PRM-RL: Long-range Robotic Navigation Tasks by Combining Reinforcement Learning and Sampling-based Planning
2018 IEEE International Conference on Robotics and Automation (ICRA)(2018)
摘要
We present PRM-RL, a hierarchical method for long-range navigation task completion that combines sampling based path planning with reinforcement learning (RL). The RL agents learn short-range, point-to-point navigation policies that capture robot dynamics and task constraints without knowledge of the large-scale topology. Next, the sampling-based planners provide roadmaps which connect robot configurations that can be successfully navigated by the RL agent. The same RL agents are used to control the robot under the direction of the planning, enabling long-range navigation. We use the Probabilistic Roadmaps (PRMs) for the sampling-based planner. The RL agents are constructed using feature-based and deep neural net policies in continuous state and action spaces. We evaluate PRM-RL, both in simulation and on-robot, on two navigation tasks with non-trivial robot dynamics: end-to-end differential drive indoor navigation in office environments, and aerial cargo delivery in urban environments with load displacement constraints. Our results show improvement in task completion over both RL agents on their own and traditional sampling-based planners. In the indoor navigation task, PRM-RL successfully completes up to 215 m long trajectories under noisy sensor conditions, and the aerial cargo delivery completes flights over 1000 m without violating the task constraints in an environment 63 million times larger than used in training.
更多查看译文
关键词
sampling based planner,hierarchical method,sampling based path planning,large scale topology,probabilistic roadmaps,feature based deep neural net policies,continuous state,action spaces,simulation,office environments,aerial cargo delivery,urban environments,load displacement constraints,trajectories,noisy sensor conditions,flights,training,PRM RL,long range robotic navigation tasks,point to point navigation policies,end to end differential drive indoor navigation,nontrivial robot dynamics,robot configurations,task constraints,capture robot dynamics,RL agent,reinforcement learning
AI 理解论文
溯源树
样例
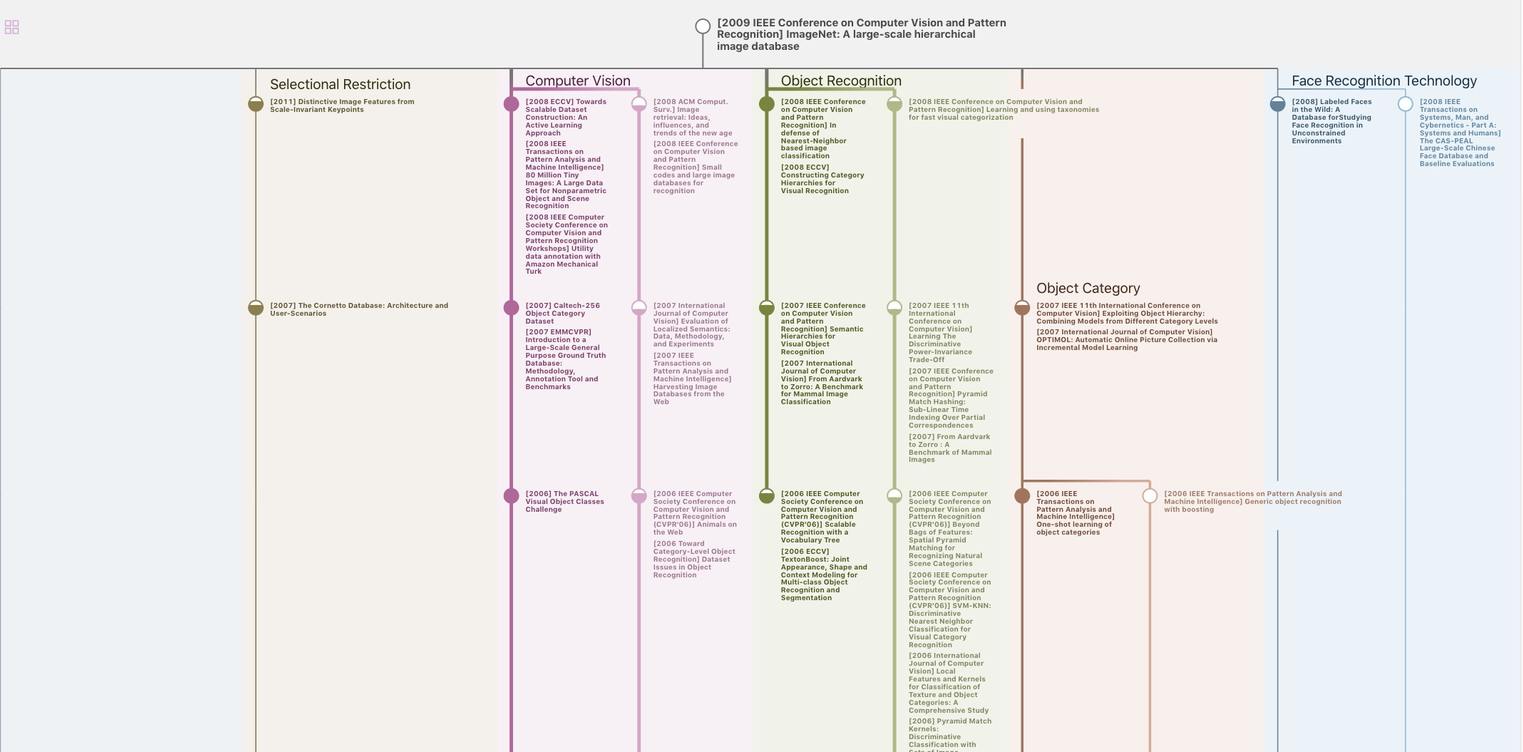
生成溯源树,研究论文发展脉络
Chat Paper
正在生成论文摘要