Deep joint discriminative learning for vehicle re-identification and retrieval
2017 IEEE International Conference on Image Processing (ICIP)(2017)
摘要
In this paper, we propose a novel vehicle re-identification method based on a Deep Joint Discriminative Learning (DJDL) model, which utilizes a deep convolutional network to effectively extract discriminative representations for vehicle images. To exploit properties and relationship among samples in different views, we design a unified framework to combine several different tasks efficiently, including identification, attribute recognition, verification and triplet tasks. The whole network is optimized jointly via a specific batch composition design. Extensive experiments are conducted on a large-scale VehicleID [1] dataset. Experimental results demonstrate the effectiveness of our method and show that it achieves the state-of-the-art performance on both vehicle re-identification and retrieval.
更多查看译文
关键词
Joint Discriminative Learning,Vehicle Re-Identification,Vehicle Retrieval
AI 理解论文
溯源树
样例
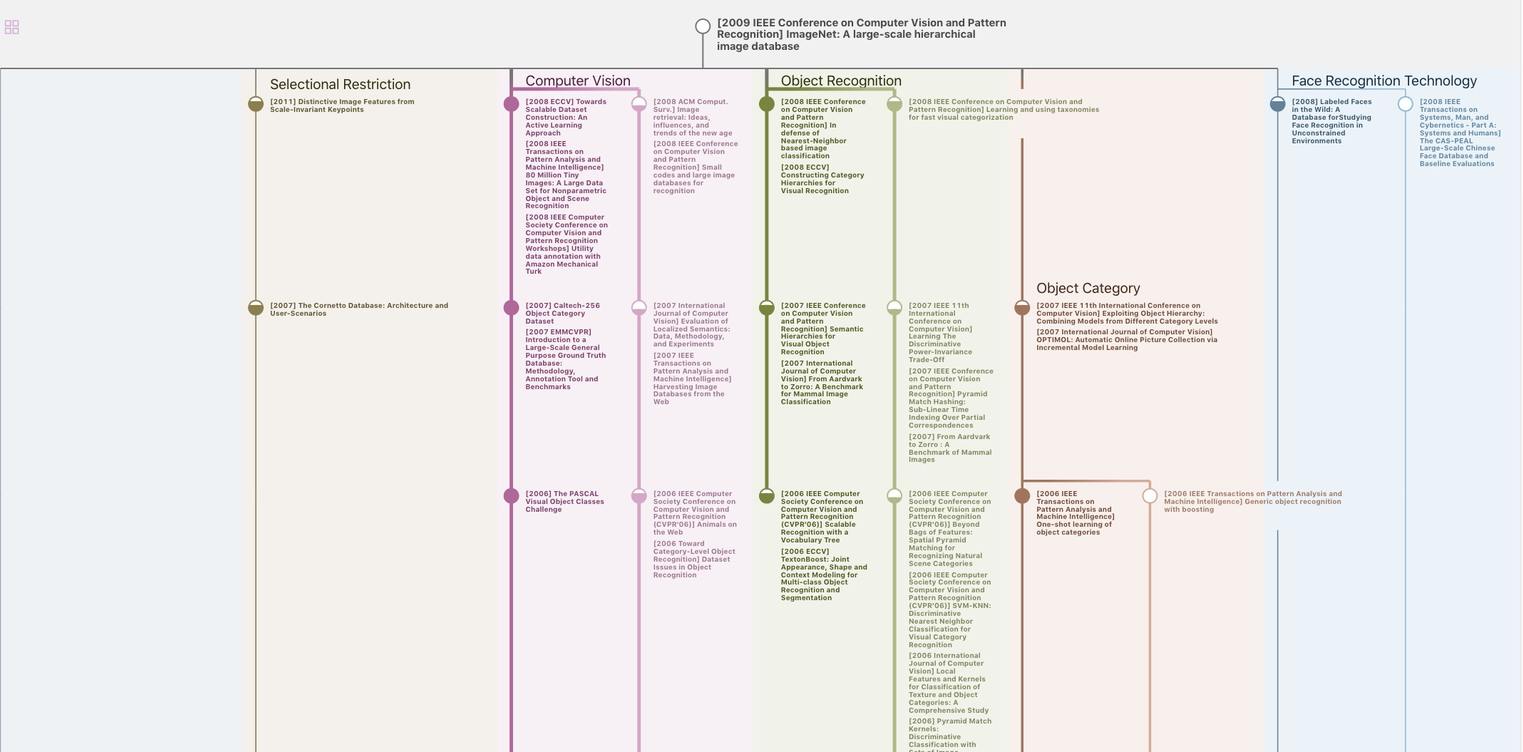
生成溯源树,研究论文发展脉络
Chat Paper
正在生成论文摘要