Autonomous Meta-Classifier For Surface Hardness Classification From Uav Landings
2017 IEEE/RSJ INTERNATIONAL CONFERENCE ON INTELLIGENT ROBOTS AND SYSTEMS (IROS)(2017)
摘要
Developing surface classification models manually requires significant time and detracts from the goal of automating systems. We create a system that automatically collects the data using an Unmanned Aerial Vehicle (UAV), extracts features, trains a large number of classifiers, selects the best classifier, and programs the UAV with that classifier. Motivating our work is a prior project [1] that manually developed a surface classifier using an accelerometer; to verify our system functionality, we replicate those results with our new automated system and improve on those results, providing a four-surface classifier with a 75% classification rate and a hard/soft classifier with a 100% classification rate. We further verify our system through a field experiment that collects and classifies new data, proving its end-to-end functionality. Overall, our system reduces the time and machine learning expertise needed by the user to develop new time-series classifiers usable by the UAV. The general form of our system provides a valuable tool for automation of classifier creation and is released as an open-source tool [2].
更多查看译文
关键词
feature extraction,machine learning expertise,time-series classifiers,Unmanned Aerial Vehicle,surface classification models,UAV landings,surface hardness classification,autonomous meta-classifier
AI 理解论文
溯源树
样例
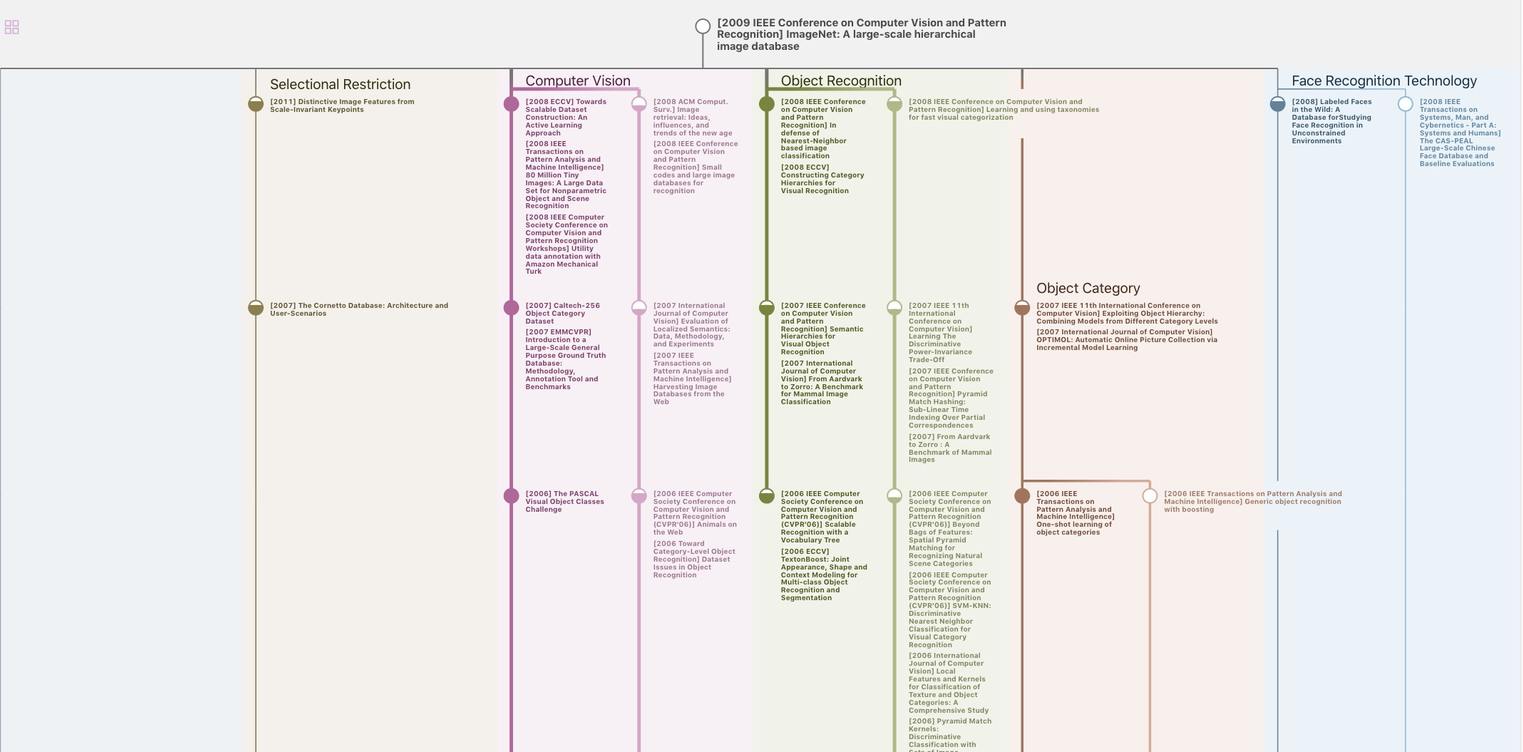
生成溯源树,研究论文发展脉络
Chat Paper
正在生成论文摘要