Efficient Emotion Recognition From Speech Using Deep Learning On Spectrograms
18TH ANNUAL CONFERENCE OF THE INTERNATIONAL SPEECH COMMUNICATION ASSOCIATION (INTERSPEECH 2017), VOLS 1-6: SITUATED INTERACTION(2017)
摘要
We present a new implementation of emotion recognition from the para-lingual information in the speech, based on a deep neural network, applied directly to spectrograms. This new method achieves higher recognition accuracy compared to previously published results, while also limiting the latency. It processes the speech input in smaller segments - up to 3 seconds, and splits a longer input into non-overlapping parts to reduce the prediction latency.The deep network comprises common neural network tools - convolutional and recurrent networks - which arc shown to effectively learn the information that represents emotions directly from spectrograms. Convolution-only lower complexity deep network achieves a prediction accuracy of 66% over four emotions (tested on IEMOCAP - a common evaluation corpus), while a combined convolution-LSTM higher-complexity model achieves 68%.The use of spectrograms in the role of speech-representing features enables effective handling of background non-speech signals such as music (excl. singing) and crowd noise, even at noise levels comparable with the speech signal levels. Using harmonic modeling to remove non-speech components from the spectrogram, we demonstrate significant improvement of the emotion recognition accuracy in the presence of unknown background non-speech signals.
更多查看译文
关键词
Speech Emotion Recognition, Para-lingual, Deep Neural Network, Spectrogram
AI 理解论文
溯源树
样例
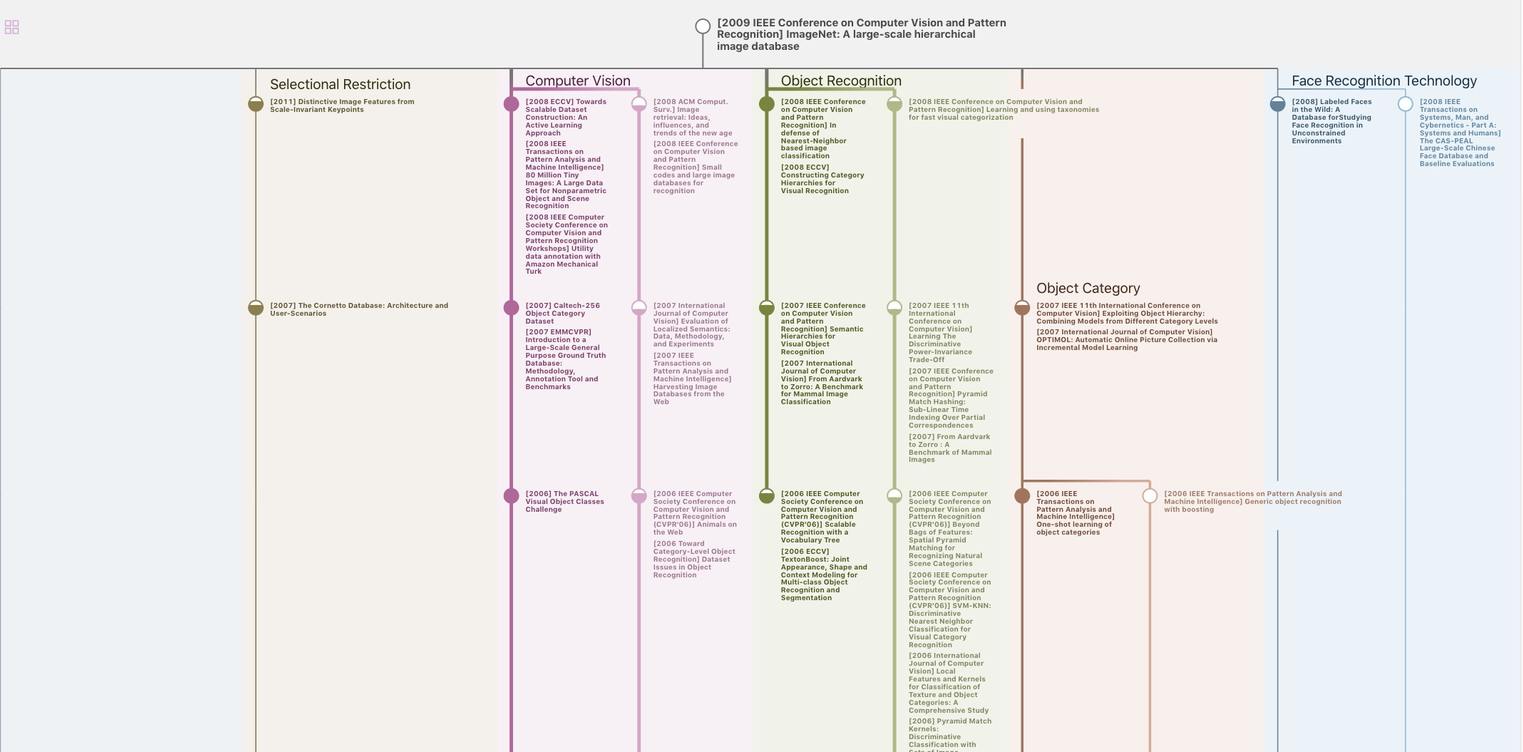
生成溯源树,研究论文发展脉络
Chat Paper
正在生成论文摘要