Gradient Regularization Improves Accuracy of Discriminative Models.
arXiv: Learning(2017)
摘要
Regularizing the gradient norm of the output of a neural network with respect to its inputs is a powerful technique, first proposed by Drucker u0026 LeCun (1991) who named it Double Backpropagation. The idea has been independently rediscovered several times since then, most often with the goal of making models robust against adversarial sampling. This paper presents evidence that gradient regularization can consistently and significantly improve classification accuracy on vision tasks, especially when the amount of training data is small. We introduce our regularizers as members of a broader class of Jacobian-based regularizers, and compare them theoretically and empirically. A straightforward objection against minimizing the gradient norm at the training points is that a locally optimal solution, where the model has small gradients at the training points, may possibly contain large changes at other regions. We demonstrate through experiments on real and synthetic tasks that stochastic gradient descent is unable to find these locally optimal but globally unproductive solutions. Instead, it is forced to find solutions that generalize well.
更多查看译文
AI 理解论文
溯源树
样例
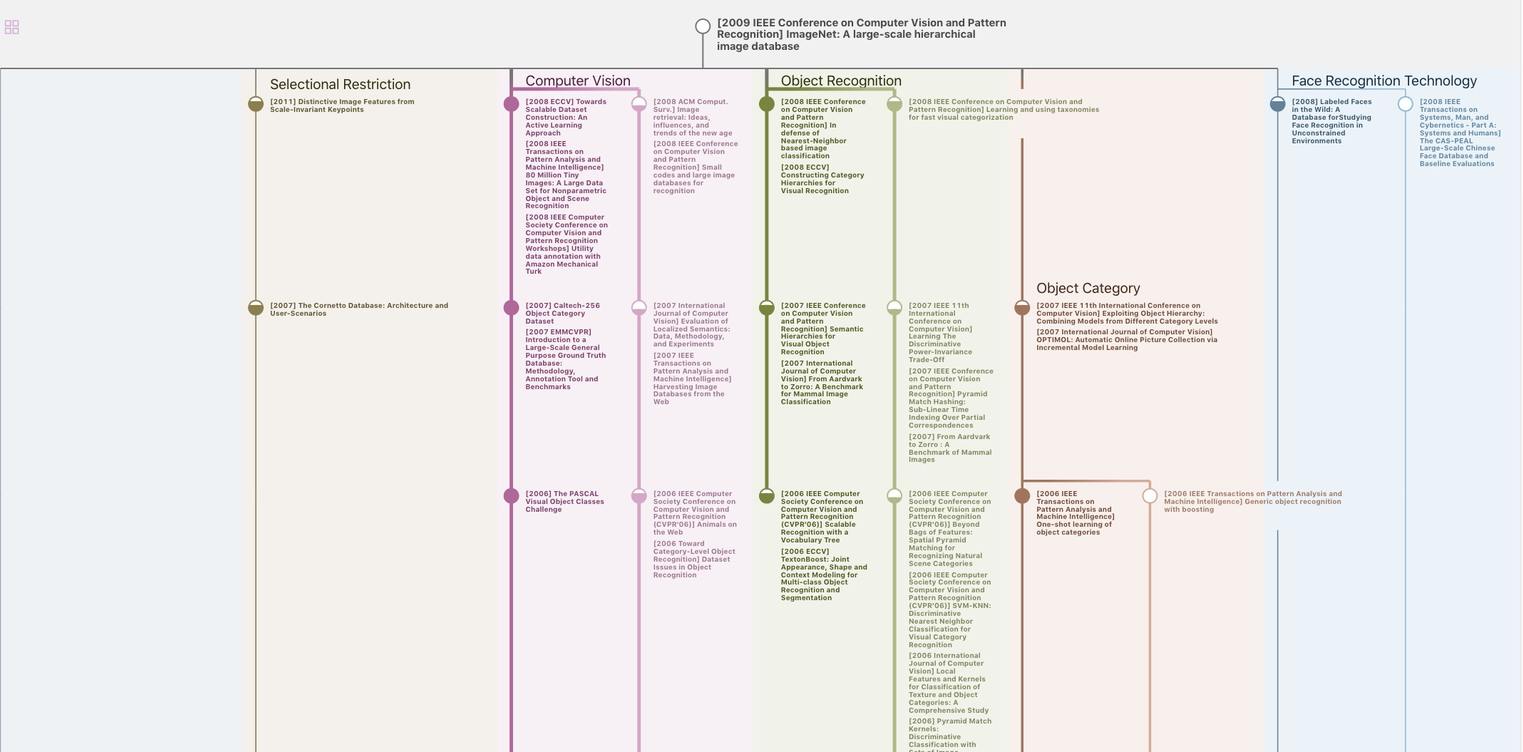
生成溯源树,研究论文发展脉络
Chat Paper
正在生成论文摘要