Flash Points: Discovering Exceptional Pairwise Behaviors in Vote or Rating Data.
Lecture Notes in Artificial Intelligence(2017)
摘要
We address the problem of discovering contexts that lead well-distinguished collections of individuals to change their pairwise agreement w.r.t. their usual one. For instance, in the European parliament, while in overall, a strong disagreement is witnessed between deputies of the far-right French party Front National and deputies of the left party Front de Gauche, a strong agreement is observed between these deputies in votes related to the thematic: External relations with the union. We devise the method DSC (Discovering Similarities Changes) which relies on exceptional model mining to uncover three-set patterns that identify contexts and two collections of individuals where an unexpected strengthening or weakening of pairwise agreement is observed. To efficiently explore the search space, we define some closure operators and pruning techniques using upper bounds on the quality measure. In addition of handling usual attributes (e.g. numerical, nominal), we propose a novel pattern domain which involves hierarchical multi-tag attributes that are present in many datasets. A thorough empirical study on two real-world datasets (i.e., European parliament votes and collaborative movie reviews) demonstrates the efficiency and the effectiveness of our approach as well as the interest and the actionability of the patterns.
更多查看译文
关键词
Exceptional model mining,Subgroup discovery
AI 理解论文
溯源树
样例
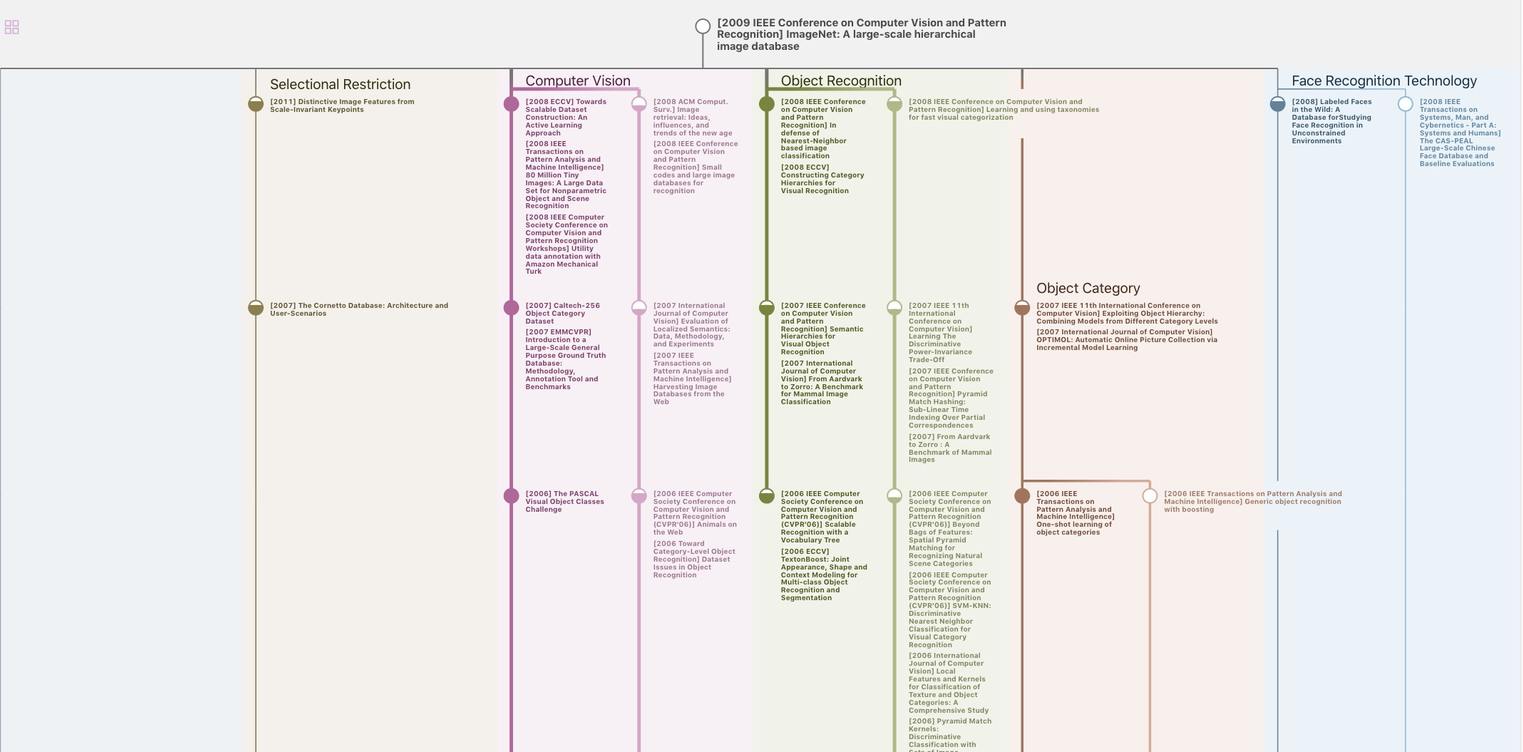
生成溯源树,研究论文发展脉络
Chat Paper
正在生成论文摘要