Predicting Success in Goal-Driven Human-Human Dialogues.
SIGDIAL Conference(2017)
Abstract
In goal-driven dialogue systems, success is often defined based on a structured definition of the goal.This requires that the dialogue system be constrained to handle a specific class of goals and that there be a mechanism to measure success with respect to that goal.However, in many human-human dialogues the diversity of goals makes it infeasible to define success in such a way.To address this scenario, we consider the task of automatically predicting success in goal-driven human-human dialogues using only the information communicated between participants in the form of text.We build a dataset from stackoverflow.com which consists of exchanges between two users in the technical domain where groundtruth success labels are available.We then propose a turn-based hierarchical neural network model that can be used to predict success without requiring a structured goal definition.We show this model outperforms rule-based heuristics and other baselines as it is able to detect patterns over the course of a dialogue and capture notions such as gratitude.
MoreTranslated text
Key words
Spoken Dialogue Systems,Dialog Management,Topic Modeling
AI Read Science
Must-Reading Tree
Example
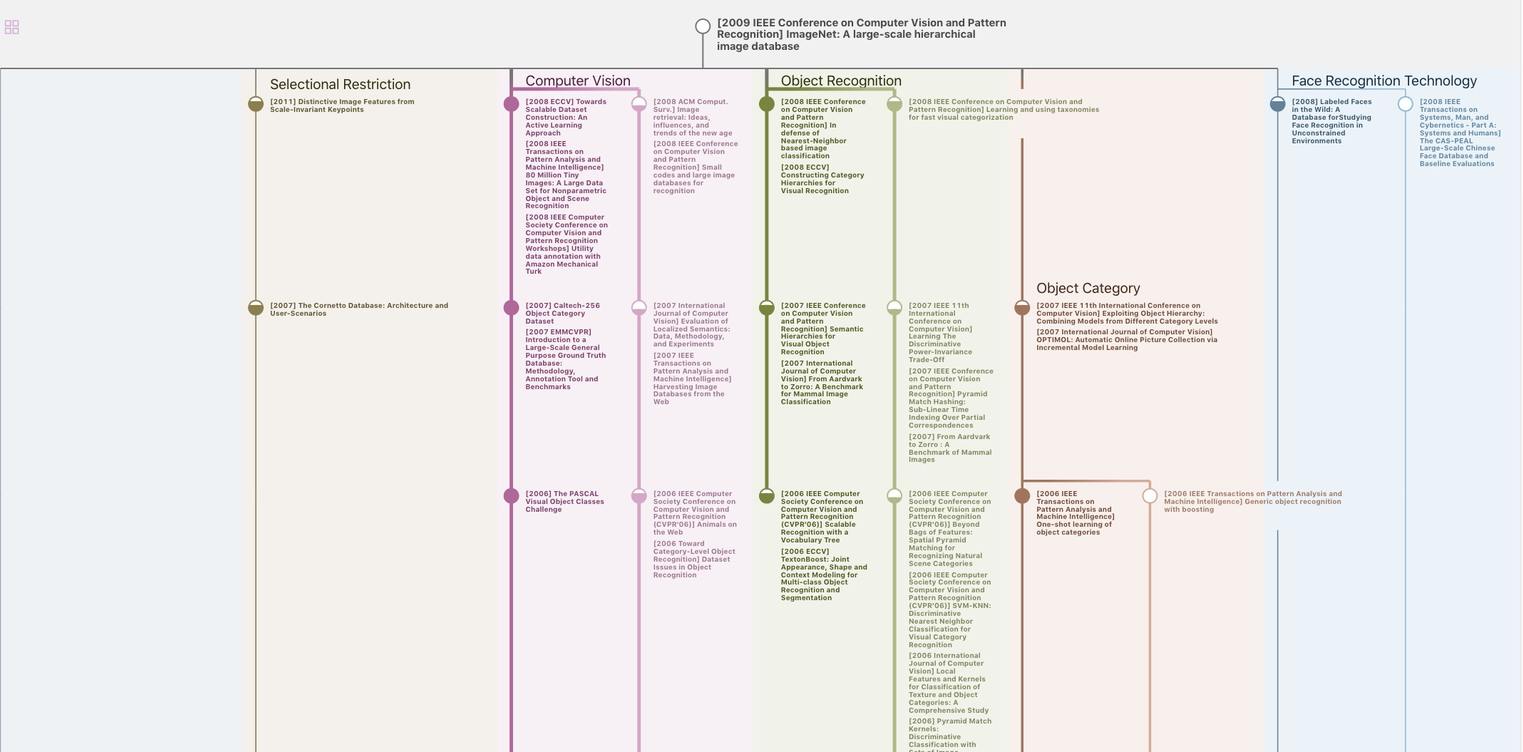
Generate MRT to find the research sequence of this paper
Chat Paper
Summary is being generated by the instructions you defined