A transfer learning approach for classification of clinical significant prostate cancers from mpMRI scans.
Proceedings of SPIE(2017)
摘要
Deep learning has shown a great potential in computer aided diagnosis. However, in many applications, large dataset is not available. This makes the training of a sophisticated deep learning neural network (DNN) difficult. In this study, we demonstrated that with transfer learning, we can quickly retrain start-of-the-art DNN models with limited data provided by the prostateX challenge. The training data consists of 330 lesions, only 78 were clinical significant. Efforts were made to balance the data during training. We used ImageNet pre-trained inceptionV3 and Vgg-16 model and obtained AUC of 0.81 and 0.83 respectively on the prostateX test data, good for a 4th place finish. We noticed that models trained for different prostate zone has different sensitivity. Applying scaling factors before merging the result improves the AUC for the final result.
更多查看译文
关键词
prostate cancer,deep learning neural network (DNN),Convolution neural Network (CNN),multiparameter MRI (mpMRI),transfer learning,computer aided diagnosis (CADx),prostateX challenge,VGG
AI 理解论文
溯源树
样例
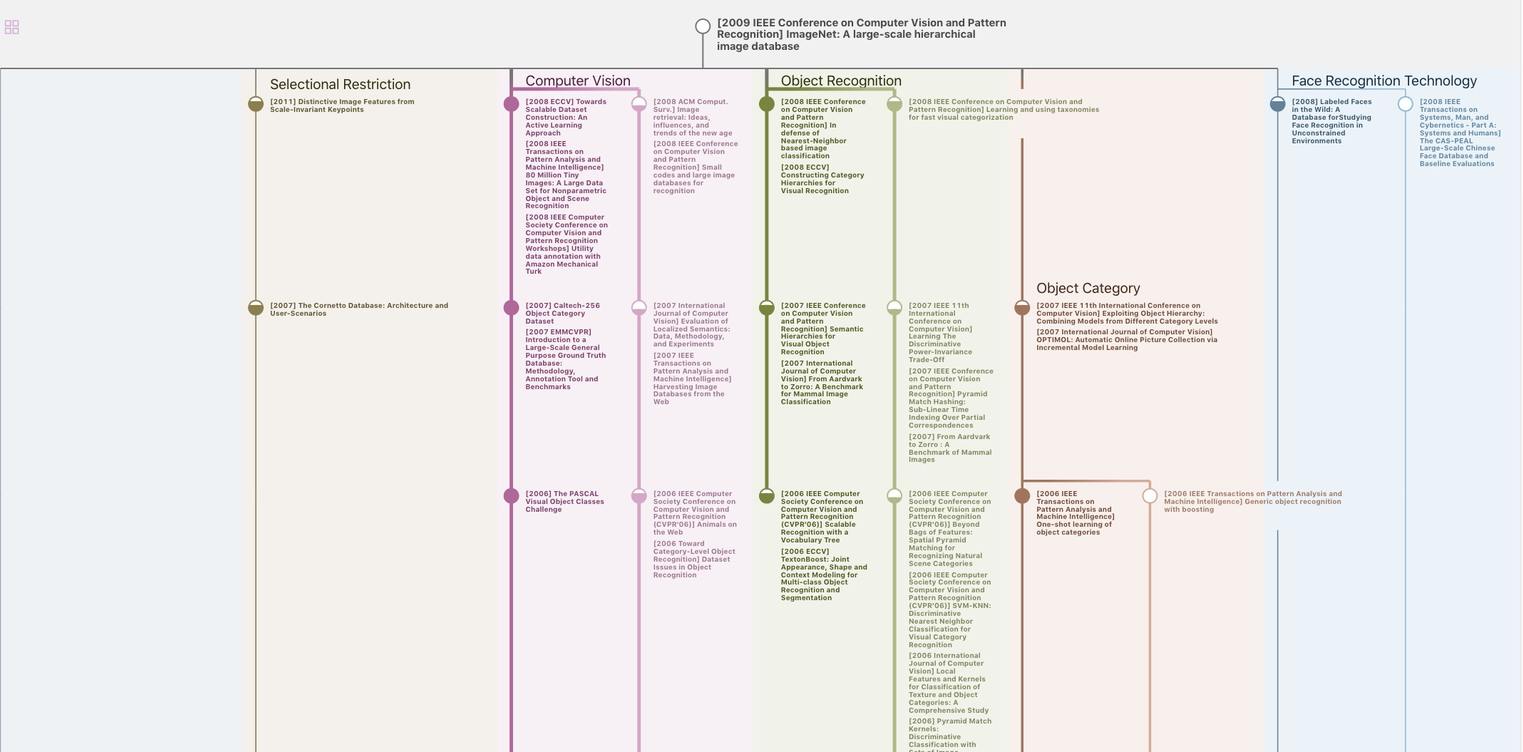
生成溯源树,研究论文发展脉络
Chat Paper
正在生成论文摘要