Deep Scene Image Classification With The Mfafvnet
2017 IEEE INTERNATIONAL CONFERENCE ON COMPUTER VISION (ICCV)(2017)
摘要
The problem of transferring a deep convolutional network trained for object recognition to the task of scene image classification is considered. An embedded implementation of the recently proposed mixture of factor analyzers Fisher vector (MFA-FV) is proposed. This enables the design of a network architecture, the MFAFVNet, that can be trained in an end to end manner. The new architecture involves the design of a MFA-FV layer that implements a statistically correct version of the MFA-FV, through a combination of network computations and regularization. When compared to previous neural implementations of Fisher vectors, the MFAFVNet relies on a more powerful statistical model and a more accurate implementation. When compared to previous non-embedded models, the MFAFVNet relies on a state of the art model, which is now embedded into a CNN. This enables end to end training. Experiments show that the MFAFVNet has state of the art performance on scene classification.
更多查看译文
关键词
factor analyzers,statistical model,Fisher vector,Fisher vectors,network computations,MFA-FV layer,network architecture,object recognition,deep convolutional network,deep scene image classification,MFAFVNet,end training
AI 理解论文
溯源树
样例
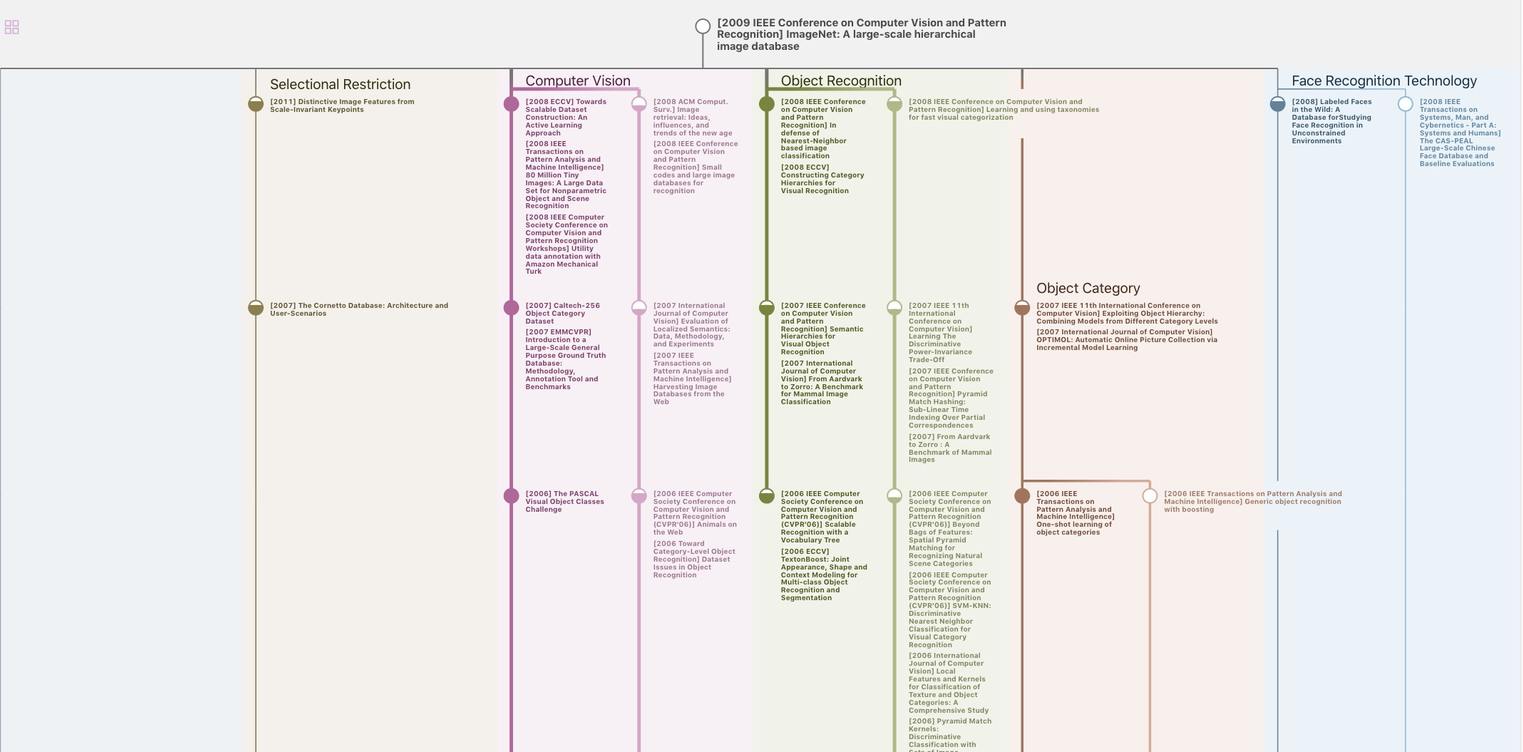
生成溯源树,研究论文发展脉络
Chat Paper
正在生成论文摘要