Content-Based Classification Of Sensitive Tweets
INTERNATIONAL JOURNAL OF SEMANTIC COMPUTING(2017)
摘要
Online Social Networks (OSNs), such as Facebook and Twitter, provide open platforms for users to easily share their statuses, opinions, and ideas, ranging from personal experiences/activities to breaking news. With the increasing popularity of online social networks and the explosion of blog and microblog messages, we have observed large amounts of potentially sensitive or private messages being published to OSNs inadvertently or voluntarily. The owners of these messages may become vulnerable to online stalkers or adversaries, especially considering that many online social network platforms (e.g. Twitter) provide open access to the public, including unregistered users and search engine bots. Studies show that users often regret posting sensitive or private messages. However, it is very difficult to completely erase such messages from the Internet, especially when the messages have been indexed by the search engines or forwarded (e.g. re-tweet in Twitter) by other users.Therefore, it is critical to identify messages that reveal private/sensitive information, and warn users before they post the messages to the public. However, the definition of sensitive information is subjective and different from user to user. For example, some users may feel comfortable sharing political opinions, while others do not. To develop a privacy protection mechanism that is customizable to fit the needs of diverse audiences, it is essential to accurately and automatically classify potentially sensitive messages into topic categories, such as health, politics, family, relationship, religion, etc. In this paper, we make the first attempt to classify sensitive tweets into 13 pre-defined topic categories. In particular, we model the semantic content of tweets with term distribution features as well as users' topic-preferences based on personal tweet history. We also add domain-specific features, i.e. domain knowledge, to improve classification performance. Experiments show that our method can boost classification accuracy compared with the well-known Bag-of-Words and TF-IDF methods.
更多查看译文
关键词
Online Social Networks, privacy, classification, Twitter
AI 理解论文
溯源树
样例
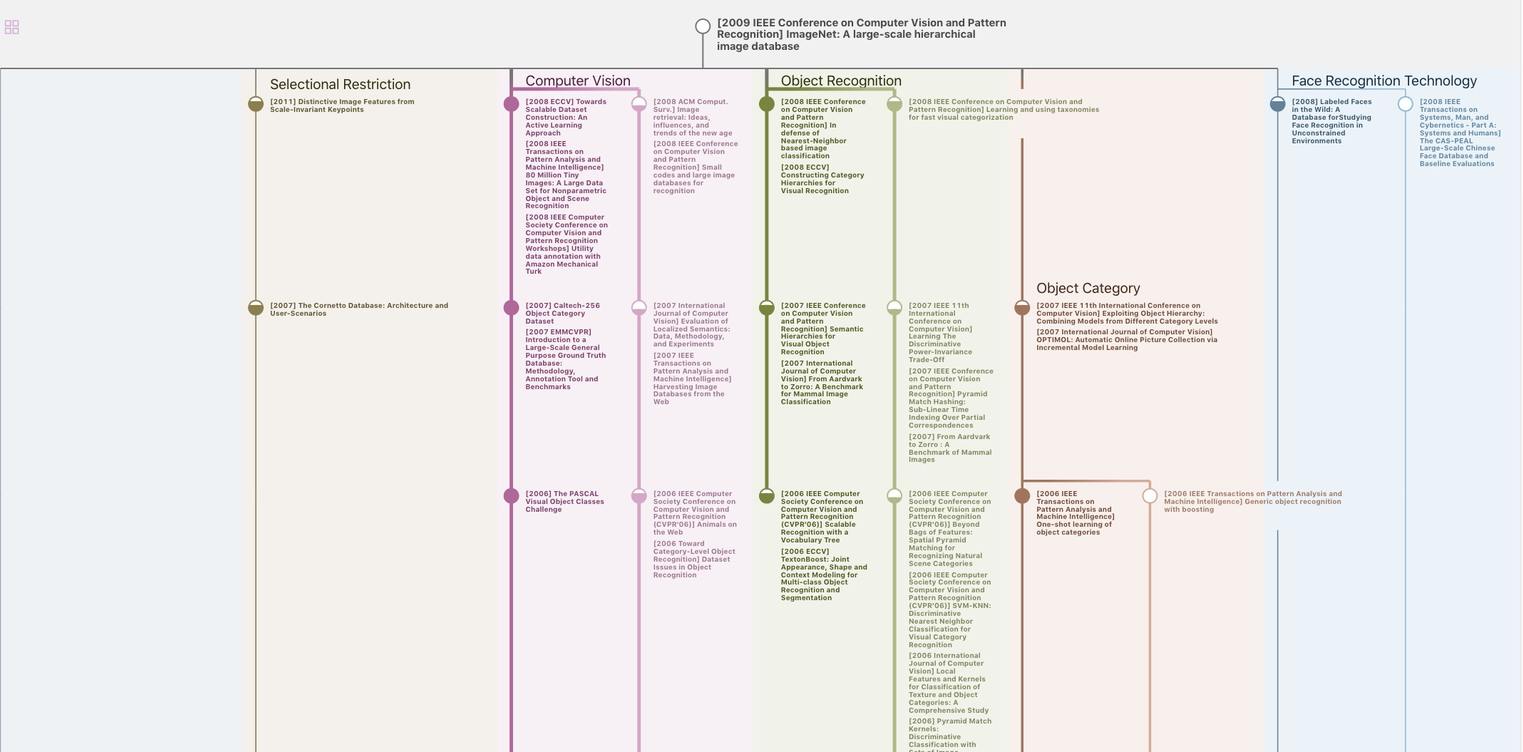
生成溯源树,研究论文发展脉络
Chat Paper
正在生成论文摘要