Kernel based online learning for imbalance multiclass classification.
Neurocomputing(2018)
摘要
In this paper, we propose a weighted online sequential extreme learning machine with kernels (WOS-ELMK) for class imbalance learning (CIL). The existing online sequential extreme learning machine (OS-ELM) methods for CIL use random feature mapping. WOS-ELMK is the first OS-ELM method which uses kernel mapping for online class imbalance learning. The kernel mapping avoids the non-optimal hidden node problem associated with weighted OS-ELM (WOS-ELM) and other existing OS-ELM methods for CIL. WOS-ELMK tackles both the binary class and multiclass imbalance problems in one-by-one as well as chunk-by-chunk learning modes. For imbalanced big data streams, a fixed size window scheme is also implemented for WOS-ELMK. We empirically show that WOS-ELMK obtains superior performance in general than some recently proposed CIL approaches on 17 binary class and 8 multiclass imbalanced datasets.
更多查看译文
关键词
Class imbalance,Extreme learning machine (ELM),Kernel learning,Multiclass,Online learning
AI 理解论文
溯源树
样例
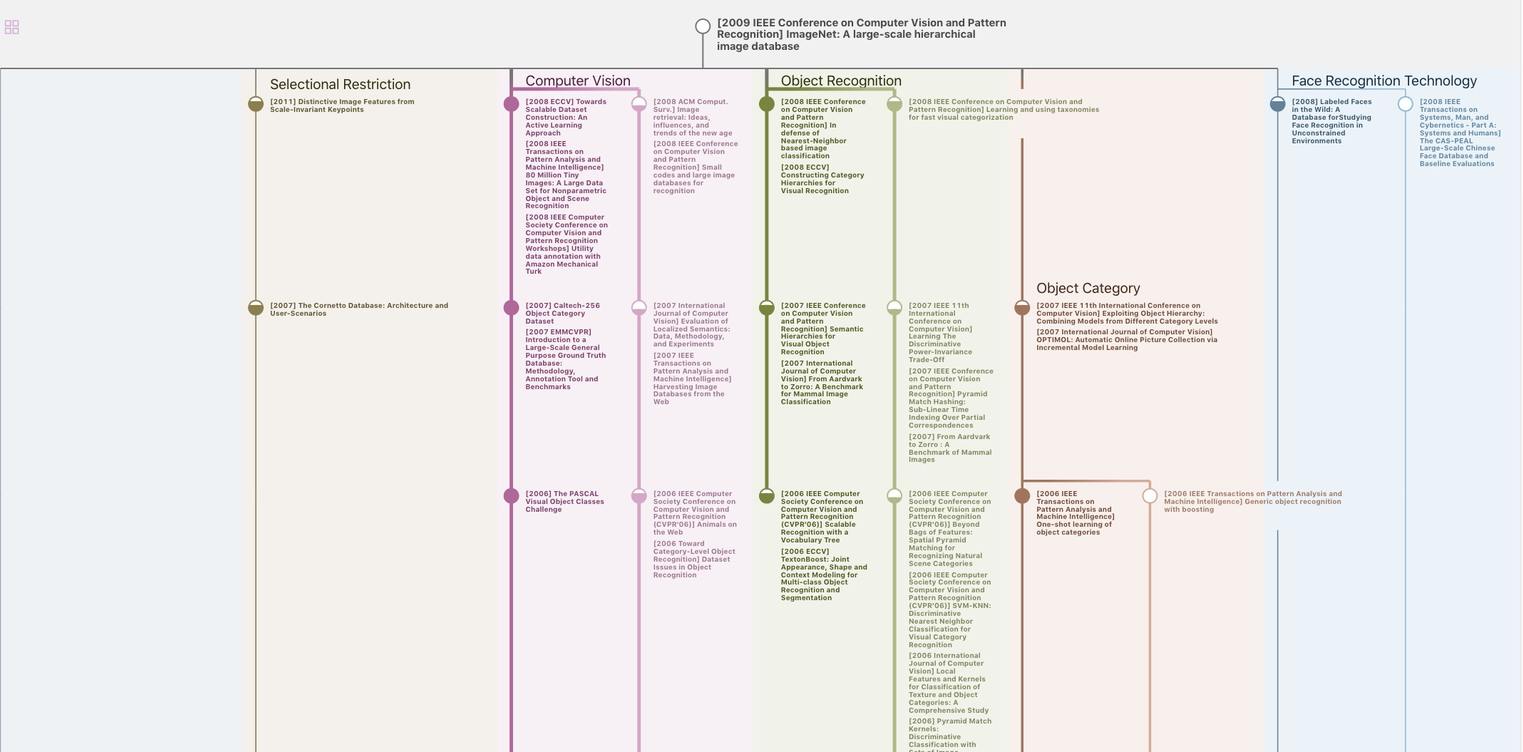
生成溯源树,研究论文发展脉络
Chat Paper
正在生成论文摘要