Exploiting Spatial Structure For Localizing Manipulated Image Regions
2017 IEEE INTERNATIONAL CONFERENCE ON COMPUTER VISION (ICCV)(2017)
摘要
The advent of high-tech journaling tools facilitates an image to be manipulated in a way that can easily evade state-of-the-art image tampering detection approaches. The recent success of the deep learning approaches in different recognition tasks inspires us to develop a high confidence detection framework which can localize manipulated regions in an image. Unlike semantic object segmentation where all meaningful regions (objects) are segmented, the localization of image manipulation focuses only the possible tampered region which makes the problem even more challenging. In order to formulate the framework, we employ a hybrid CNN-LSTM model to capture discriminative features between manipulated and non-manipulated regions. One of the key properties of manipulated regions is that they exhibit discriminative features in boundaries shared with neighboring non-manipulated pixels. Our motivation is to learn the boundary discrepancy, i.e., the spatial structure, between manipulated and non-manipulated regions with the combination of LSTM and convolution layers. We perform end-to-end training of the network to learn the parameters through back-propagation given ground-truth mask information. The overall framework is capable of detecting different types of image manipulations, including copy-move, removal and splicing. Our model shows promising results in localizing manipulated regions, which is demonstrated through rigorous experimentation on three diverse datasets.
更多查看译文
关键词
spatial structure,high-tech journaling tools,high confidence detection framework,semantic object segmentation,possible tampered region,hybrid CNN-LSTM model,discriminative features,nonmanipulated regions,recognition tasks,manipulated image region localization,image tampering detection approaches,meaningful region segmentation,image manipulation localization,neighboring nonmanipulated pixels,convolution layer,LSTM layer,back-propagation,removal,splicing,copy-move
AI 理解论文
溯源树
样例
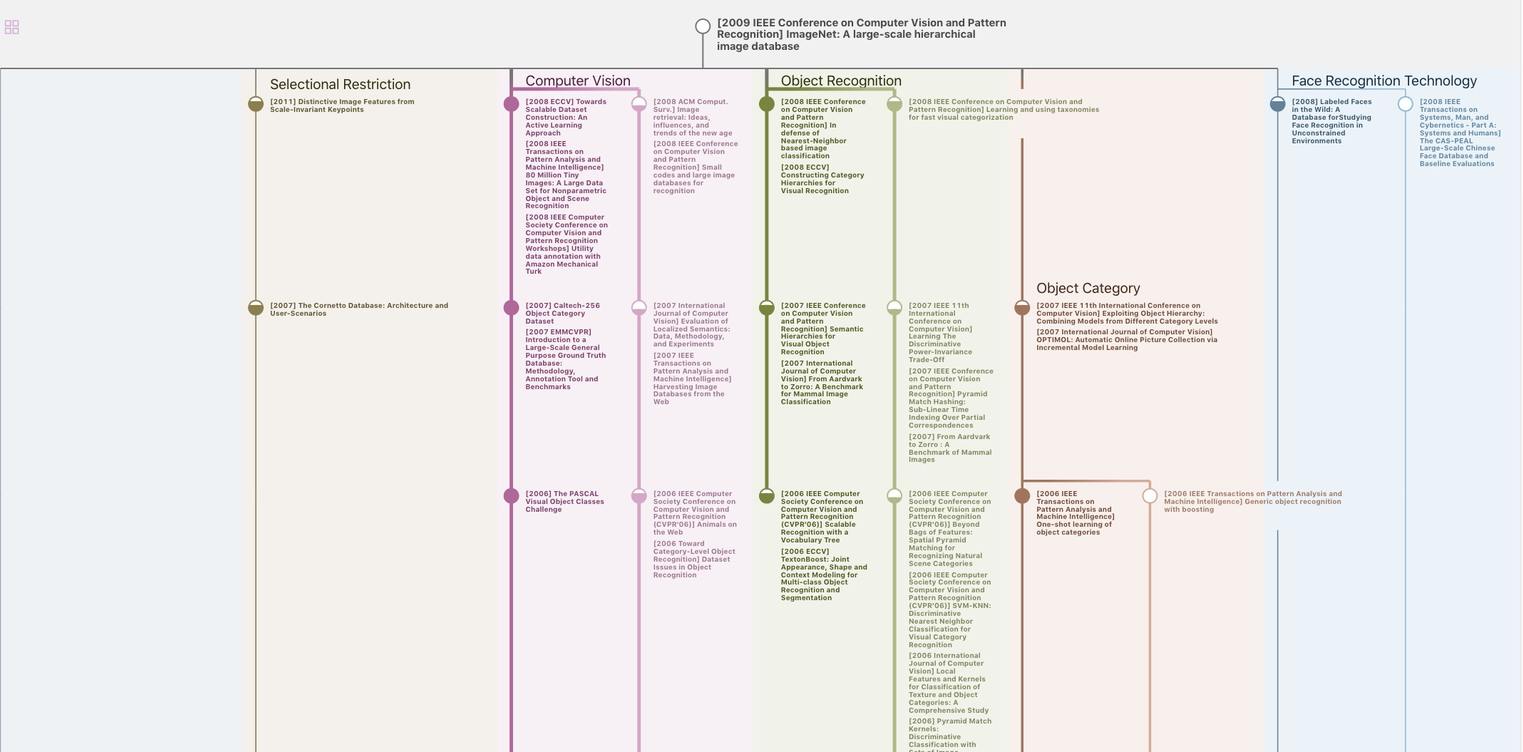
生成溯源树,研究论文发展脉络
Chat Paper
正在生成论文摘要