Unmixing In The Presence Of Nuisances With Deep Generative Models
2017 IEEE INTERNATIONAL GEOSCIENCE AND REMOTE SENSING SYMPOSIUM (IGARSS)(2017)
摘要
Spectral datasets acquired for unmixing are noisy and largely unlabeled (with unknown abundances). As a result the ability to accurately predict endmember abundances of surface samples is as important as the capacity to generate spectra from hypothetical abundances, e.g. endmembers from abundances sampled from the corner of a simplex. We construct a deep (semi-supervised) generative model to accomplish both these tasks while making use of the readily available unlabeled spectra and being able to encode environmental and instrumental nuisances. Our main technical contribution is that we train our model both forward and in reverse. The algorithm successfully identifies endmember spectra while isolating photometric effects and imaging errors as nuisances in a real dataset of intimately mixed samples.
更多查看译文
关键词
Deep generative models, variational autoencoders, hyperspectral data unmixing
AI 理解论文
溯源树
样例
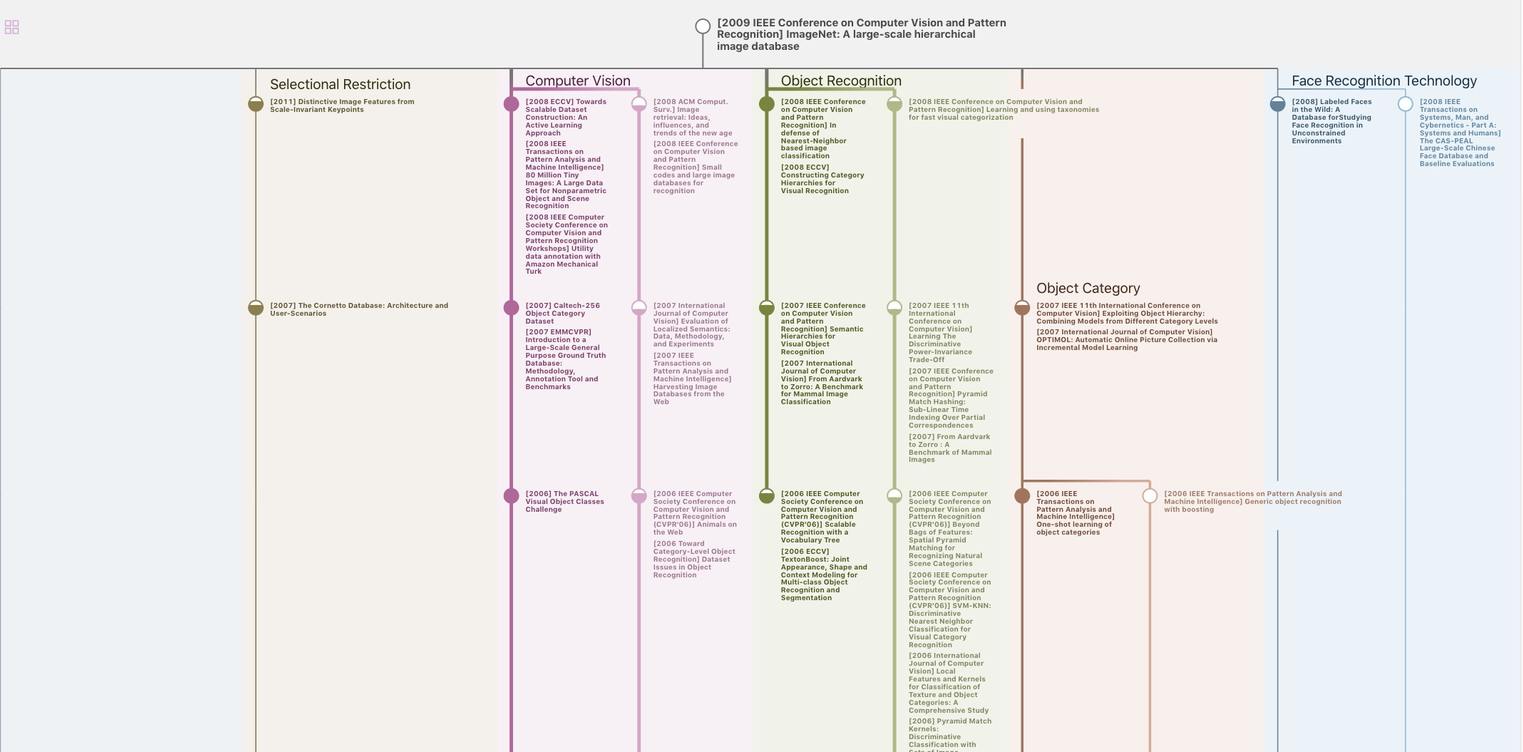
生成溯源树,研究论文发展脉络
Chat Paper
正在生成论文摘要