Hidden Markov Model Variational Autoencoder For Acoustic Unit Discovery
18TH ANNUAL CONFERENCE OF THE INTERNATIONAL SPEECH COMMUNICATION ASSOCIATION (INTERSPEECH 2017), VOLS 1-6: SITUATED INTERACTION(2017)
摘要
Variational Autoencoders (VAEs) have been shown to provide efficient neural-network-based approximate Bayesian inference for observation models for which exact inference is intractable. Its extension. the so-called Structured VAE (SVAE) allows inference in the presence of both discrete and continuous latent variables. Inspired by this extension, we developed a VAE with Hidden Markov Models (HMMs) as latent models. We applied the resulting HMM-VAE to the task of acoustic unit discovery in a zero resource scenario. Starting from an initial model based on variational inference in an HMM with Gaussian Mixture Model (GMM) emission probabilities, the accuracy of the acoustic unit discovery could be significantly improved by the HMM-VAE. In doing so we were able to demonstrate for an unsupervised learning task what is well-known in the supervised learning case: Neural networks provide superior modeling power compared to GMMs.
更多查看译文
关键词
variational autoencoder, hidden Markov Model, unsupervised learning, acoustic unit discovery
AI 理解论文
溯源树
样例
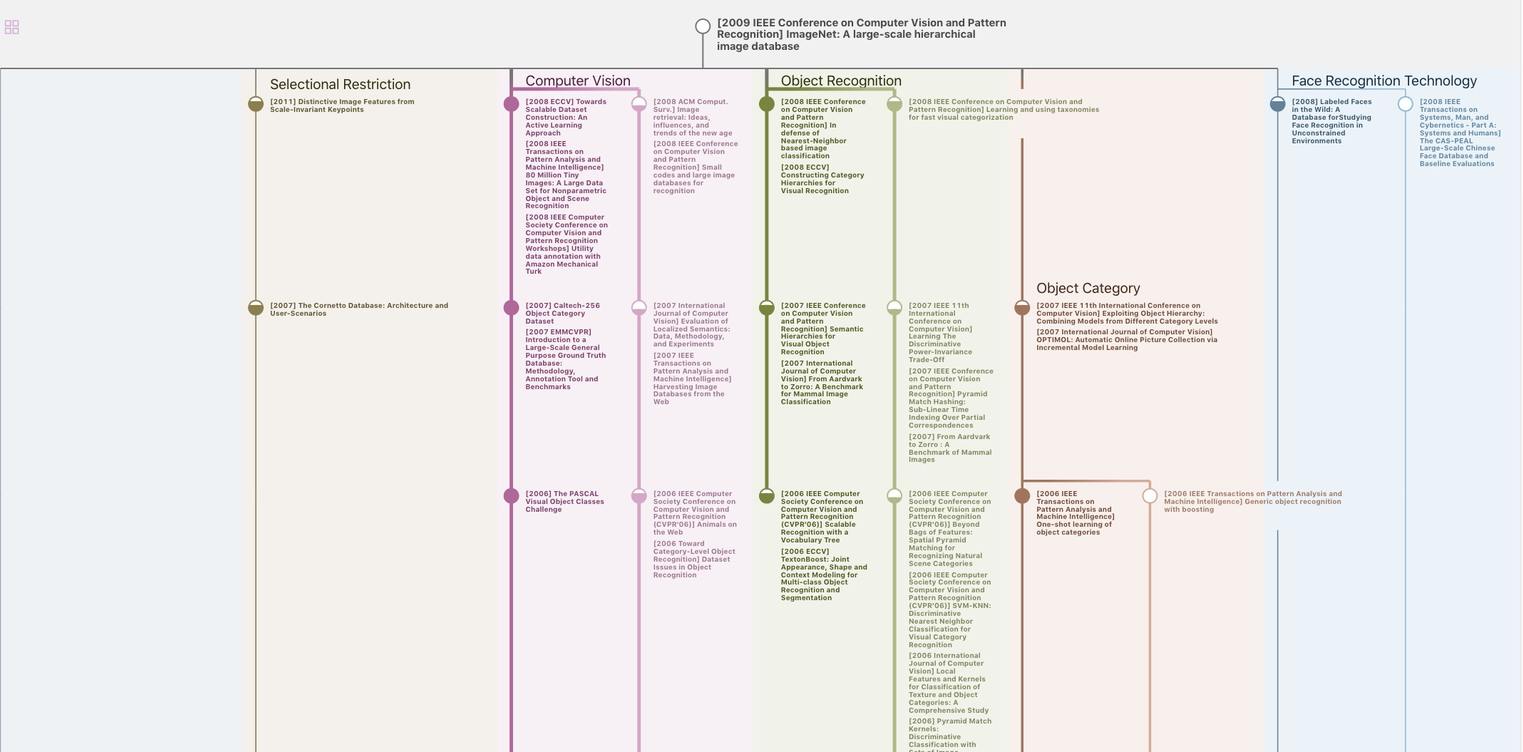
生成溯源树,研究论文发展脉络
Chat Paper
正在生成论文摘要