Unsupervised Feature Selection By Manifold Regularized Self-Representation
2017 24TH IEEE INTERNATIONAL CONFERENCE ON IMAGE PROCESSING (ICIP)(2017)
摘要
Unsupervised feature selection has been proven to be an efficient technique in mitigating the curse of dimensionality. It helps to understand and analyze the prevalent high dimensional unlabeled data. Recently, the self-similarity property of objects, which assumes that a feature can be represented by the linear combination of its relevant features, has been successfully used in unsupervised feature selection. However, it does not take the geometry structure of the sample space into consideration. In this paper, we propose a novel algorithm termed manifold regularized self-representation(MRSR). To preserve the local spatial structure, we incorporate an effective manifold regularization into the objective function. An iterative reweighted least square (IRLS) algorithm is developed to solve the optimization problem and the convergence is proved. Extensive experimental results on several benchmark datasets validate the effectiveness of the proposed method.
更多查看译文
关键词
Unsupervised feature selection, Self-representation, Manifold regularization, Group Sparsity
AI 理解论文
溯源树
样例
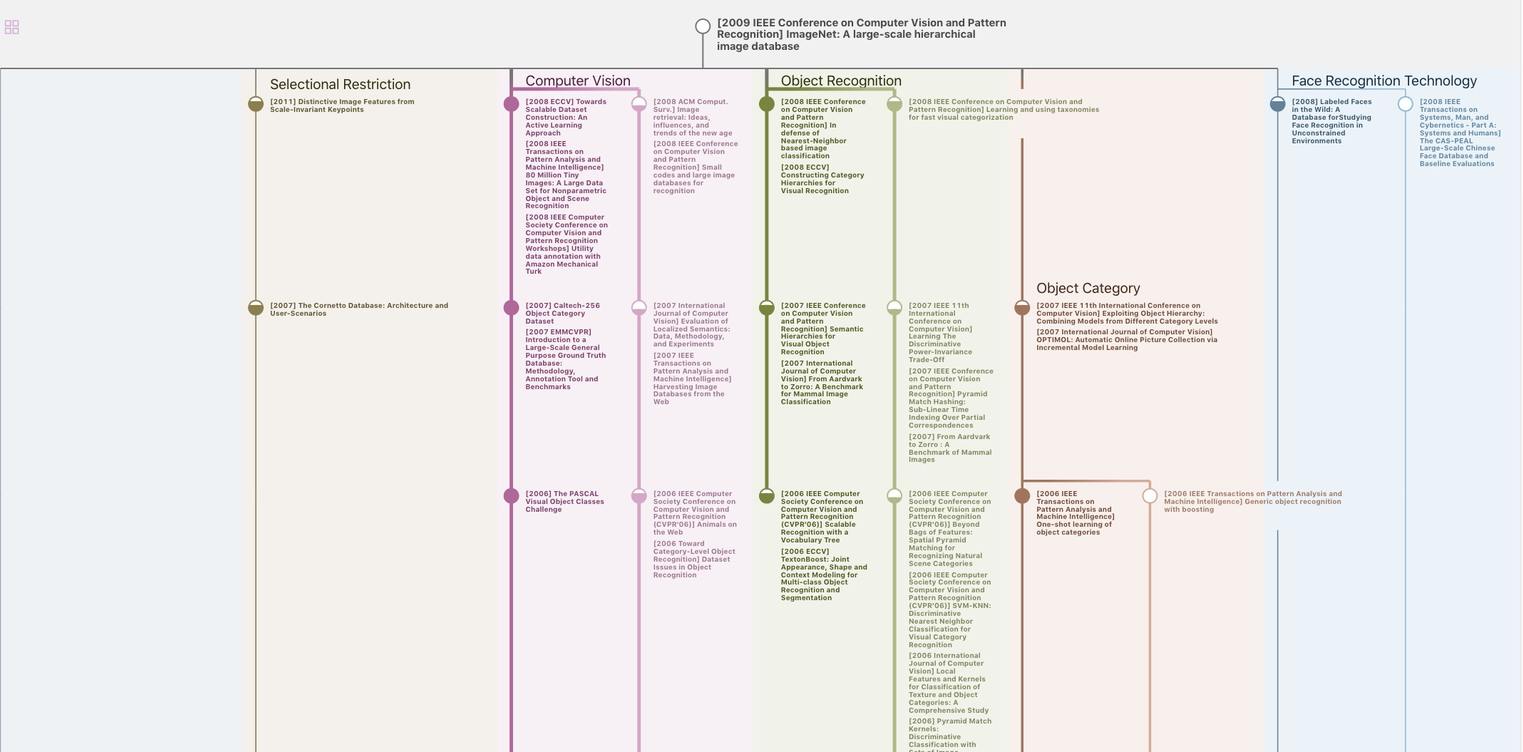
生成溯源树,研究论文发展脉络
Chat Paper
正在生成论文摘要