STARK: Structured Dictionary Learning Through Rank-one Tensor Recovery
2017 IEEE 7th International Workshop on Computational Advances in Multi-Sensor Adaptive Processing (CAMSAP)(2017)
Abstract
In recent years, a class of dictionaries have been proposed for multidimensional (tensor) data representation that exploit the structure of tensor data by imposing a Kronecker structure on the dictionary underlying the data. In this work, a novel algorithm called "STARK" is provided to learn Kronecker structured dictionaries that can represent tensors of any order. By establishing that the Kronecker product of any number of matrices can be rearranged to form a rank-1 tensor, we show that Kronecker structure can be enforced on the dictionary by solving a rank-1 tensor recovery problem. Because rank-1 tensor recovery is a challenging nonconvex problem, we resort to solving a convex relaxation of this problem. Empirical experiments on synthetic and real data show promising results for our proposed algorithm.
MoreTranslated text
Key words
structured dictionary learning,tensor data representation,STARK algorithm,matrices,nonconvex problem,convex relaxation,tensor data structure,multidimensional data representation,rank-one tensor recovery,rank-1 tensor recovery problem,Kronecker product,Kronecker structured dictionaries,Kronecker structure
AI Read Science
Must-Reading Tree
Example
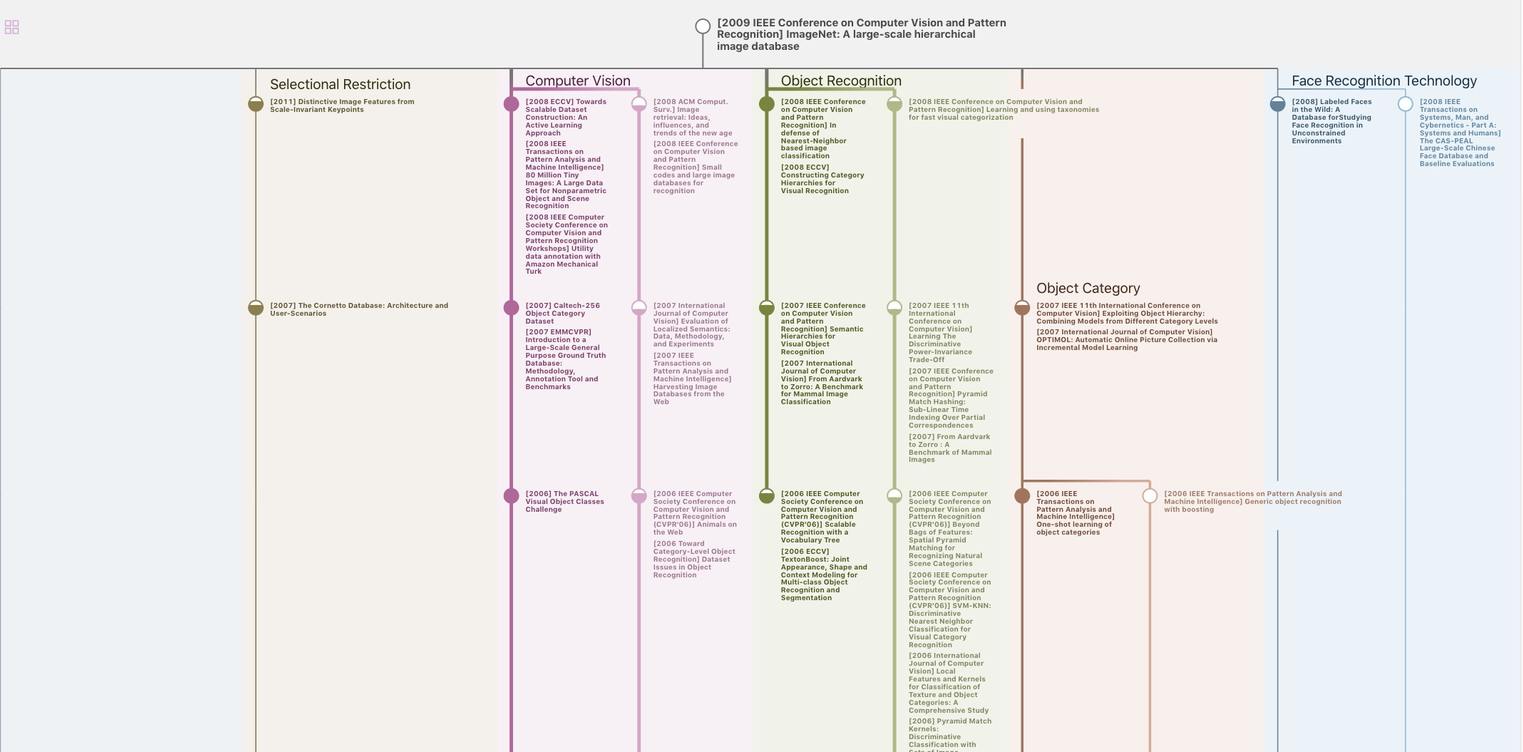
Generate MRT to find the research sequence of this paper
Chat Paper
Summary is being generated by the instructions you defined