Improving multivariate time series forecasting with random walks with restarts on causality graphs
2017 17TH IEEE INTERNATIONAL CONFERENCE ON DATA MINING WORKSHOPS (ICDMW 2017)(2017)
摘要
Forecasting models that utilize multiple predictors are gaining popularity in a variety of fields. In some cases they allow constructing more precise forecasting models, leveraging the predictive potential of many variables. Unfortunately, in practice we do not know which observed predictors have a direct impact on the target variable. Moreover, adding unrelated variables may diminish the quality of forecasts. Thus, constructing a set of predictor variables that can be used in a forecast model is one of the greatest challenges in forecasting. We propose a new selection model for predictor variables based on the directed causality graph and a modification of the random walk with restarts model. Experiments conducted using the two popular macroeconomics sets, from the US and Australia, show that this simple and scalable approach performs well compared to other well established methods.
更多查看译文
关键词
Economic forecasting,Forecasting,Time series analysis,Data mining,Machine learning
AI 理解论文
溯源树
样例
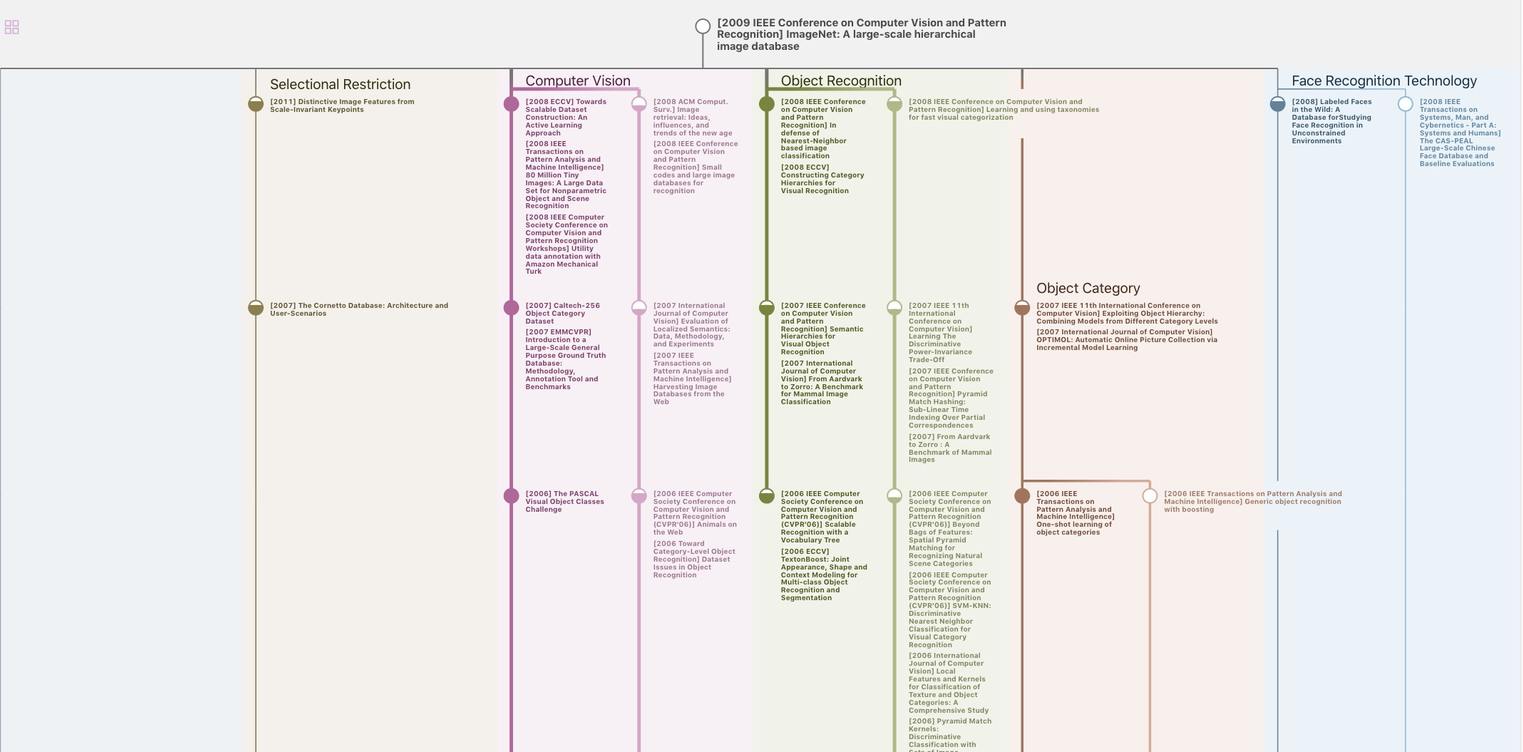
生成溯源树,研究论文发展脉络
Chat Paper
正在生成论文摘要