Automatic Ground Truths: Projected Image Annotations for Omnidirectional Vision
2017 INTERNATIONAL CONFERENCE ON DIGITAL IMAGE COMPUTING - TECHNIQUES AND APPLICATIONS (DICTA)(2017)
摘要
We present a novel data set made up of omnidirectional video of multiple objects whose centroid positions are annotated automatically. Omnidirectional vision is an active field of research focused on the use of spherical imagery in video analysis and scene understanding, involving tasks such as object detection, tracking and recognition. Our goal is to provide a large and consistently annotated video data set that can be used to train and evaluate new algorithms for these tasks. Here we describe the experimental setup and software environment used to capture and map the 3D ground truth positions of multiple objects into the image. Furthermore, we estimate the expected systematic error on the mapped positions. In addition to final data products, we release publicly the software tools and raw data necessary to re-calibrate the camera and/or redo this mapping. The software also provides a simple framework for comparing the results of standard image annotation tools or visual tracking systems against our mapped ground truth annotations.
更多查看译文
关键词
software tools,raw data,standard image annotation tools,mapped ground truth annotations,automatic ground truths,image annotations,omnidirectional vision,omnidirectional video,multiple objects,centroid positions,spherical imagery,video analysis,video data,expected systematic error,mapped positions,final data products
AI 理解论文
溯源树
样例
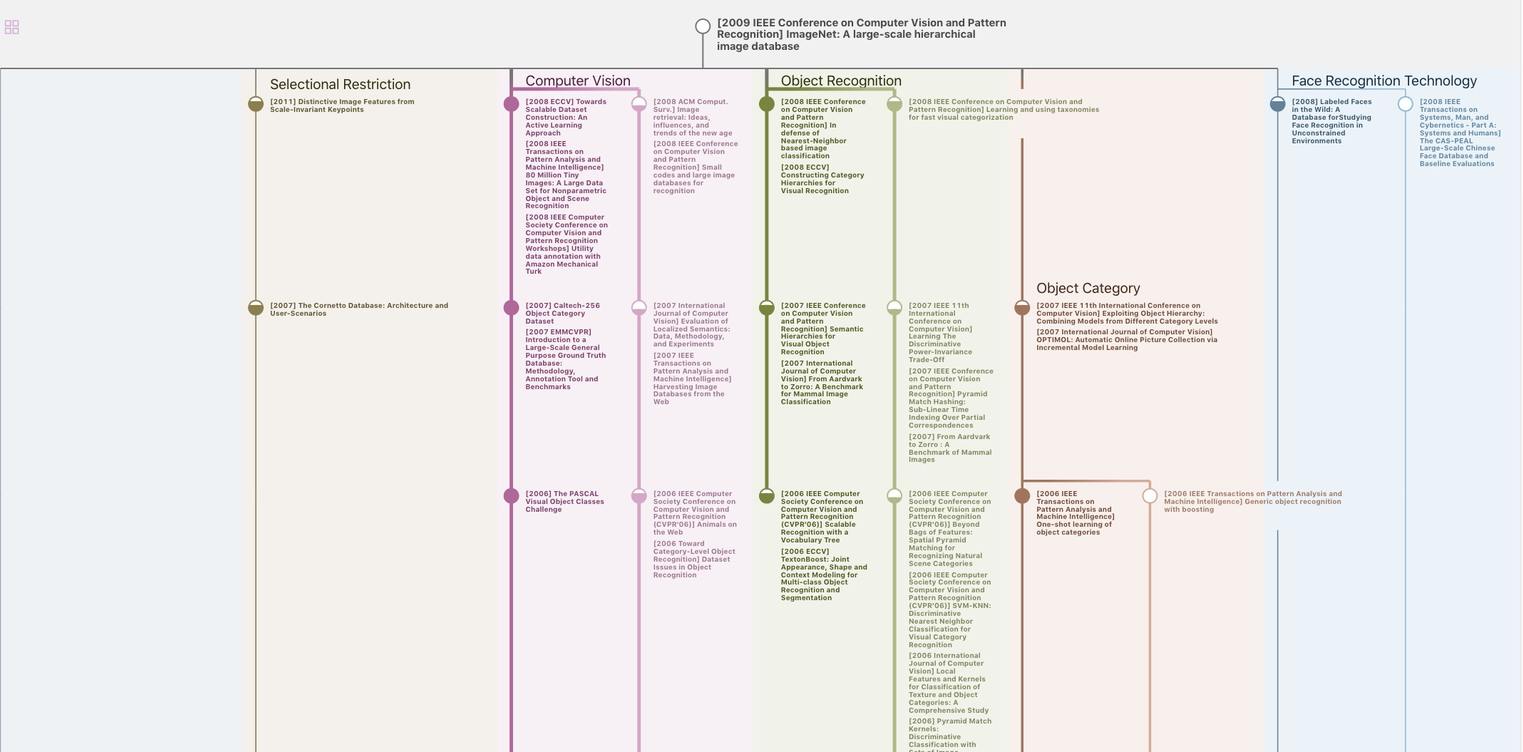
生成溯源树,研究论文发展脉络
Chat Paper
正在生成论文摘要