Domain Mismatch Modeling Of Out-Domain I-Vectors For Plda Speaker Verification
18TH ANNUAL CONFERENCE OF THE INTERNATIONAL SPEECH COMMUNICATION ASSOCIATION (INTERSPEECH 2017), VOLS 1-6: SITUATED INTERACTION(2017)
摘要
The state-of-the-art i-vector based probabilistic linear discriminant analysis (PLDA) trained on non-target (or out-domain) data significantly affects the speaker verification performance due to the domain mismatch between training and evaluation data. To improve the speaker verification performance, sufficient amount of domain mismatch compensated out-domain data must be used to train the PLDA models successfully. In this paper, we propose a domain mismatch modeling (DMM) technique using maximum-a-posteriori (MAP) estimation to model and compensate the domain variability from the out-domain training i-vectors. From our experimental results. we found that the DMM technique can achieve at least a 24% improvement in EER over an out-domain only baseline when speaker labels are available. Further improvement of 3% is obtained when combining DMM with domain-invariant covariance normalization (DICN) approach. The DMM/DICN combined technique is shown to perform better than in-domain PLDA system with only 200 labeled speakers or 2,000 unlabeled i-vectors.
更多查看译文
关键词
Speaker verification, domain adaptation, GPLDA, DMM, DICN
AI 理解论文
溯源树
样例
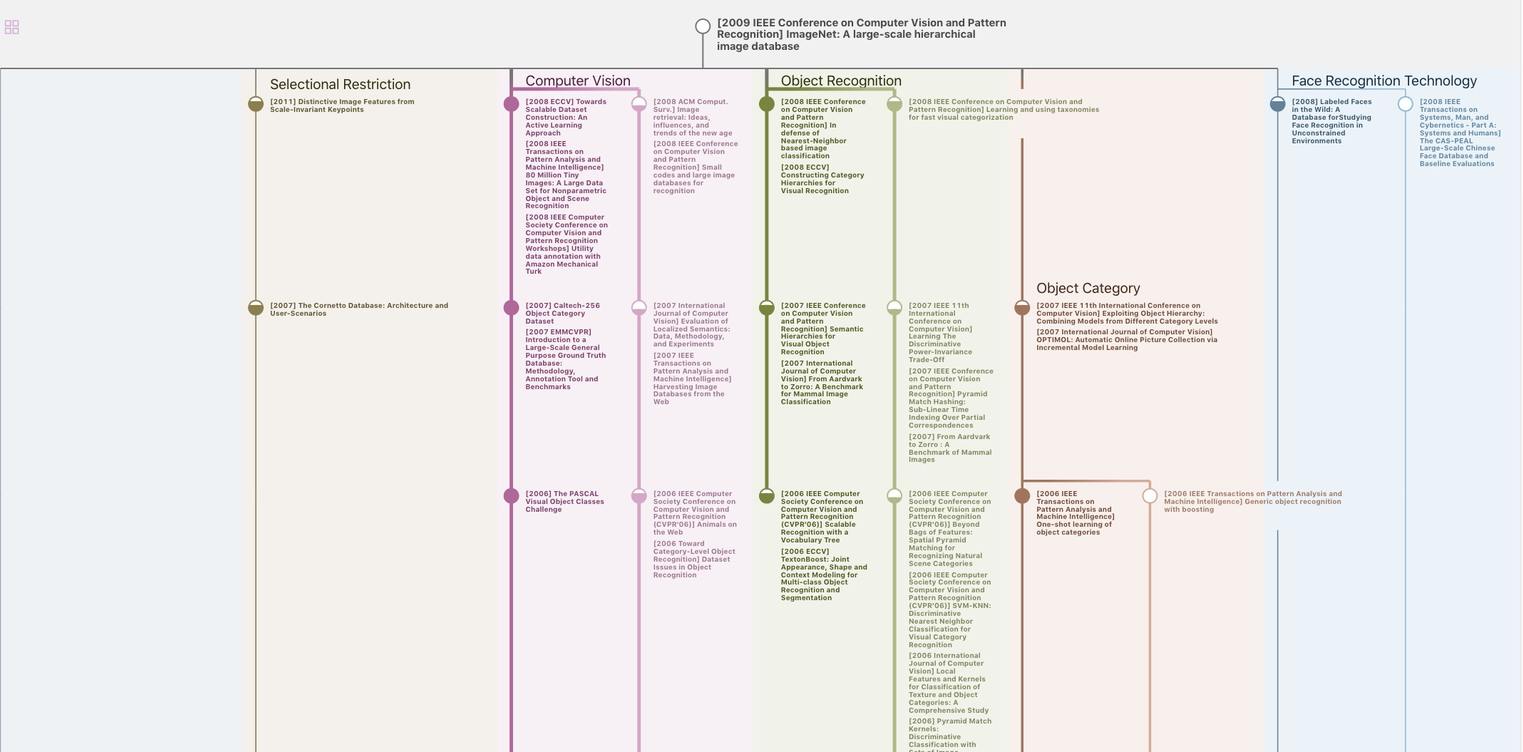
生成溯源树,研究论文发展脉络
Chat Paper
正在生成论文摘要