Exploring Spatial Context for 3D Semantic Segmentation of Point Clouds
2017 IEEE International Conference on Computer Vision Workshops (ICCVW)(2017)
摘要
Deep learning approaches have made tremendous progress in the field of semantic segmentation over the past few years. However, most current approaches operate in the 2D image space. Direct semantic segmentation of unstructured 3D point clouds is still an open research problem. The recently proposed PointNet architecture presents an interesting step ahead in that it can operate on unstructured point clouds, achieving encouraging segmentation results. However, it subdivides the input points into a grid of blocks and processes each such block individually. In this paper, we investigate the question how such an architecture can be extended to incorporate larger-scale spatial context. We build upon PointNet and propose two extensions that enlarge the receptive field over the 3D scene. We evaluate the proposed strategies on challenging indoor and outdoor datasets and show improved results in both scenarios.
更多查看译文
关键词
input points,blocks,larger-scale spatial context,receptive field,2D image space,direct semantic segmentation,open research problem,unstructured point clouds,deep learning approaches,PointNet architecture
AI 理解论文
溯源树
样例
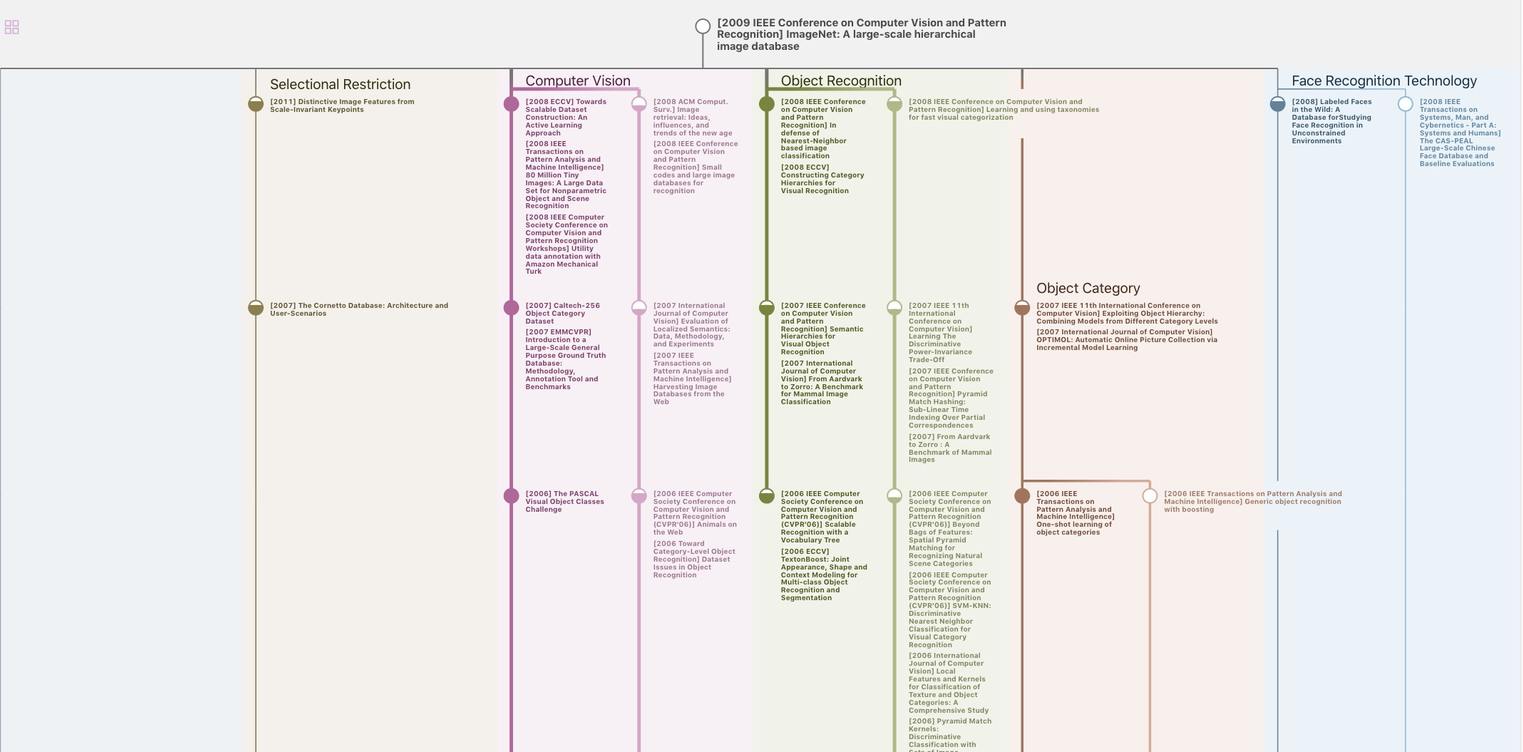
生成溯源树,研究论文发展脉络
Chat Paper
正在生成论文摘要