Computer aided analysis of prostate histopathology images to support a refined Gleason grading system.
Proceedings of SPIE(2017)
摘要
The Gleason grading system used to render prostate cancer diagnosis has recently been updated to allow more accurate grade stratification and higher prognostic discrimination when compared to the traditional grading system. In spite of progress made in trying to standardize the grading process, there still remains approximately a 30% grading discrepancy between the score rendered by general pathologists and those provided by experts while reviewing needle biopsies for Gleason pattern 3 and 4, which accounts for more than 70% of daily prostate tissue slides at most institutions. We propose a new computational imaging method for Gleason pattern 3 and 4 classification, which better matches the newly established prostate cancer grading system. The computer aided analysis method includes two phases. First, the boundary of each glandular region is automatically segmented using a deep convolutional neural network. Second, color, shape and texture features are extracted from superpixels corresponding to the outer and inner glandular regions and are subsequently forwarded to a random forest classifier to give a gradient score between 3 and 4 for each delineated glandular region. The F-1 score for glandular segmentation is 0.8460 and the classification accuracy is 0.83 +/- 0.03.
更多查看译文
关键词
Gleason grading,histopathology segmentation,convolutional neural network,random forest,regression
AI 理解论文
溯源树
样例
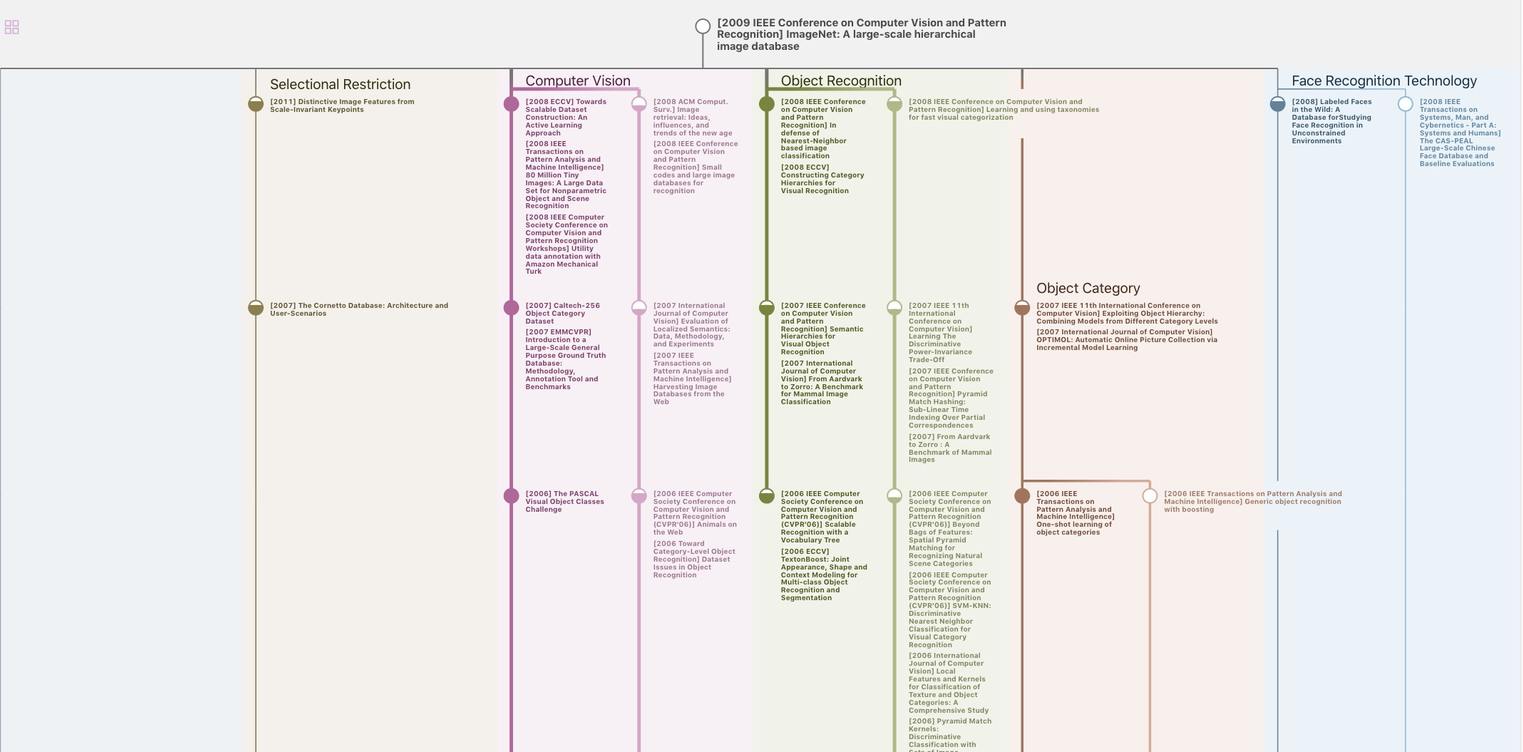
生成溯源树,研究论文发展脉络
Chat Paper
正在生成论文摘要