Grounding Referring Expressions in Images by Variational Context
2018 IEEE/CVF Conference on Computer Vision and Pattern Recognition(2018)
摘要
We focus on grounding (i.e., localizing or linking) referring expressions in images, e.g., "largest elephant standing behind baby elephant". This is a general yet challenging vision-language task since it does not only require the localization of objects, but also the multimodal comprehension of context --- visual attributes (e.g., "largest", "baby") and relationships (e.g., "behind") that help to distinguish the referent from other objects, especially those of the same category. Due to the exponential complexity involved in modeling the context associated with multiple image regions, existing work oversimplifies this task to pairwise region modeling by multiple instance learning. In this paper, we propose a variational Bayesian method, called Variational Context, to solve the problem of complex context modeling in referring expression grounding. Our model exploits the reciprocal relation between the referent and context, i.e., either of them influences the estimation of the posterior distribution of the other, and thereby the search space of context can be greatly reduced, resulting in better localization of referent. We develop a novel cue-specific language-vision embedding network that learns this reciprocity model end-to-end. We also extend the model to the unsupervised setting where no annotation for the referent is available. Extensive experiments on various benchmarks show consistent improvement over state-of-the-art methods in both supervised and unsupervised settings.
更多查看译文
关键词
multimodal comprehension,exponential complexity,multiple image regions,multiple instance learning,variational Bayesian method,variational context,context modeling,referring expressions grounding,vision-language task,context - visual attributes,pairwise region modeling,posterior distribution estimation
AI 理解论文
溯源树
样例
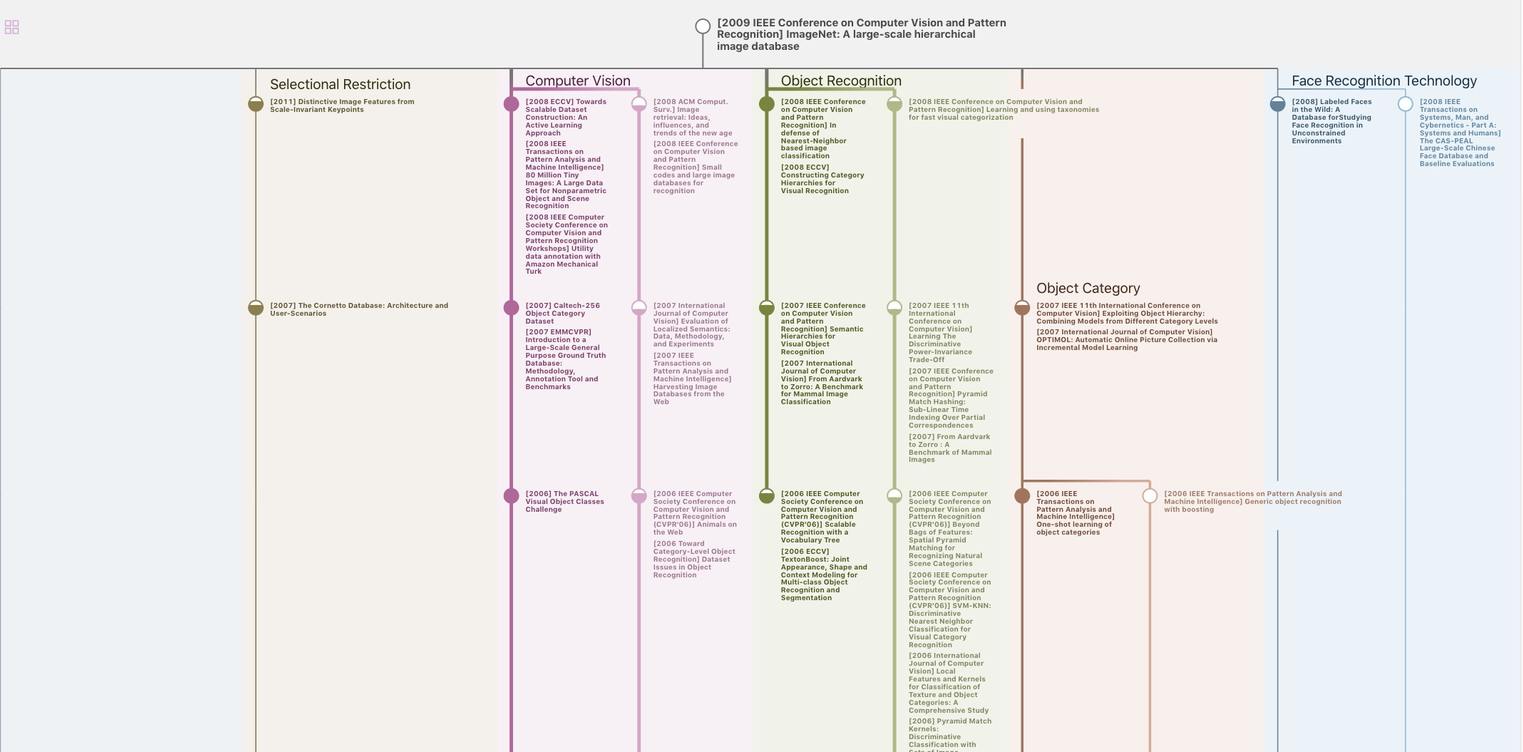
生成溯源树,研究论文发展脉络
Chat Paper
正在生成论文摘要