Inverse Solutions For Brain-Computer Interfaces: Effects Of Regularisation On Localisation And Classification
2017 IEEE INTERNATIONAL CONFERENCE ON SYSTEMS, MAN, AND CYBERNETICS (SMC)(2017)
摘要
Estimation of intracranial sources, using inverse solutions methods, has been proposed as a mean to improve performance in non-invasive brain-computer interfaces. These methods estimate the activity of a large number of neural sources from a smaller number of scalp electroencephalography (EEG) channels. This is a highly undetermined problem and regularisation constraints need to be applied. In this paper we compared the effect of several regularisation constraints and parameters in the localisation error and classification performance. Results on three event-related potential protocols -rapid serial visual processing, P300-speller and error-related potentials- showed no significant difference in the maximum performance between minimum norm or weighted minimum norm regularisation constraints. Standardised methods despite yielding lower localisation error resulted in decreased classification performance. Noteworthy, testing on data acquired in different days than the training suggests that discriminant features extracted from intracranial sources are stable across sessions.
更多查看译文
关键词
brain-computer interfaces,event-related potential protocols,EEG channels,serial visual processing,feature extraction,scalp electroencephalography channels,inverse solutions methods,intracranial sources
AI 理解论文
溯源树
样例
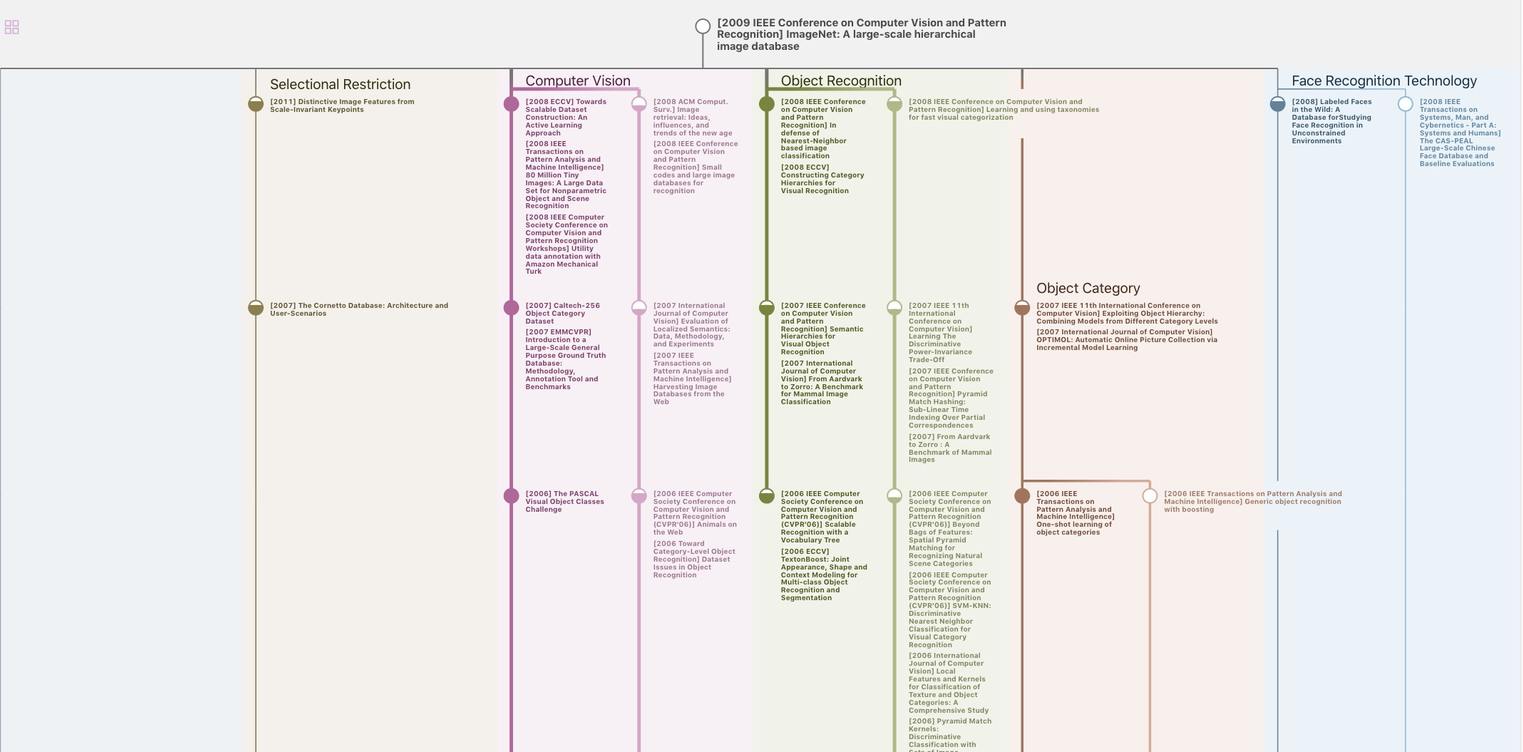
生成溯源树,研究论文发展脉络
Chat Paper
正在生成论文摘要