DMSS: A Robust Deep Meta Structure Based Similarity Measure in Heterogeneous Information Networks.
arXiv: Databases(2017)
摘要
Similarity measure as a fundamental task in heterogeneous information network analysis has been applied to many areas, e.g. product recommendation, clustering and web search. The state-of-the-art metrics depend on meta paths or meta structures specified by users. In this paper, a novel similarity measure on heterogeneous information networks, called Deep Meta Structure based Similarity ($DMSS$), is proposed. The deep meta structure as a schematic structure on heterogeneous information networks provides a unified framework integrating all the meta paths and meta structures. It can be constructed automatically. In order to formalize the semantics encapsulated in the deep meta structure, we decompose it into several deep meta paths, and then combine all the commuting matrices of these deep meta paths according to different weights. It is noteworthy that the weights can be determined by the proposed strategy. As a result, $DMSS$ is defined by virtue of the final commuting matrix and therefore is robust to different schematic structures. Experimental evaluations show that the state-of-the-art metrics are really sensitive to meta paths or meta structures in terms of clustering and ranking. Besides, $DMSS$ outperforms the state-of-the-art metrics in terms of ranking and clustering in the case of selecting an appropriate decaying parameter.
更多查看译文
AI 理解论文
溯源树
样例
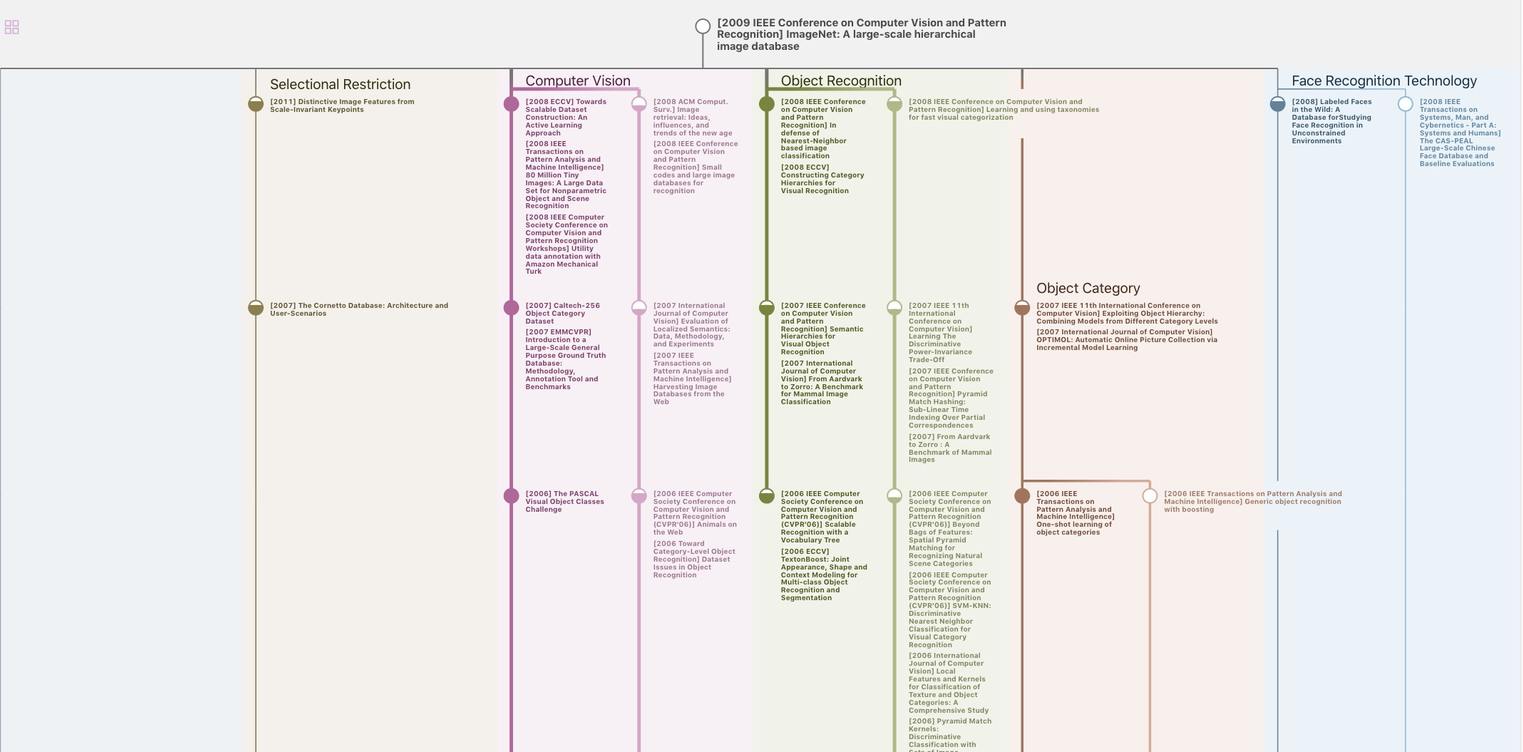
生成溯源树,研究论文发展脉络
Chat Paper
正在生成论文摘要