Optimizing the efficiency of deep learning through accelerator virtualization.
IBM Journal of Research and Development(2017)
摘要
Training deep learning models often occupies entire compute clusters, built solely for this purpose, for days or even weeks at a time. There exists a large body of work on approaches for improving training performance, ranging from novel algorithms to full custom hardware accelerators. Offering compute capabilities of multiple teraflops (trillion floating point operations per second), graphics pro...
更多查看译文
关键词
Training,Graphics processing units,Acceleration,Speech recognition,Machine learning,Virtualization,Hardware
AI 理解论文
溯源树
样例
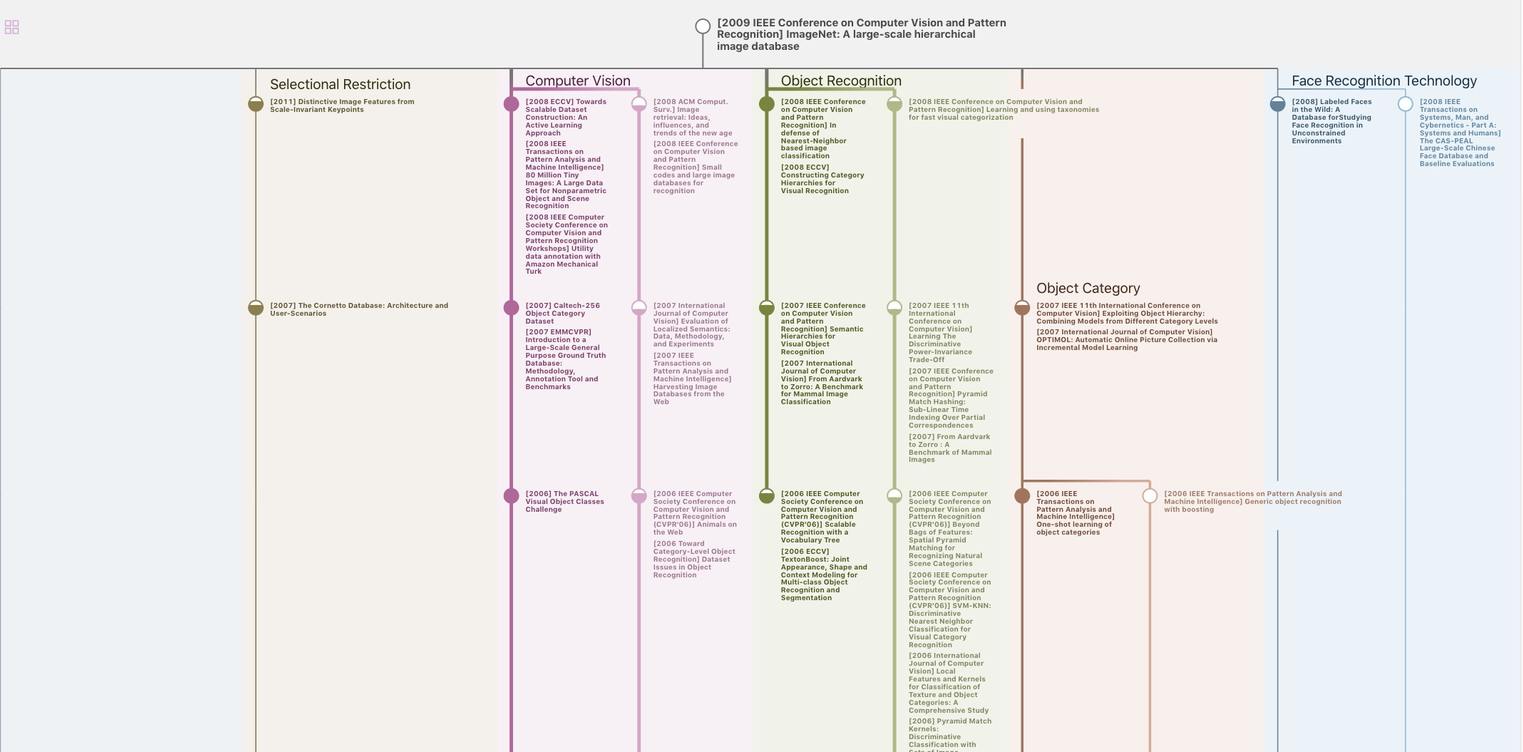
生成溯源树,研究论文发展脉络
Chat Paper
正在生成论文摘要