Organ detection in thorax abdomen CT using multi-label convolutional neural networks.
Proceedings of SPIE(2017)
摘要
A convolutional network architecture is presented to determine bounding boxes around six organs in thorax-abdomen CT scans. A single network for each orthogonal view determines the presence of lungs, kidneys, spleen and liver. We show that an architecture that takes additional slices before and after the slice of interest as an additional input outperforms an architecture that processes single slices. From the slice-based analysis, a bounding box around the structures of interest can be computed. The system uses 6 convolutional, 4 pooling and one fully connected layer and uses 333 scans for training and 110 for validation. The test set contains 110 scans. The average Dice score of the proposed method was 0.95 and 0.95 for the lungs, 0.59 and 0.58 for the kidneys, 0.83 for the liver and 0.63 for the spleen. This paper shows that automatic localization of organs using multi-label convolution neural networks is possible. This architecture can likely be used to identify other organs of interest as well.
更多查看译文
关键词
Deep learning,convolutional neural networks,multi-label,computed tomography
AI 理解论文
溯源树
样例
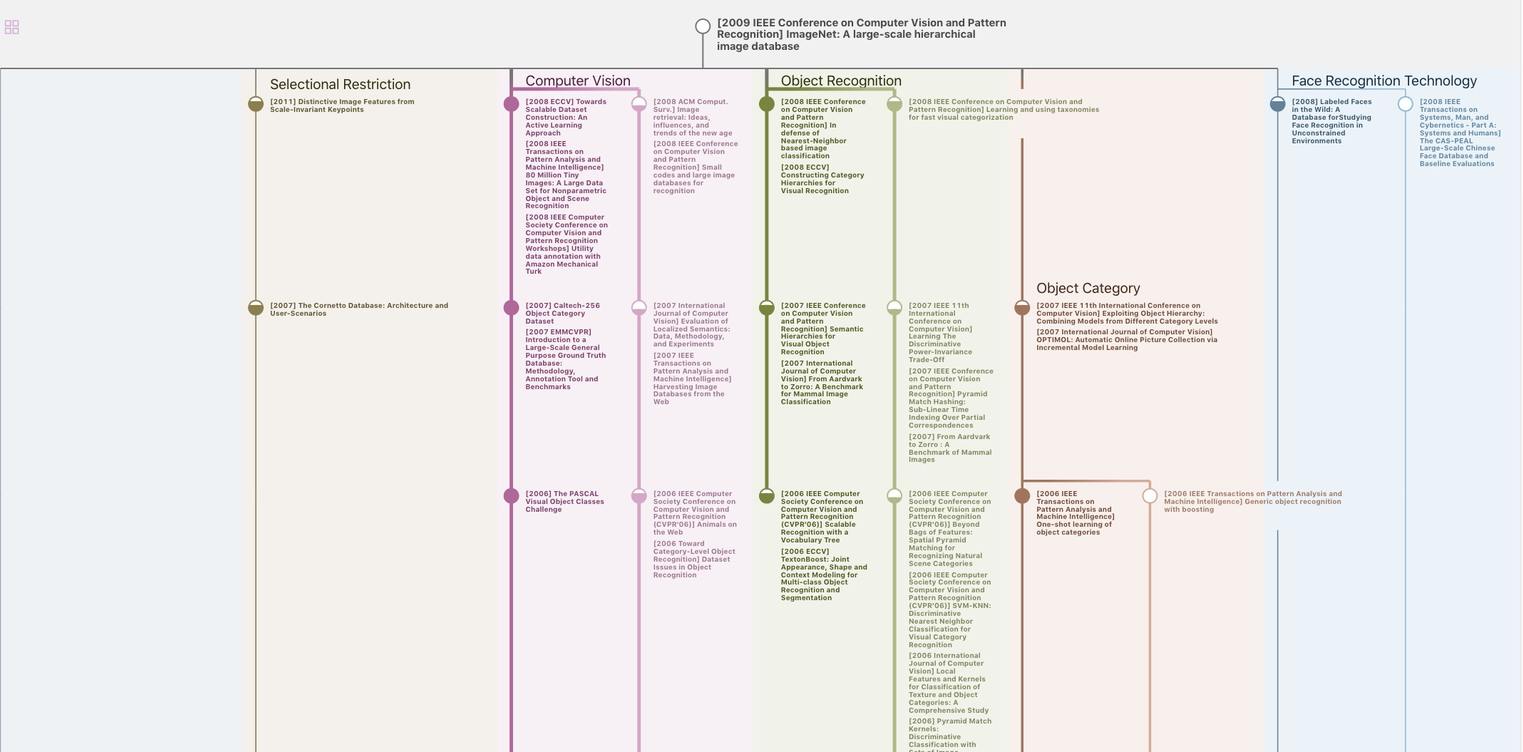
生成溯源树,研究论文发展脉络
Chat Paper
正在生成论文摘要