Exploiting Efficiency Opportunities Based On Workloads With Electron On Heterogeneous Clusters
PROCEEDINGS OF THE 10TH INTERNATIONAL CONFERENCE ON UTILITY AND CLOUD COMPUTING (UCC' 17)(2017)
摘要
Resource Management tools for large-scale clusters and data centers typically schedule resources based on task requirements specified in terms of processor, memory, and disk space. As these systems scale, two non-traditional resources also emerge as limiting factors: power and energy. Maintaining a low power envelope is especially important during Coincidence Peak, a window of time where power may cost up to 200 times the base rate. Using Electron, our power-aware framework that leverages Apache Mesos as a resource broker, we quantify the impact of four scheduling policies on three workloads of varying power intensity. We also quantify the impact of two dynamic power capping strategies on power consumption, energy consumption, and makespan when used in combination with scheduling policies across workloads. Our experiments show that choosing the right combination of scheduling and power capping policies can lead to a 16% reduction of energy and a 37% reduction in the 99th percentile of power consumption while having a negligible impact on makespan and resource utilization.
更多查看译文
关键词
Apche Mesos, Power, Energy, Efficiency, RAPL, Heterogeneous
AI 理解论文
溯源树
样例
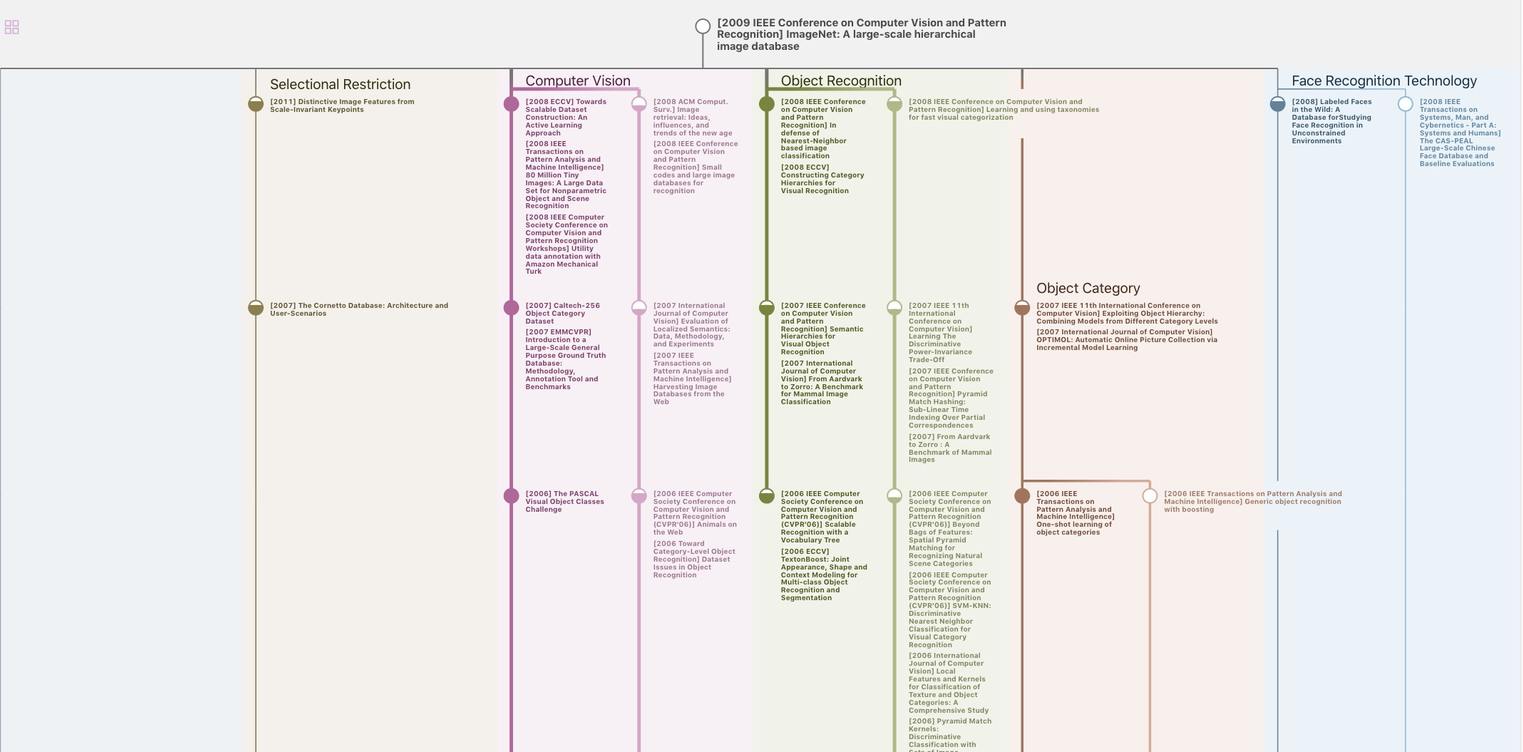
生成溯源树,研究论文发展脉络
Chat Paper
正在生成论文摘要