A multi-label cellular automata model for land change simulation.
Trans. GIS(2017)
摘要
The use of cellular automata (CA) has for some time been considered among the most appropriate approaches for modeling land-use changes. Each cell in a traditional CA model has a state that evolves according to transition rules, taking into consideration its own and its neighbors' states and characteristics. Here, we present a multi-label CA model in which a cell may simultaneously have more than one state. The model uses a multi-label learning methoda multi-label support vector machine, Rank-SVMto define the transition rules. The model was used with a multi-label land-use dataset for Luxembourg, built from vector-based land-use data using a method presented here. The proposed multi-label CA model showed promising performance in terms of its ability to capture and model the details and complexities of changes in land-use patterns. Applied to historical land use data, the proposed model estimated the land use change with an accuracy of 87.2% exact matching and 98.84% when including cells with a misclassification of a single label, which is comparably better than a classical multi-class model that achieved 83.6%. The multi-label cellular automata outperformed a model combining CA and artificial neural networks. All model goodness-of-fit comparisons were quantified using various performance metrics for predictive models.
更多查看译文
AI 理解论文
溯源树
样例
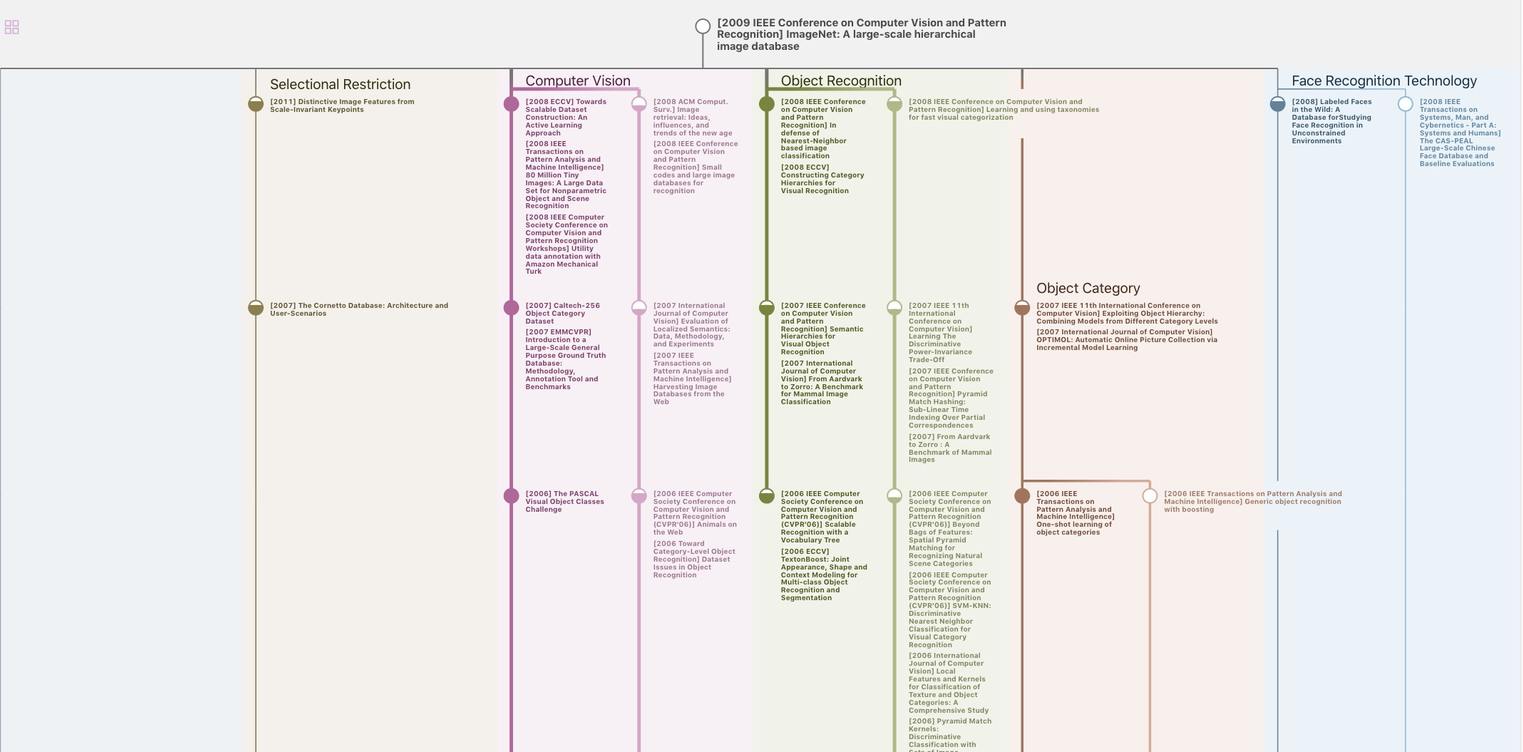
生成溯源树,研究论文发展脉络
Chat Paper
正在生成论文摘要