Optimal detection and error exponents for hidden multi-state processes via random duration model approach.
arXiv: Information Theory(2017)
摘要
We study detection of random signals corrupted by noise that over time switch their values (states) from a finite set of possible values, where the switchings occur at unknown points in time. We model such signals by means of a random duration model that to each possible state assigns a probability mass function which controls the statistics of durations of that state occurrences. Assuming two possible signal states and Gaussian noise, we derive optimal likelihood ratio test and show that it has a computationally tractable form of a matrix product, with the number of matrices involved in the product being the number of process observations. Each matrix involved in the product is of dimension equal to the sum of durations spreads of the two states, and it can be decomposed as a product of a diagonal random matrix controlled by the process observations and a sparse constant matrix which governs transitions in the sequence of states. Using this result, we show that the Neyman-Pearson error exponent is equal to the top Lyapunov exponent for the corresponding random matrices. Using theory of large deviations, we derive a lower bound on the error exponent. Finally, we show that this bound is tight by means of numerical simulations.
更多查看译文
AI 理解论文
溯源树
样例
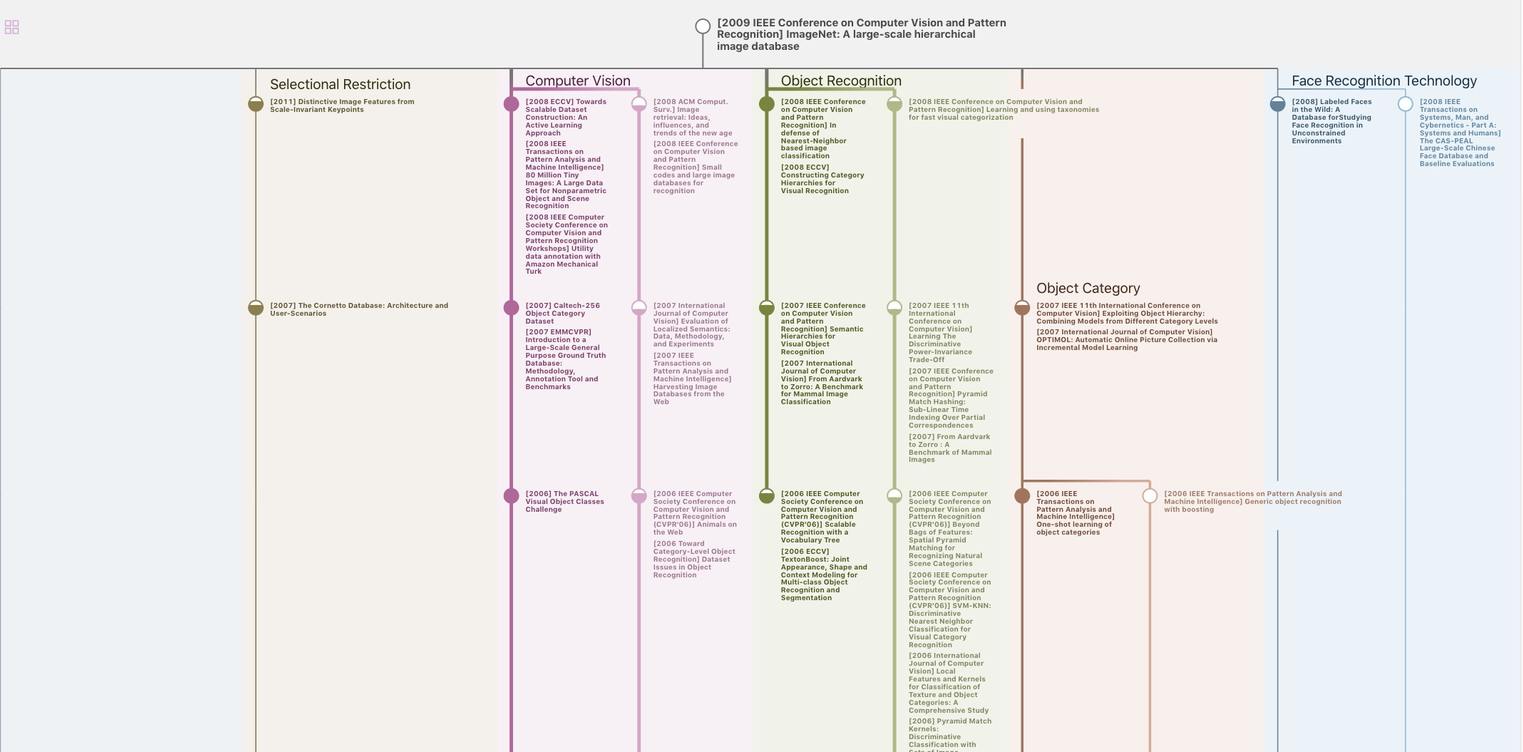
生成溯源树,研究论文发展脉络
Chat Paper
正在生成论文摘要