Modular Continual Learning in a Unified Visual Environment.
arXiv (Cornell University)(2017)
摘要
A core aspect of human intelligence is the ability to learn new tasks quickly and switch between them flexibly. Here, we describe a modular continual reinforcement learning paradigm inspired by these abilities. We first introduce a visual interaction environment that allows many types of tasks to be unified in a single framework. We then describe a reward map prediction scheme that learns new tasks robustly in the very large state and action spaces required by such an environment. We investigate how properties of module architecture influence efficiency of task learning, showing that a module motif incorporating specific design principles (e.g. early bottlenecks, low-order polynomial nonlinearities, and symmetry) significantly outperforms more standard neural network motifs, needing fewer training examples and fewer neurons to achieve high levels of performance. Finally, we present a meta-controller architecture for task switching based on a dynamic neural voting scheme, which allows new modules to use information learned from previously-seen tasks to substantially improve their own learning efficiency.
更多查看译文
关键词
Working Memory,Brain-inspired Computing
AI 理解论文
溯源树
样例
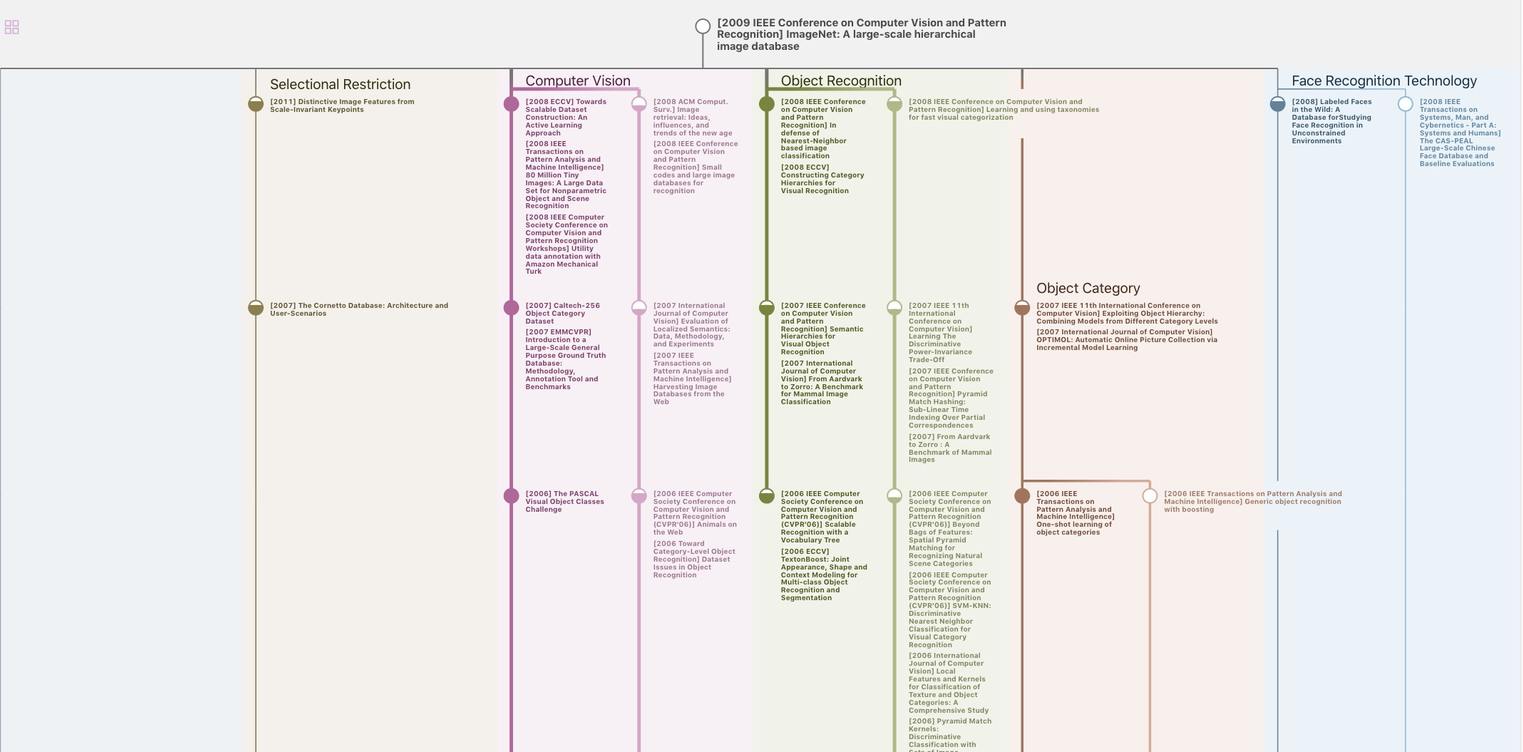
生成溯源树,研究论文发展脉络
Chat Paper
正在生成论文摘要