Lower bounds for the low-rank matrix approximation
Journal of inequalities and applications(2017)
摘要
Low-rank matrix recovery is an active topic drawing the attention of many researchers. It addresses the problem of approximating the observed data matrix by an unknown low-rank matrix. Suppose that A is a low-rank matrix approximation of D , where D and A are m × n matrices. Based on a useful decomposition of D^† - A^† , for the unitarily invariant norm · , when D≥A and D≤A , two sharp lower bounds of D - A are derived respectively. The presented simulations and applications demonstrate our results when the approximation matrix A is low-rank and the perturbation matrix is sparse.
更多查看译文
关键词
approximation,error estimation,low-rank matrix,matrix norms,pseudo-inverse
AI 理解论文
溯源树
样例
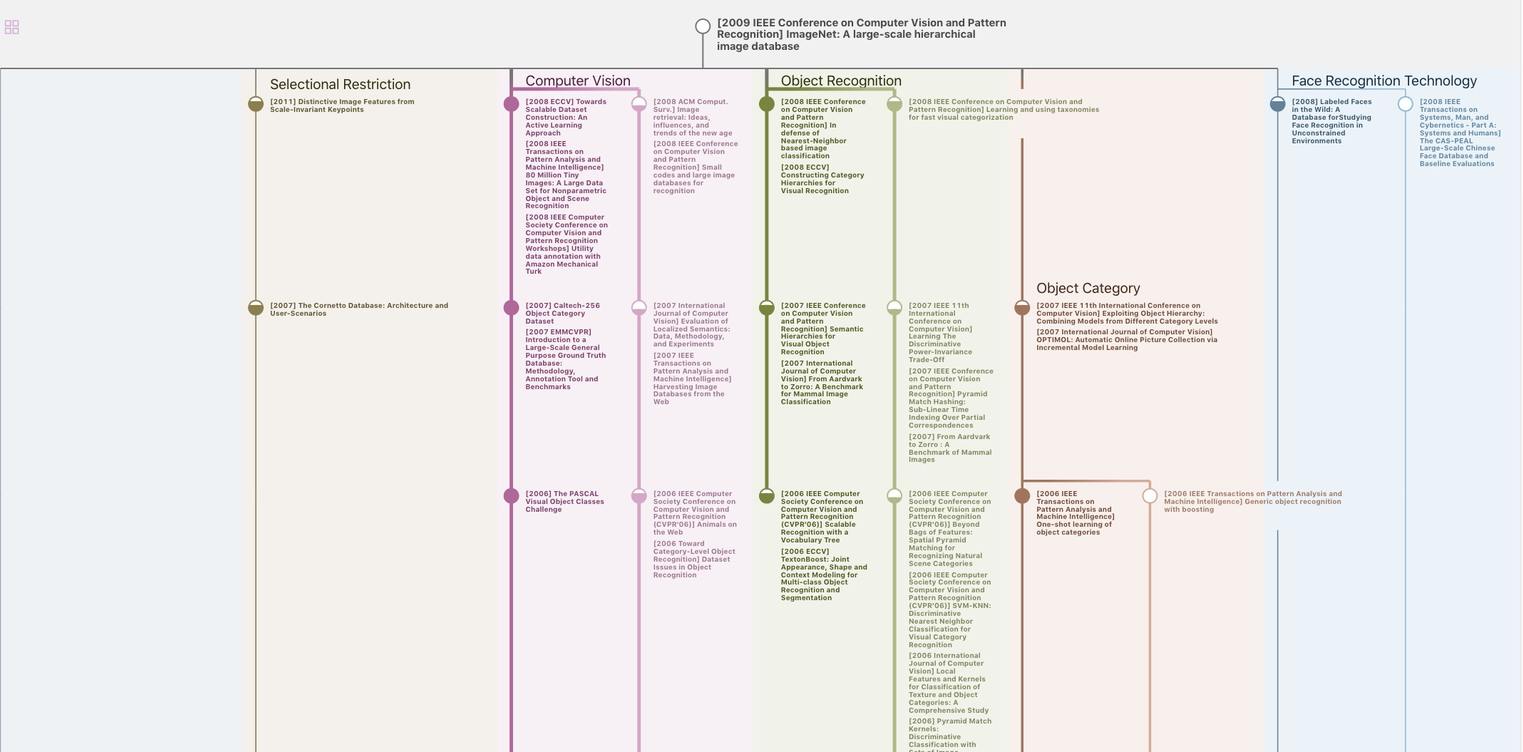
生成溯源树,研究论文发展脉络
Chat Paper
正在生成论文摘要