Classification of calcium in intravascular OCT images for the purpose of intervention planning.
Proceedings of SPIE(2016)
摘要
The presence of extensive calcification is a primary concern when planning and implementing a vascular percutaneous intervention such as stenting. If the balloon does not expand, the interventionalist must blindly apply high balloon pressure, use an atherectomy device, or abort the procedure. As part of a project to determine the ability of Intravascular Optical Coherence Tomography (IVOCT) to aid intervention planning, we developed a method for automatic classification of calcium in coronary IVOCT images. We developed an approach where plaque texture is modeled by the joint probability distribution of a bank of filter responses where the filter bank was chosen to reflect the qualitative characteristics of the calcium. This distribution is represented by the frequency histogram of filter response cluster centers. The trained algorithm was evaluated on independent ex-vivo image data accurately labeled using registered 3D microscopic cryo-image data which was used as ground truth. In this study, regions for extraction of sub-images (SI's) were selected by experts to include calcium, fibrous, or lipid tissues. We manually optimized algorithm parameters such as choice of filter bank, size of the dictionary, etc. Splitting samples into training and testing data, we achieved 5-fold cross validation calcium classification with F-1 score of 93.7 +/- 2.7% with recall of >= 89% and a precision of >= 97% in this scenario with admittedly selective data. The automated algorithm performed in close-to-real-time (2.6 seconds per frame) suggesting possible on-line use. This promising preliminary study indicates that computational IVOCT might automatically identify calcium in IVOCT coronary artery images.
更多查看译文
关键词
automated classification,OCT,IVOCT,intravascular,calcium,machine learning,plaque
AI 理解论文
溯源树
样例
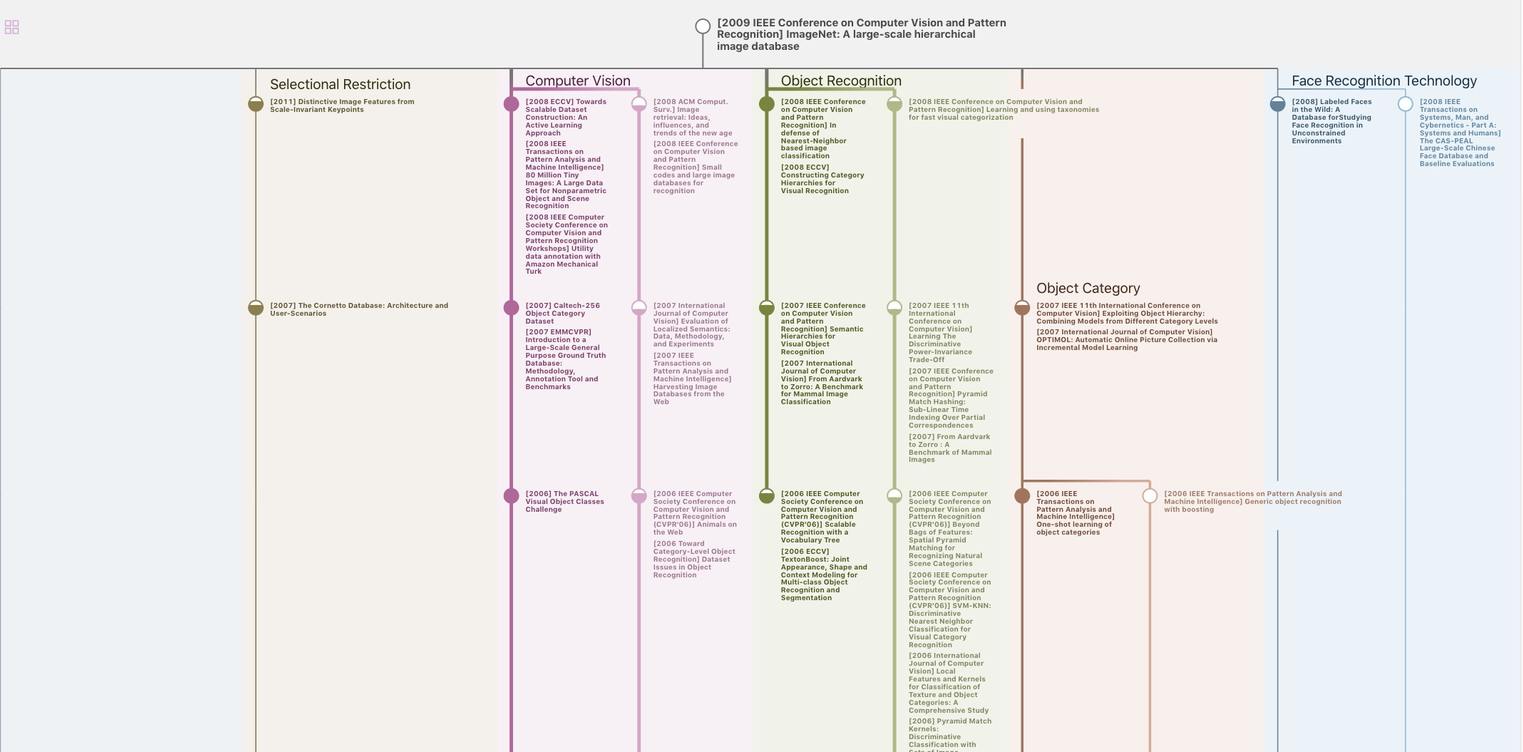
生成溯源树,研究论文发展脉络
Chat Paper
正在生成论文摘要