Exploring Transfer Learning For Crime Prediction
2017 17TH IEEE INTERNATIONAL CONFERENCE ON DATA MINING WORKSHOPS (ICDMW 2017)(2017)
摘要
Crime prediction plays a crucial role in addressing crime, violence, conflict and insecurity in cities to promote good governance, appropriate urban planning and management. Plenty efforts have been made on developing crime prediction models by leveraging demographic data, but they failed to capture the dynamic nature of crimes in urban. Recently, with the development of new techniques for collecting and integrating fine-grained crime-related datasets, there is a potential to obtain better understandings about the dynamics of crimes and advance crime prediction. However, for a city, it is hard to build a uniform framework for all boroughs due to the uneven distribution of data. To this end, in this paper, we exploit spatio-temporal patterns in urban data in one borough in a city, and then leverage transfer learning techniques to reinforce the crime prediction of other boroughs. Specifically, we first validate the existence of spatio-temporal patterns in urban crime. Then we extract the crime-related features from cross-domain datasets. Finally we propose a novel transfer learning framework to integrate these features and model spatio-temporal patterns for crime prediction.
更多查看译文
关键词
Crime Prediction, Transfer Learning, Spatio-Temporal Patterns
AI 理解论文
溯源树
样例
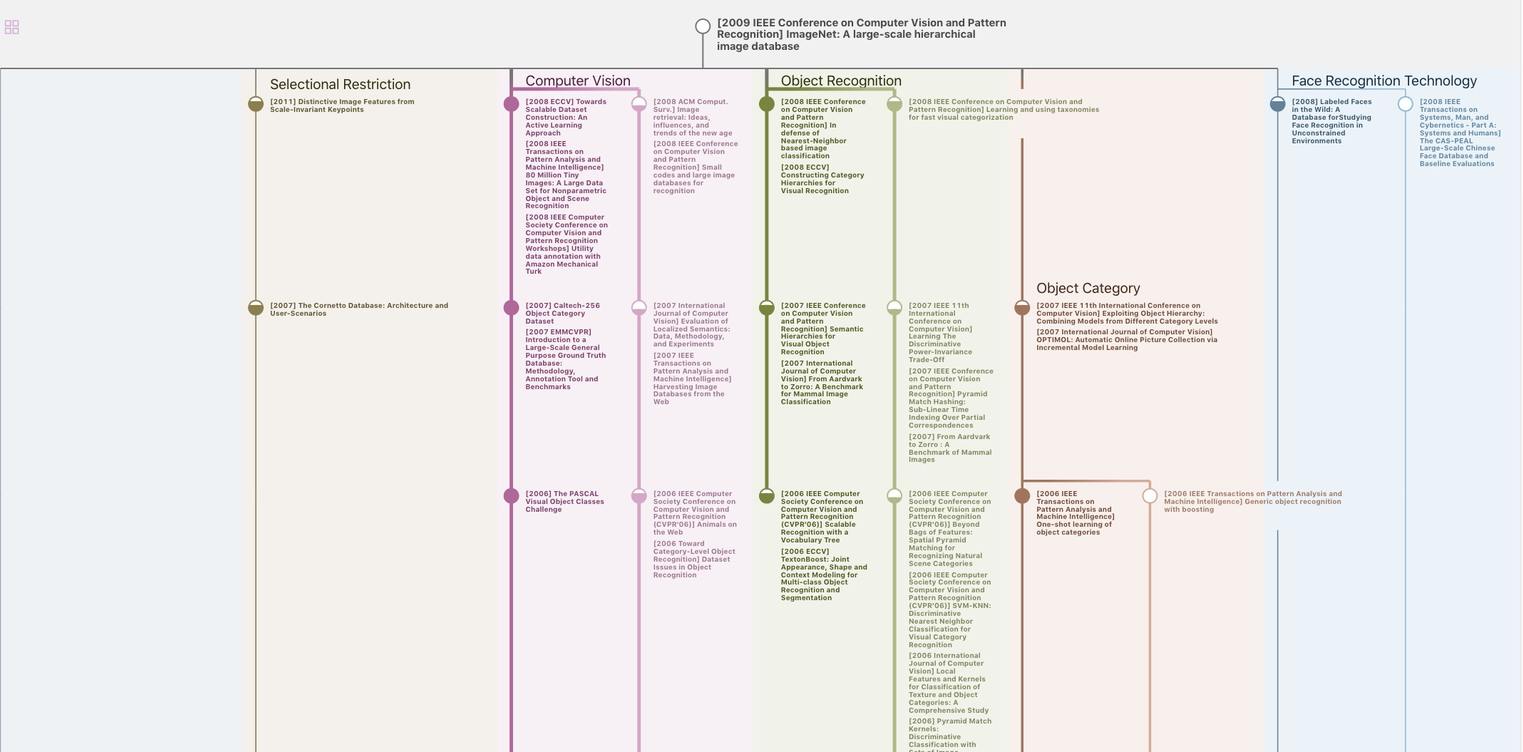
生成溯源树,研究论文发展脉络
Chat Paper
正在生成论文摘要