Deep gaussian process metamodeling of sequentially sampled non-stationary response surfaces
WSC '17: Winter Simulation Conference Las Vegas Nevada December, 2017(2017)
摘要
Simulations are often used for the design of complex systems as they allow to explore the design space without the need to build several prototypes. Over the years, the simulation accuracy, as well as the associated computational cost has increased significantly, limiting the overall number of simulations during the design process. Therefore, metamodeling aims to approximate the simulation response with a cheap-to-evaluate mathematical approximation, learned from a limited set of simulator evaluations. Kernel-based methods using stationary kernels are nowadays wildly used. However, using stationary kernels for non-stationary responses can be inappropriate and result in poor models when combined with sequential design. We present the application of a novel kernel-based technique, known as Deep Gaussian Processes, which is better able to cope with these difficulties. We evaluate the method for non-stationary regression on a series of real-world problems, showing that it outperforms the standard Gaussian Processes with stationary kernels.
更多查看译文
关键词
sequentially sampled non-stationary response surfaces,Simulations,mathematical approximation,complex systems,Deep Gaussian process metamodeling,nonstationary regression,stationary kernels
AI 理解论文
溯源树
样例
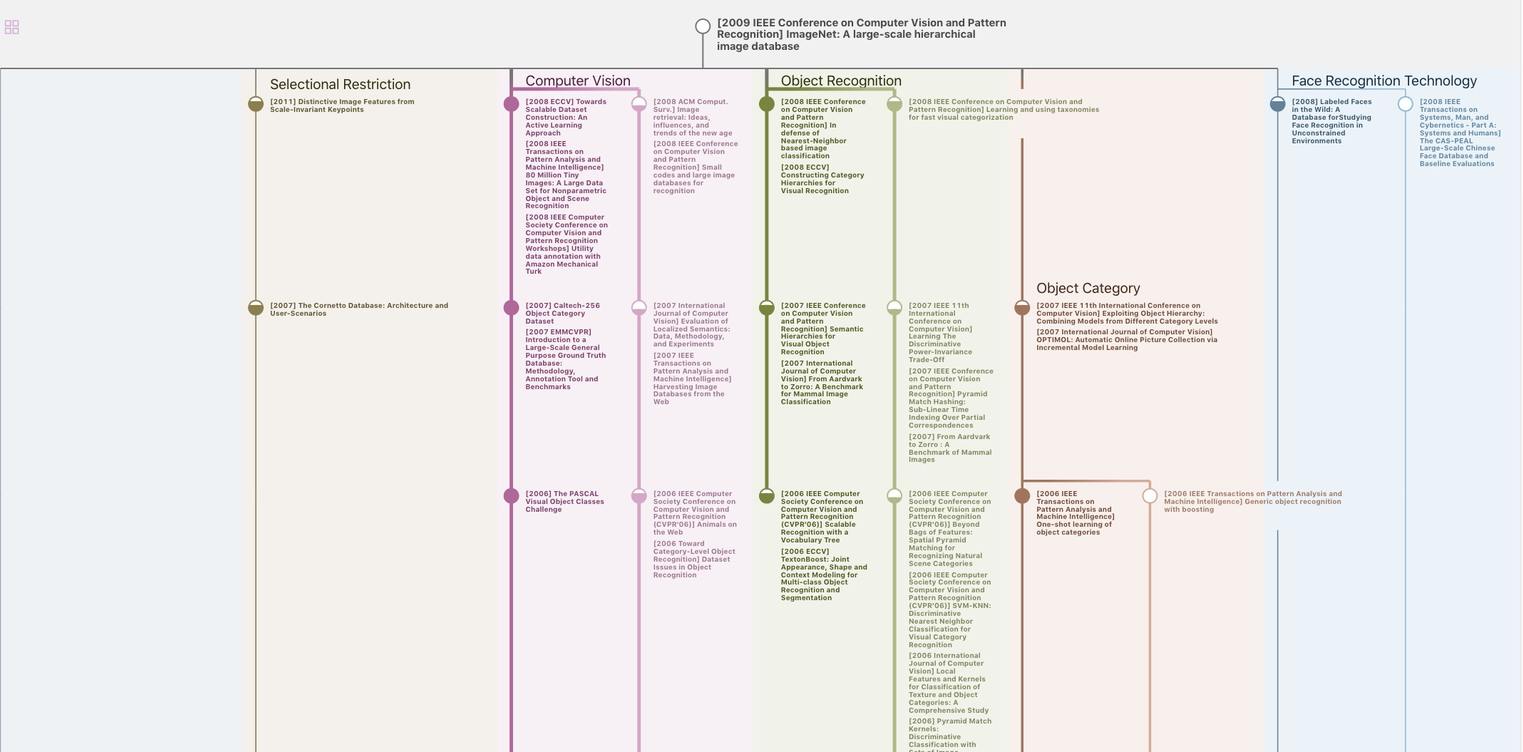
生成溯源树,研究论文发展脉络
Chat Paper
正在生成论文摘要