Extended Methods to Handle Classification Biases
2017 IEEE International Conference on Data Science and Advanced Analytics (DSAA)(2017)
摘要
Classifiers can provide counts of items per class, but systematic classification errors yield biases (e.g., if a class is often misclassified as another, its size may be under-estimated). To handle classification biases, the statistics and epidemiology domains devised methods for estimating unbiased class sizes (or class probabilities) without identifying which individual items are misclassified. These bias correction methods are applicable to machine learning classifiers, but in some cases yield high result variance and increased biases. We present the applicability and drawbacks of existing methods and extend them with three novel methods. Our Sample-to-Sample method provides accurate confidence intervals for the bias correction results. Our Maximum Determinant method predicts which classifier yields the least result variance. Our Ratio-to-TP method details the error decomposition in classifier outputs (i.e., how many items classified as class C_y truly belong to C_x, for all possible classes) and has properties of interest for applying the Maximum Determinant method. Our methods are demonstrated empirically, and we discuss the need for establishing theory and guidelines for choosing the methods and classifier to apply.
更多查看译文
关键词
Classification Error and Bias,Bias Correction,Variance Estimation,Error Composition
AI 理解论文
溯源树
样例
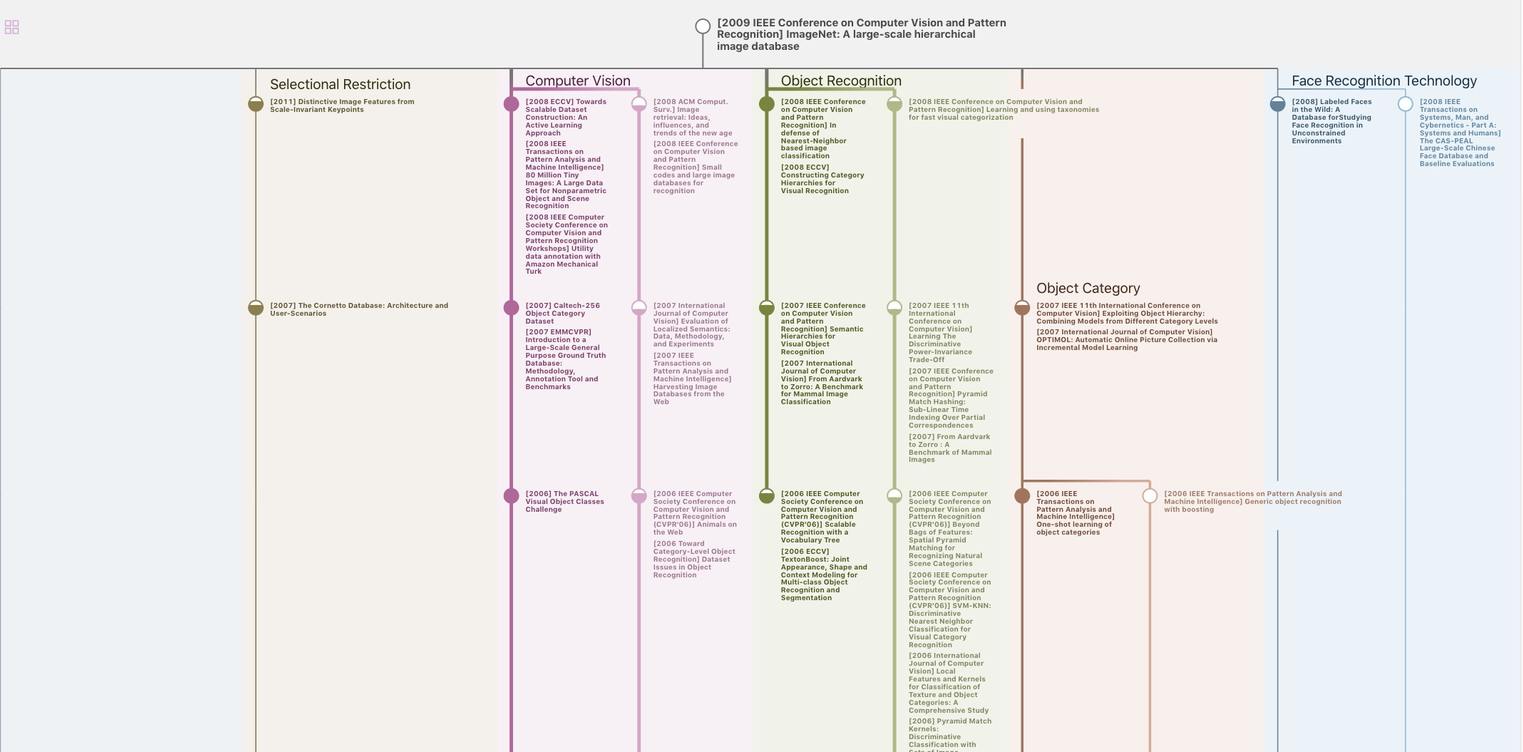
生成溯源树,研究论文发展脉络
Chat Paper
正在生成论文摘要