MODIS-Based Estimation of Terrestrial Latent Heat Flux over North America Using Three Machine Learning Algorithms.
REMOTE SENSING(2017)
摘要
Terrestrial latent heat flux (LE) is a key component of the global terrestrial water, energy, and carbon exchanges. Accurate estimation of LE from moderate resolution imaging spectroradiometer (MODIS) data remains a major challenge. In this study, we estimated the daily LE for different plant functional types (PFTs) across North America using three machine learning algorithms: artificial neural network (ANN); support vector machines (SVM); and, multivariate adaptive regression spline (MARS) driven by MODIS and Modern Era Retrospective Analysis for Research and Applications (MERRA) meteorology data. These three predictive algorithms, which were trained and validated using observed LE over the period 2000-2007, all proved to be accurate. However, ANN outperformed the other two algorithms for the majority of the tested configurations for most PFTs and was the only method that arrived at 80% precision for LE estimation. We also applied three machine learning algorithms for MODIS data and MERRA meteorology to map the average annual terrestrial LE of North America during 2002-2004 using a spatial resolution of 0.05 degrees, which proved to be useful for estimating the long-term LE over North America.
更多查看译文
关键词
latent heat,machine learning algorithms,plant functional type
AI 理解论文
溯源树
样例
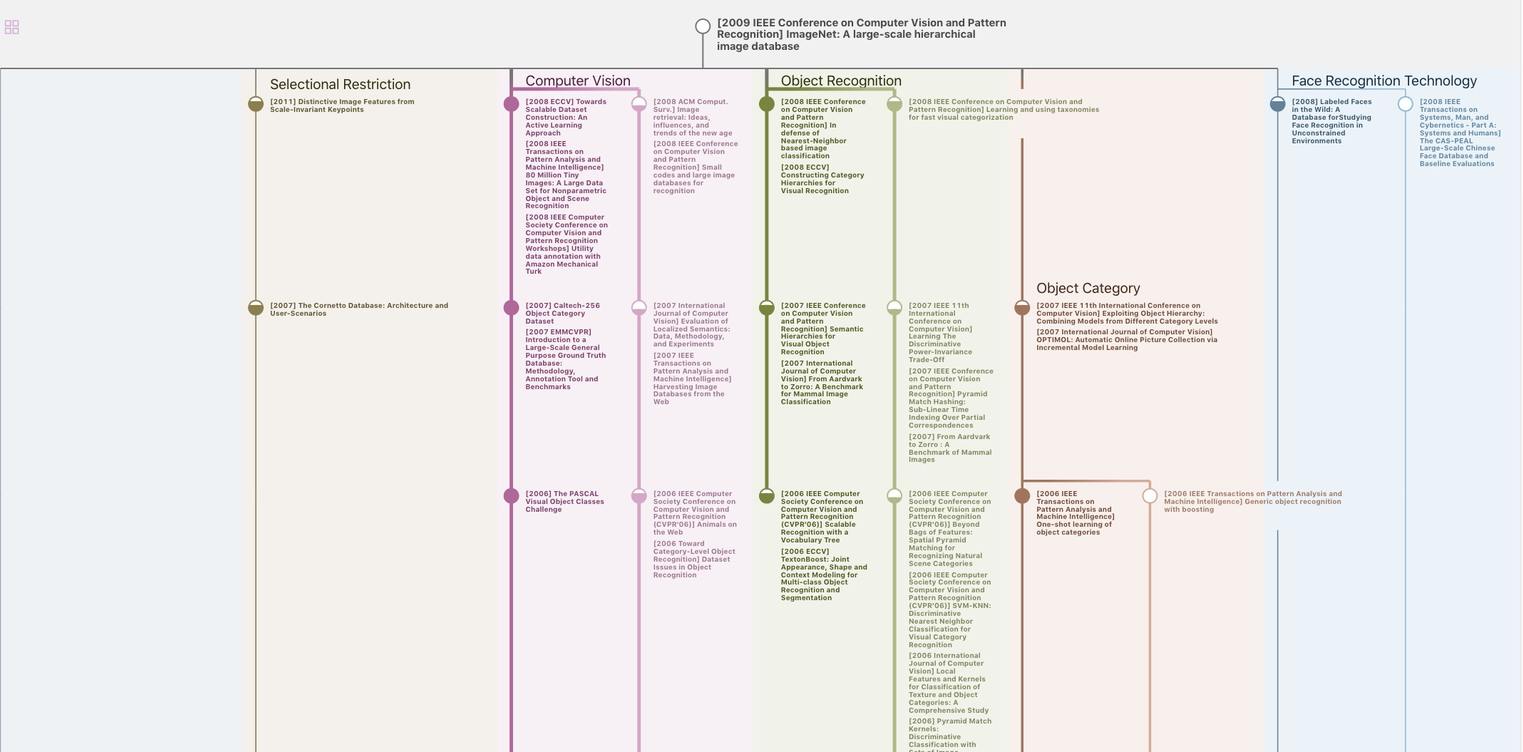
生成溯源树,研究论文发展脉络
Chat Paper
正在生成论文摘要