Big Data Analytical Approaches to the NACC Dataset: Aiding Preclinical Trial Enrichment.
ALZHEIMER DISEASE & ASSOCIATED DISORDERS(2018)
摘要
Background:Clinical trials increasingly aim to retard disease progression during presymptomatic phases of Mild Cognitive Impairment (MCI) and thus recruiting study participants at high risk for developing MCI is critical for cost-effective prevention trials. However, accurately identifying those who are destined to develop MCI is difficult. Collecting biomarkers is often expensive.Methods:We used only noninvasive clinical variables collected in the National Alzheimer's Coordinating Center (NACC) Uniform Data Sets version 2.0 and applied machine learning techniques to build a low-cost and accurate Mild Cognitive Impairment (MCI) conversion prediction calculator. Cross-validation and bootstrap were used to select as few variables as possible accurately predicting MCI conversion within 4 years.Results:A total of 31,872 unique subjects, 748 clinical variables, and additional 128 derived variables in NACC data sets were used. About 15 noninvasive clinical variables are identified for predicting MCI/aMCI/naMCI converters, respectively. Over 75% Receiver Operating Characteristic Area Under the Curves (ROC AUC) was achieved. By bootstrap we created a simple spreadsheet calculator which estimates the probability of developing MCI within 4 years with a 95% confidence interval.Conclusions:We achieved reasonably high prediction accuracy using only clinical variables. The approach used here could be useful for study enrichment in preclinical trials where enrolling participants at risk of cognitive decline is critical for proving study efficacy, and also for developing a shorter assessment battery.
更多查看译文
关键词
study enrichment,National Alzheimer's Coordinating Center Uniform Data Set (NACC UDS),mild cognitive impairment,incidence,prediction,dementia,bootstrap,machine learning,ROC AUC
AI 理解论文
溯源树
样例
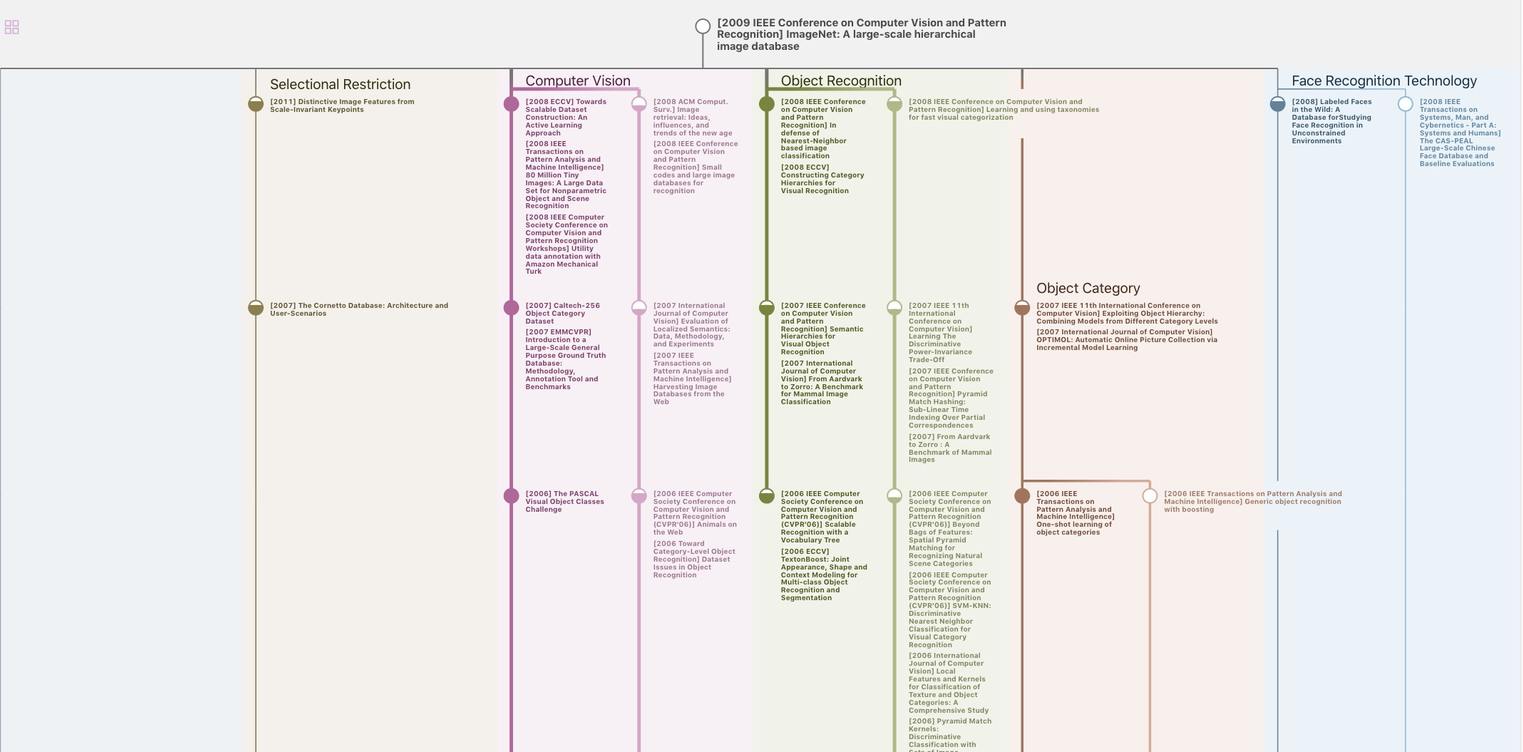
生成溯源树,研究论文发展脉络
Chat Paper
正在生成论文摘要