Simulation study in quantifying heterogeneous causal effects
WSC '17: Winter Simulation Conference Las Vegas Nevada December, 2017(2017)
摘要
Quantifying the impact of an intervention or treatment in a real setting is a common and challenging problem. For example, we would like to calculate the environmental implications of aid projects in third world countries that target economic development. For causal inference problems of this kind, the Rubin causal model is one of several popular theoretical frameworks that comes with a set of algorithmic methods to quantify treatment effects. However, for a given data set, we neither know the ground truth nor can we easily increase the size of the data set. So, simulation is a natural choice to evaluate the applicability of a set of methods for a particular problem. In this paper, we report findings of a simulation study with four causal inference approaches, namely two single tree approaches (transformed outcome tree, causal tree), and two random forest versions of the former.
更多查看译文
关键词
causal inference problems,Rubin causal model,algorithmic methods,treatment effects,causal inference approaches,single tree approaches,causal tree,heterogeneous causal effects
AI 理解论文
溯源树
样例
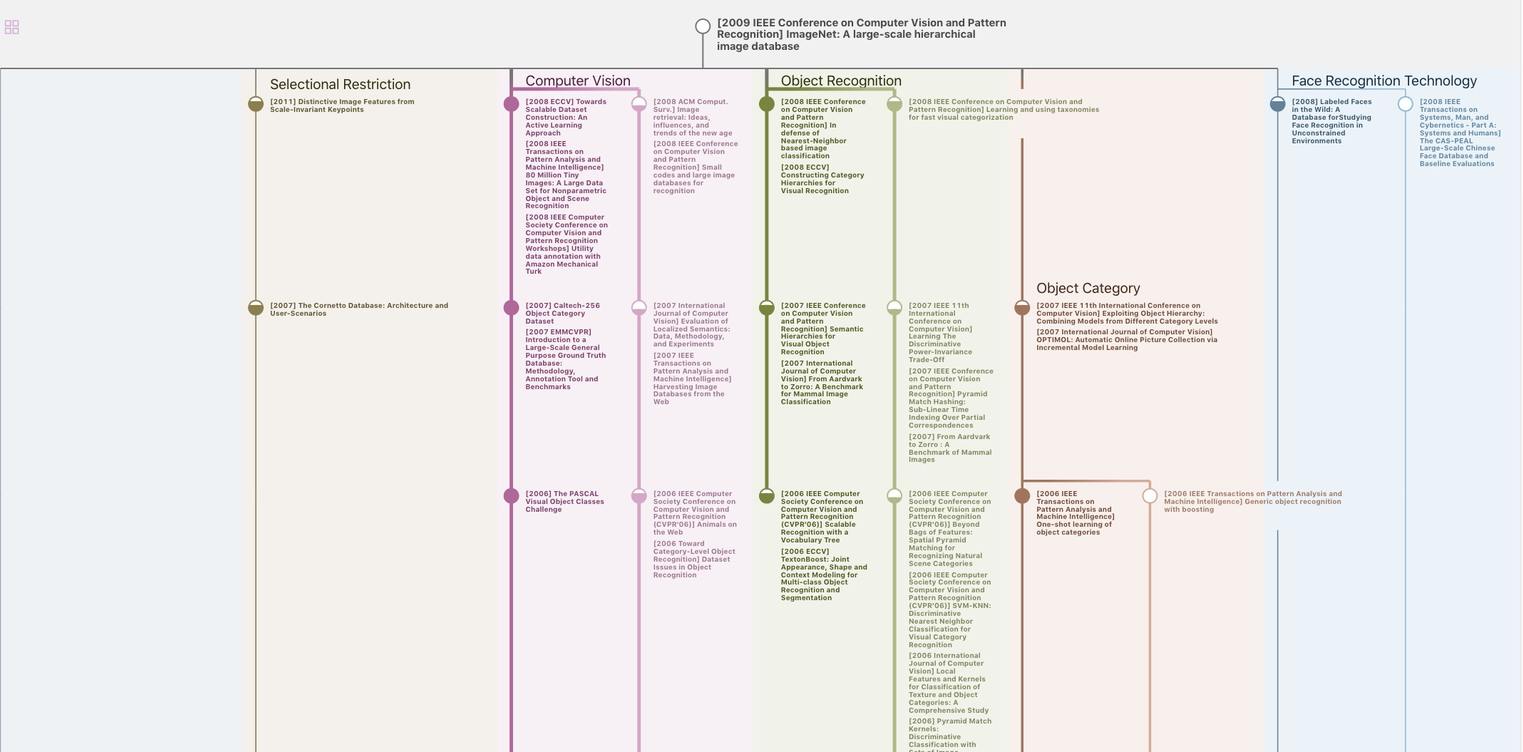
生成溯源树,研究论文发展脉络
Chat Paper
正在生成论文摘要