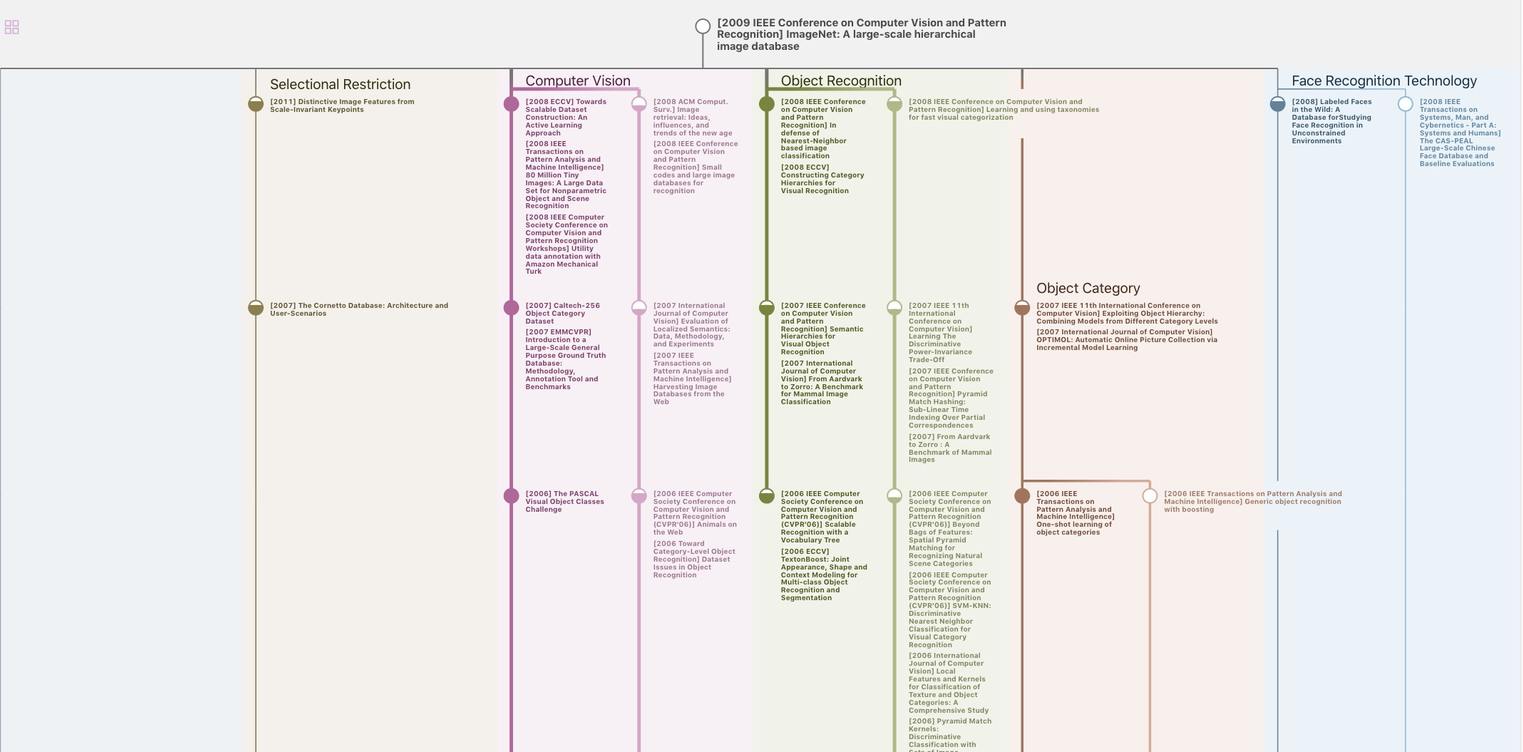
Generate MRT to find the research sequence of this paper
Chat Paper
Summary is being generated by the instructions you defined