Decision-Theoretic Planning Under Uncertainty For Multimodal Human-Robot Interaction
2017 26TH IEEE INTERNATIONAL SYMPOSIUM ON ROBOT AND HUMAN INTERACTIVE COMMUNICATION (RO-MAN)(2017)
摘要
This paper proposes a Decision-Theoretic approach to problems involving interaction between robot systems and human users, which takes into account the latent aspects of Human-Robot interaction, e.g., the user's status. The presented approach is based on the Partially Observable Markov Decision Process framework, which handles uncertainty in planning problems, extended with information rewards to optimize the information-gathering capabilities of the system. The approach is formalized into a framework which considers: observable and latent state variables; gesture and speech observations; and action factors which are related to the agent's actuators or to the information gain goals (Information-Reward actions). Under the proposed framework, the robot system is able to: actively gain information and react according to latent states, inherent to Human-Robot interaction settings; effectively achieve the goals of the task in which the robot is employed; and follow a socially appealing behavior. Finally, the framework was thoroughly tested in a socially assistive scenario, in a realistic apartment testbed and resorting to an autonomous mobile social robot. The experiments' results validate the proposed approach for problems involving robot systems in Human Robot interaction scenarios.
更多查看译文
关键词
robot system,human users,information-gathering capabilities,speech observations,Information-Reward actions,Human-Robot interaction settings,autonomous mobile social robot,Decision-theoretic planning,Markov Decision Process framework,gesture observations
AI 理解论文
溯源树
样例
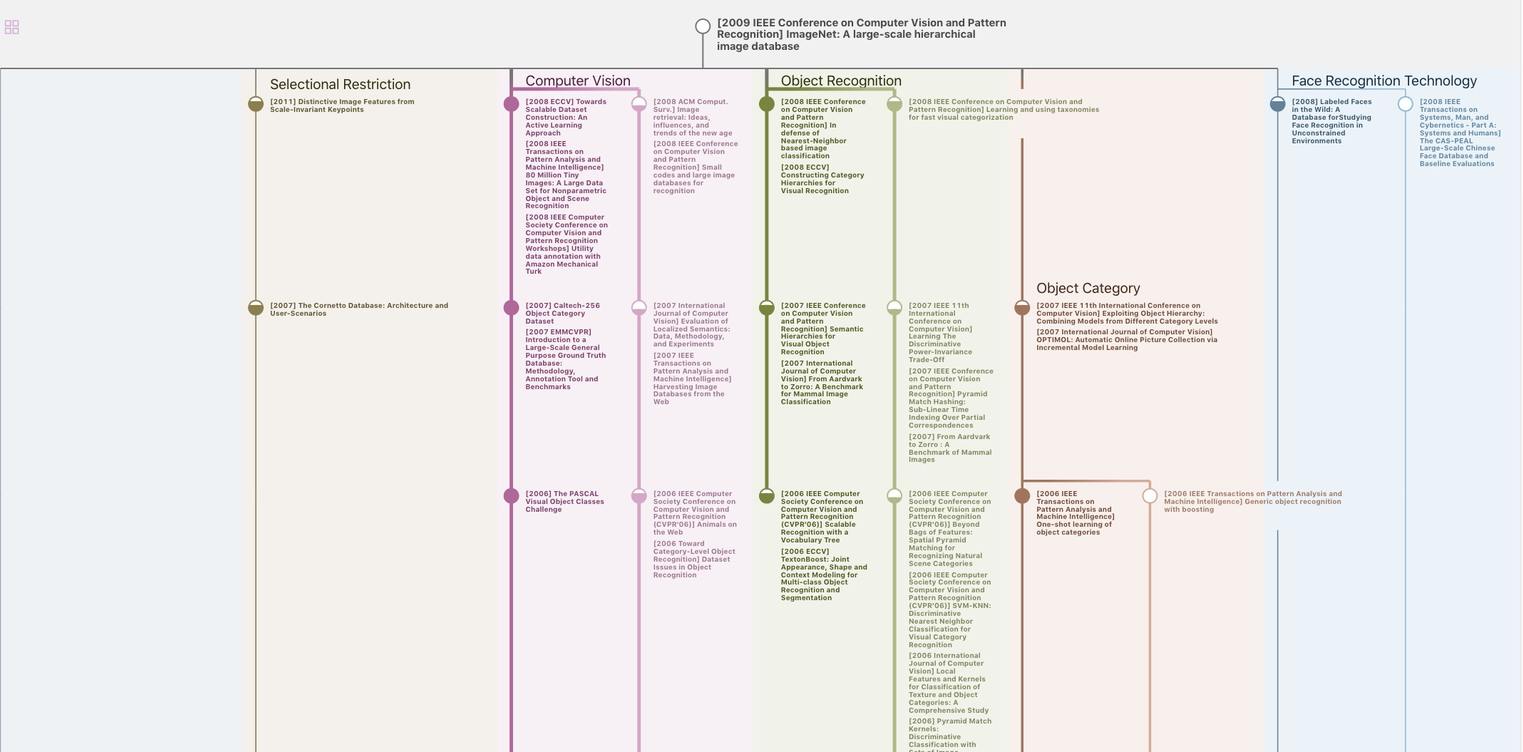
生成溯源树,研究论文发展脉络
Chat Paper
正在生成论文摘要