A Stochastic Multi-Armed Bandit Approach To Nonparametric H-Infinity-Norm Estimation
2017 IEEE 56TH ANNUAL CONFERENCE ON DECISION AND CONTROL (CDC)(2017)
摘要
We study the problem of estimating the largest gain of an unknown linear and time-invariant filter, which is also known as the H-infinity norm of the system. By using ideas from the stochastic multi-armed bandit framework, we present a new algorithm that sequentially designs an input signal in order to estimate this quantity by means of input-output data. The algorithm is shown empirically to beat an asymptotically optimal method, known as Thompson Sampling, in the sense of its cumulative regret function. Finally, for a general class of algorithms, a lower bound on the performance of finding the H-infinity norm is derived.
更多查看译文
关键词
time-invariant filter,linear filter,stochastic multiarmed bandit,nonparametric H∞-norm estimation,Thompson Sampling,asymptotically optimal method
AI 理解论文
溯源树
样例
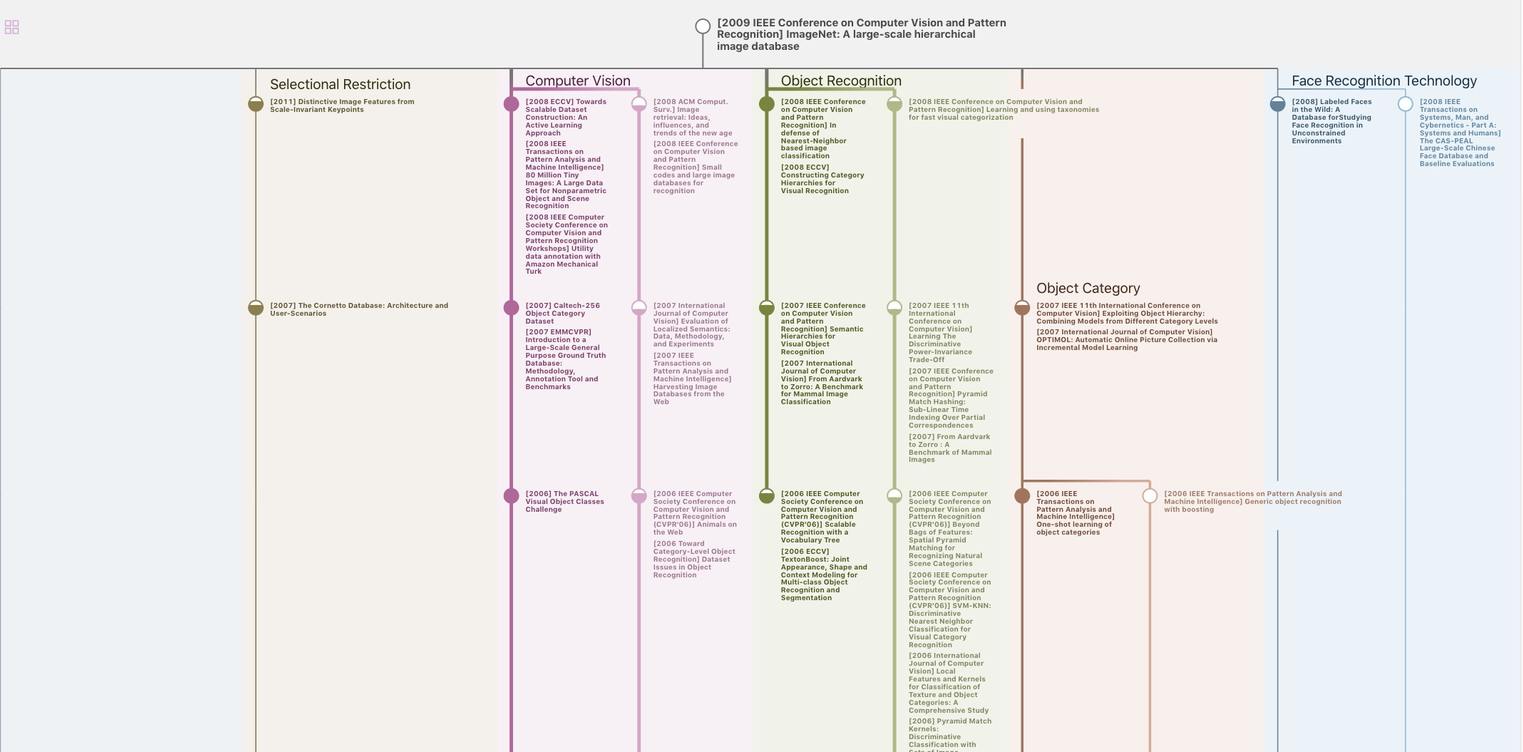
生成溯源树,研究论文发展脉络
Chat Paper
正在生成论文摘要