Joint denoising and decompression: A patch-based Bayesian approach
2017 24TH IEEE INTERNATIONAL CONFERENCE ON IMAGE PROCESSING (ICIP)(2017)
摘要
JPEG and Wavelet compression artifacts leading to Gibbs effects and loss of texture are well known and many restoration solutions exist in the literature. So is denoising, which has occupied the image processing community for decades. However, when a noisy image is compressed, the noisy wavelet coefficients can be assigned to the “wrong” quantization interval, generating artifacts that can have dramatic consequences in products derived from satellite image pairs such as sub-pixel stereo vision and digital terrain elevation models. Despite the fact that the importance of such artifacts in very high resolution satellite imaging has recently been recognized, this restoration problem has been rarely addressed in the literature. In this work we present a thorough probabilistic analysis of the wavelet outliers phenomenon, and conclude that their probabilistic nature is characterized by a single parameter related to the ratio q/σ of the compression rate and the instrumental noise. This analysis provides the conditional probability for a Bayesian MAP estimator, whereas a patch-based local Gaussian prior model is learnt from the corrupted image iteratively, like in state-of-the-art patch-based denoising algorithms, albeit with the additional difficulty of dealing with non-Gaussian noise during the learning process. The resulting joint denoising and decompression algorithm is experimentally evaluated under realistic conditions. The results show its ability to simultaneously denoise, decompress and remove wavelet outliers better than the available alternatives, both from a quantitative and a qualitative point of view. As expected, the advantage of our method is more evident for large values of q/σ.
更多查看译文
关键词
Inverse problems in Imaging, Satellite Imaging, Remote Sensing, Joint Denoising and Decompression, Patch-based non-local methods, Bayesian estimation
AI 理解论文
溯源树
样例
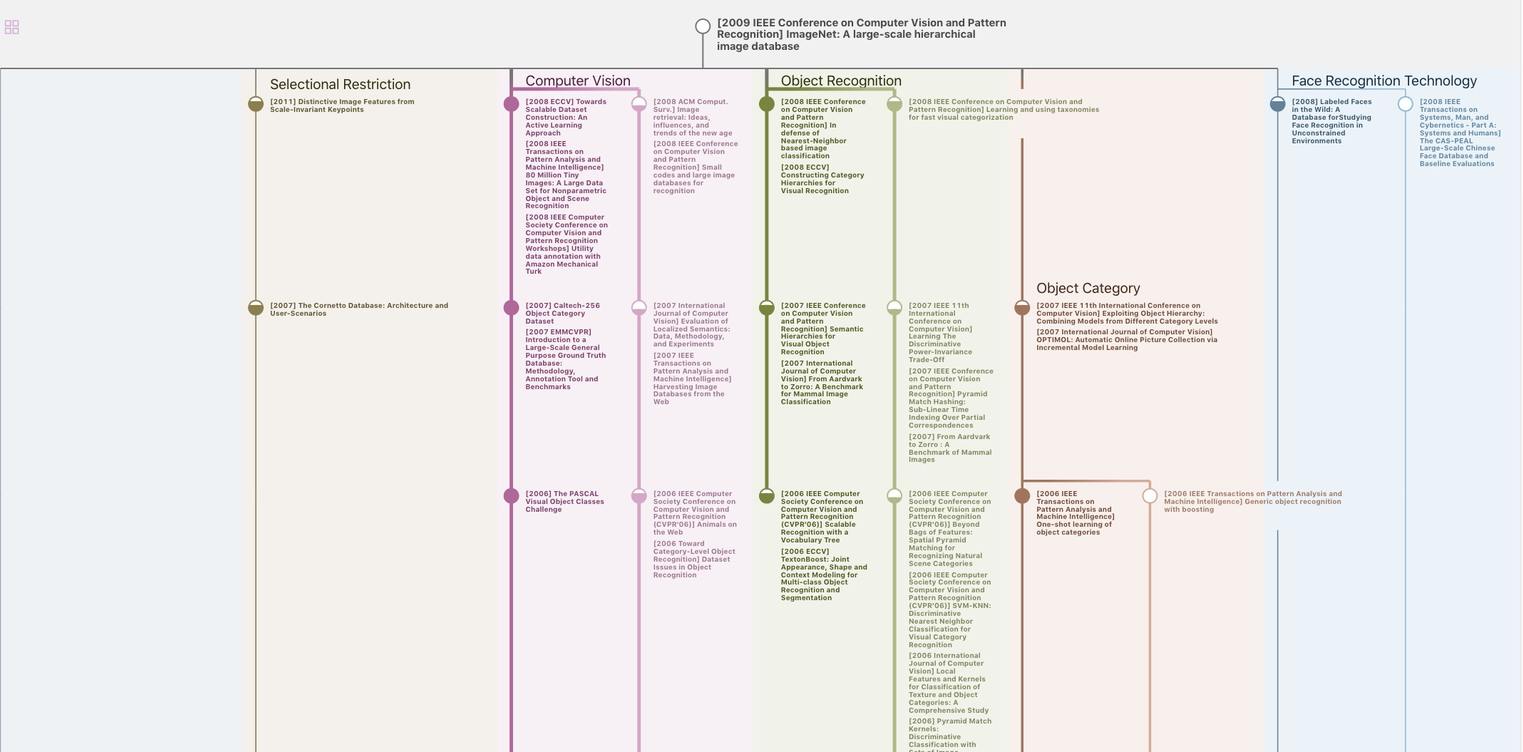
生成溯源树,研究论文发展脉络
Chat Paper
正在生成论文摘要